German CheXpert Chest X-ray Radiology Report Labeler
ROFO-FORTSCHRITTE AUF DEM GEBIET DER RONTGENSTRAHLEN UND DER BILDGEBENDEN VERFAHREN(2024)
摘要
Purpose The aim of this study was to develop an algorithm to automatically extract annotations from German thoracic radiology reports to train deep learning-based chest X-ray classification models. Materials and Methods An automatic label extraction model for German thoracic radiology reports was designed based on the CheXpert architecture. The algorithm can extract labels for twelve common chest pathologies, the presence of support devices, and "no finding". For iterative improvements and to generate a ground truth, a web-based multi-reader annotation interface was created. With the proposed annotation interface, a radiologist annotated 1086 retrospectively collected radiology reports from 2020-2021 (data set 1). The effect of automatically extracted labels on chest radiograph classification performance was evaluated on an additional, in-house pneumothorax data set (data set 2), containing 6434 chest radiographs with corresponding reports, by comparing a DenseNet-121 model trained on extracted labels from the associated reports, image-based pneumothorax labels, and publicly available data, respectively. Results Comparing automated to manual labeling on data set 1: "mention extraction" class-wise F1 scores ranged from 0.8 to 0.995, the "negation detection" F1 scores from 0.624 to 0.981, and F1 scores for "uncertainty detection" from 0.353 to 0.725. Extracted pneumothorax labels on data set 2 had a sensitivity of 0.997 [95 % CI: 0.994, 0.999] and specificity of 0.991 [95 % CI: 0.988, 0.994]. The model trained on publicly available data achieved an area under the receiver operating curve (AUC) for pneumothorax classification of 0.728 [95 % CI: 0.694, 0.760], while the models trained on automatically extracted labels and on manual annotations achieved values of 0.858 [95 % CI: 0.832, 0.882] and 0.934 [95 % CI: 0.918, 0.949], respectively. Conclusion Automatic label extraction from German thoracic radiology reports is a promising substitute for manual labeling. By reducing the time required for data annotation, larger training data sets can be created, resulting in improved overall modeling performance. Our results demonstrated that a pneumothorax classifier trained on automatically extracted labels strongly outperformed the model trained on publicly available data, without the need for additional annotation time and performed competitively compared to manually labeled data.
更多查看译文
关键词
thorax,radiography,pneumothorax,neural networks,label extraction,annotation
AI 理解论文
溯源树
样例
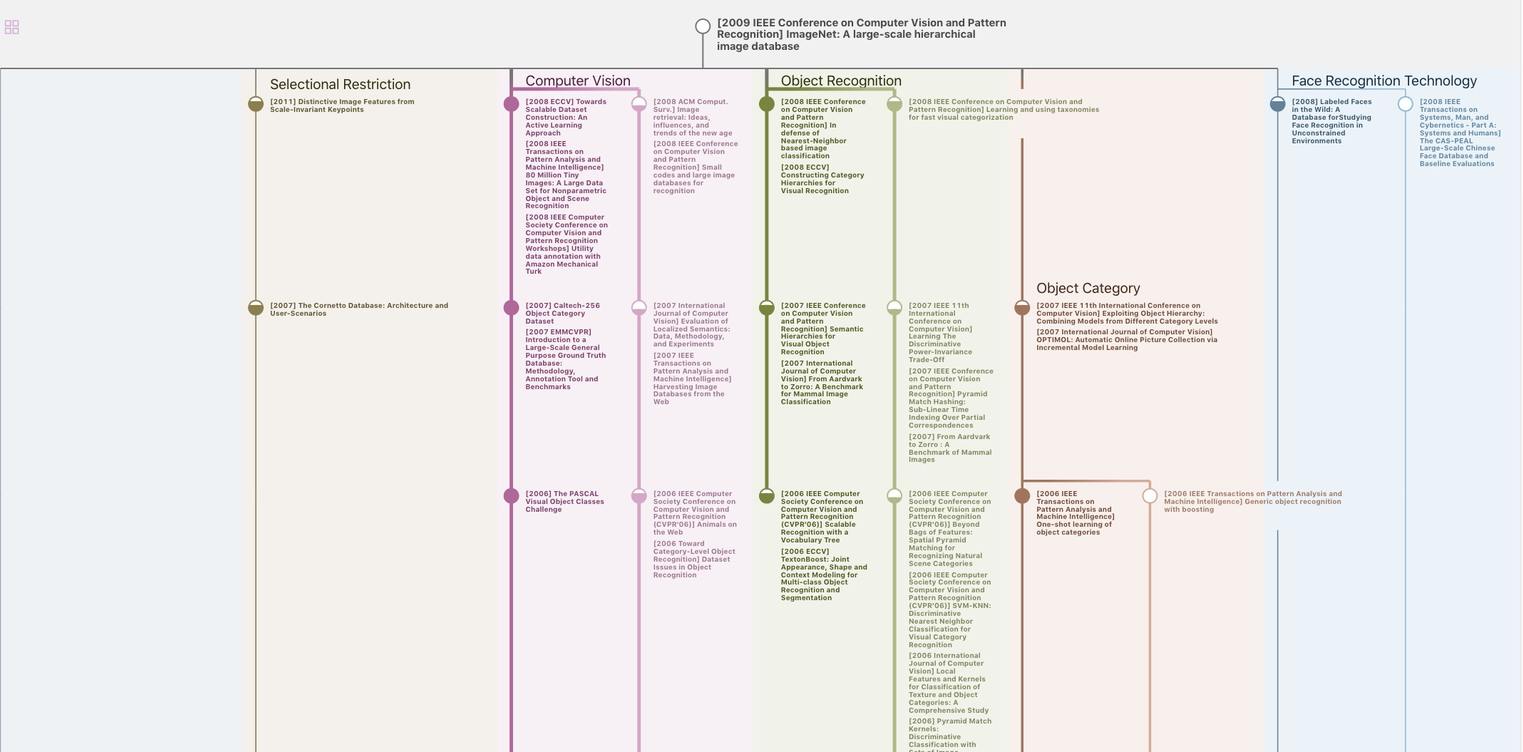
生成溯源树,研究论文发展脉络
Chat Paper
正在生成论文摘要