TFMA: Few-Shot RF Fingerprint Identification with Time-Frequency Mask Augmentation.
International Conference on Communication Technology(2023)
Abstract
Radio Frequency Fingerprinting Identification (RFFI), a promising wireless physical layer security technique, relies on inherent impairments within the hardware circuitry of wireless devices and parasitic elements in the signal waveform. Recently, deep learning methods have been widely used in RFFI, yielding advanced performance. However, these methods are proposed for big data scenarios and perform poorly in data-limited environments. Moreover, collecting labeled data for training is both expensive and time-consuming. For this reason, we propose a novel framework called Time-Frequency Mask Augmentation (TFMA) for Few-Shot RFFI (FS-RFFI). The framework comprises key components: Data Augmentation based on Occlusion Analysis (DAOA) and Data Augmentation based on Frequency Transform (DAFT). Simulation results show that TFMA methods can improve the performance of few-shot learning (FSL) methods to some extent, with a maximum improvement of 18% in cross-domain scenarios. Furthermore, our proposed method achieves the best performance compared to existing data enhancement methods.
MoreTranslated text
Key words
Few-shot learning,data augmentation,occlusion analysis,wireless physical layer security
AI Read Science
Must-Reading Tree
Example
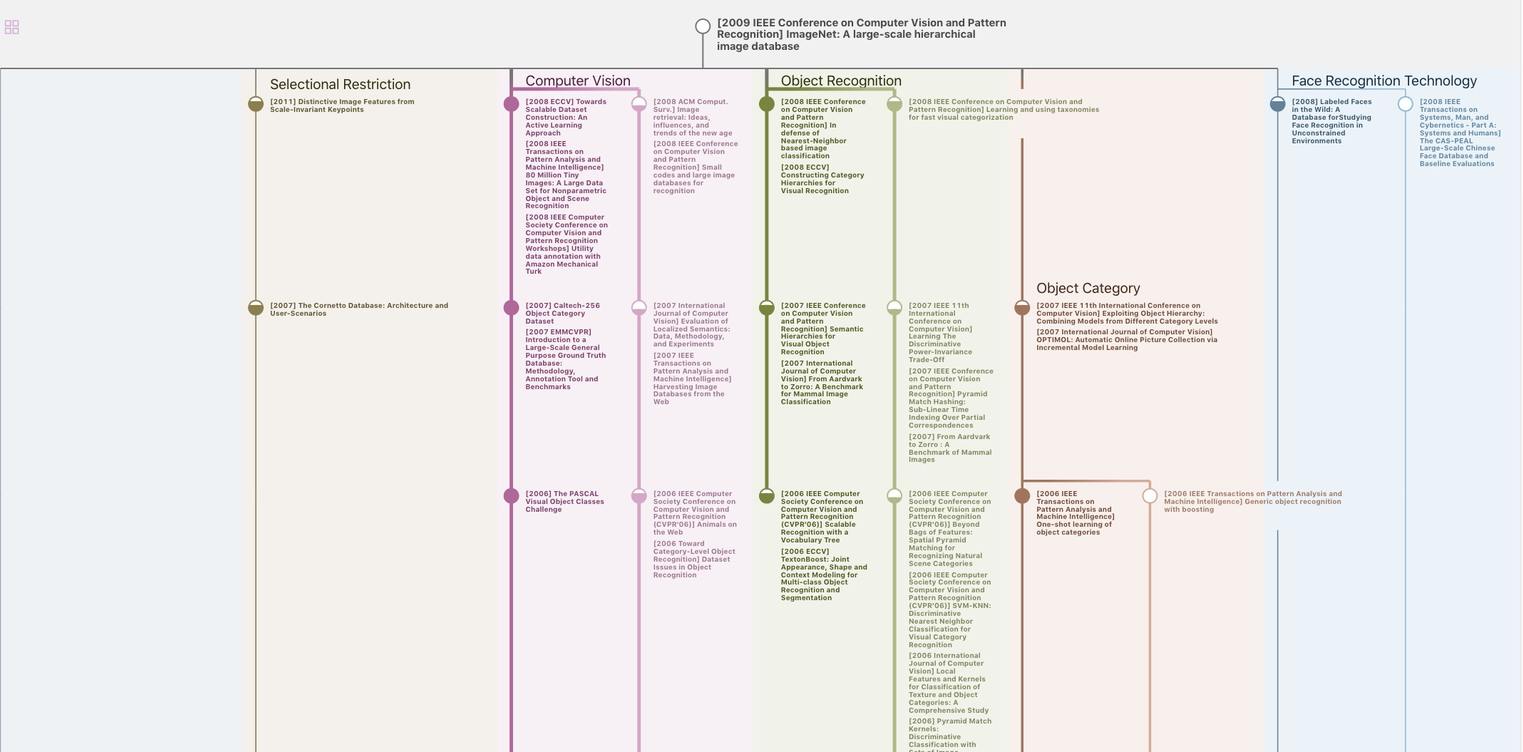
Generate MRT to find the research sequence of this paper
Chat Paper
Summary is being generated by the instructions you defined