Delay-Sensitive SFC Scheduling Optimization With DRL in Satellite-Terrestrial Networks.
International Conference on Communication Technology(2023)
摘要
Large-scale low Earth orbit (LEO) satellite communication systems play a pivotal role in shaping the future of 6G communication networks. This study delves into the intricacies of deploying delay-sensitive virtual network functions (VNFs) and scheduling service function chain (SFC) requests in satellite-terrestrial networks. We frame the SFC request scheduling (SFCRS) challenge as an integer linear programming (ILP) problem. We introduce an effective Deep Reinforcement Learning (DRL) approach to effectively tackle the SFCRS problem. The DRL-SFCRS algorithm primarily employs a sequence-to-sequence model and leverages Asynchronous Advantage Actor-Critic (A3C) for updating network parameters to attain the optimal policy. Simulation results underscore the algorithm's ability to adeptly balance the trade-off between end-to-end delay and resource utilization in the context of the SFCRS problem. As a result, the algorithm yields enhanced operator profits.
更多查看译文
关键词
Low-orbit satellite,network function virtualization,service function chain,DRL,end-to-end delay
AI 理解论文
溯源树
样例
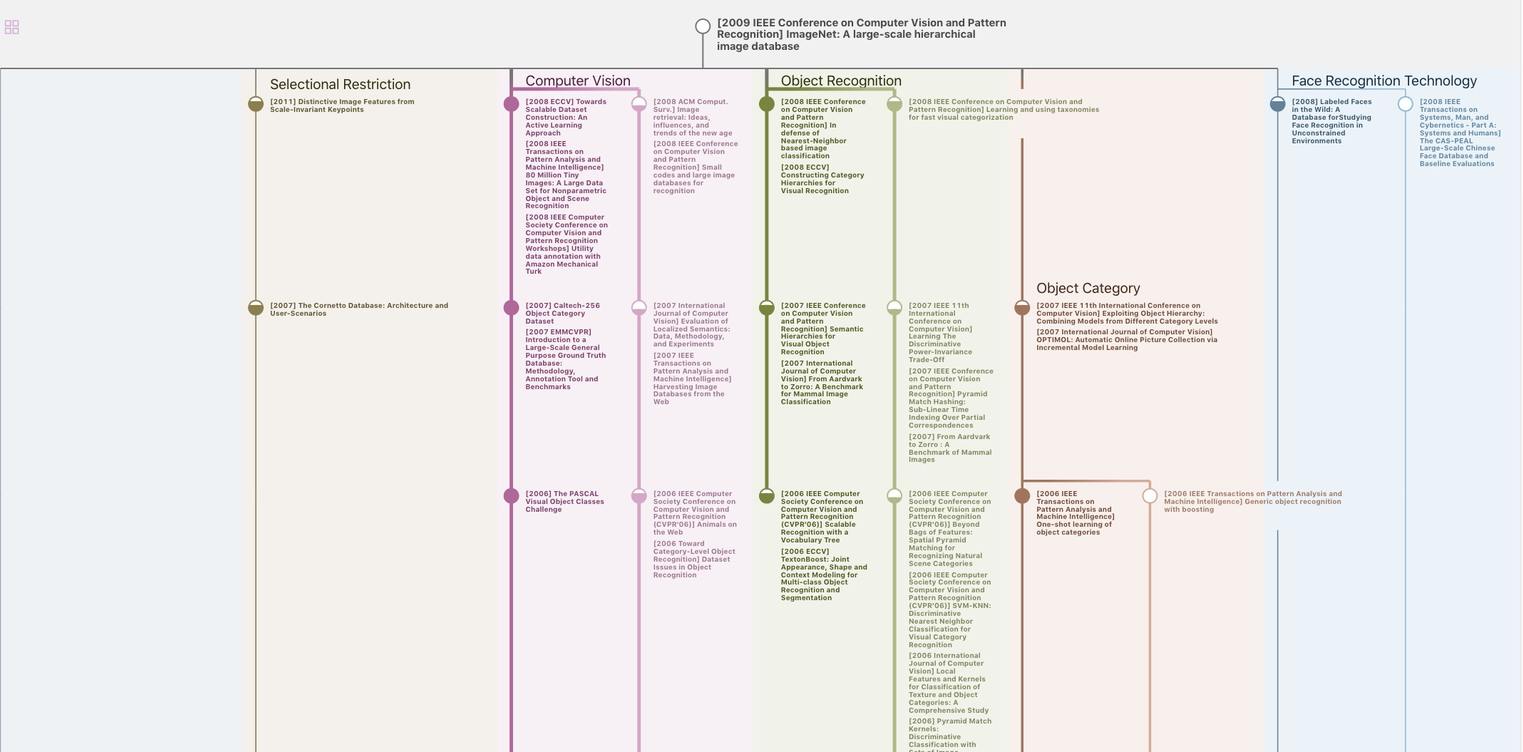
生成溯源树,研究论文发展脉络
Chat Paper
正在生成论文摘要