Few-Shot Domain Adaption-Based Specific Emitter Identification under Varying Modulation
2023 IEEE 23rd International Conference on Communication Technology (ICCT)(2023)
关键词
Specific emitter identification (SEI),transfer learning (TL),maximum mean discrepancy (MMD)
AI 理解论文
溯源树
样例
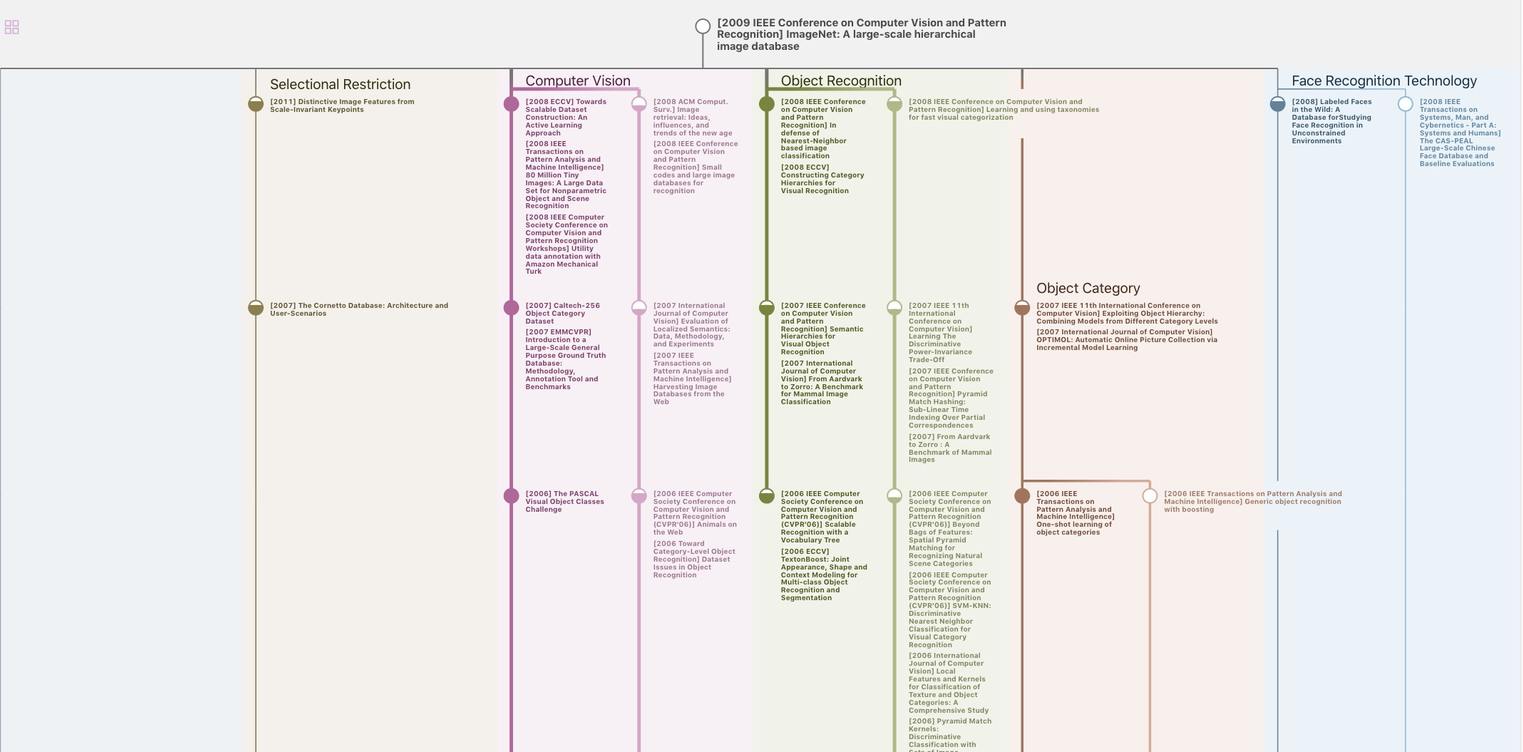
生成溯源树,研究论文发展脉络
Chat Paper
正在生成论文摘要