Beam Training and Power Allocation in Near-Field NOMA Systems Based on DRL.
International Conference on Communication Technology(2023)
摘要
Extremely large-scale array (XL-array) is able to significantly improve the spectral efficiency of wireless communication systems, so it is regarded as one of the most important technologies for the next-generation networks. However, larger antenna aperture makes the Rayleigh distance significantly increased, so future communication may take place more in the near-field region, hence many communication technologies designed for traditional far-field scenarios are no longer applicable. Also, as an emerging access technology, non-orthogonal multiple access (NOMA) can meet the needs of large-scale access and large system rates, Therefore, it is necessary to study NOMA system in near-field region. In this paper, we consider the joint beam training and power allocation problem in a near-field downlink NOMA system. Depending on the multi-cluster nature of the NOMA system, we need to consider user grouping, beam training, and power allocation to achieve maximum system rate. We consider both communication scenarios with complete CSI and communication scenarios with only users' location, and we propose two deep reinforcement learning (DRL) based beam training and power allocation schemes to solve this problem. Simulation results show that our proposed scheme can effectively improve system rate and reduce system computation time.
更多查看译文
关键词
Near-field,non-orthogonal multiple access (NOMA),beam training,power allocation,deep reinforcement learning (DRL)
AI 理解论文
溯源树
样例
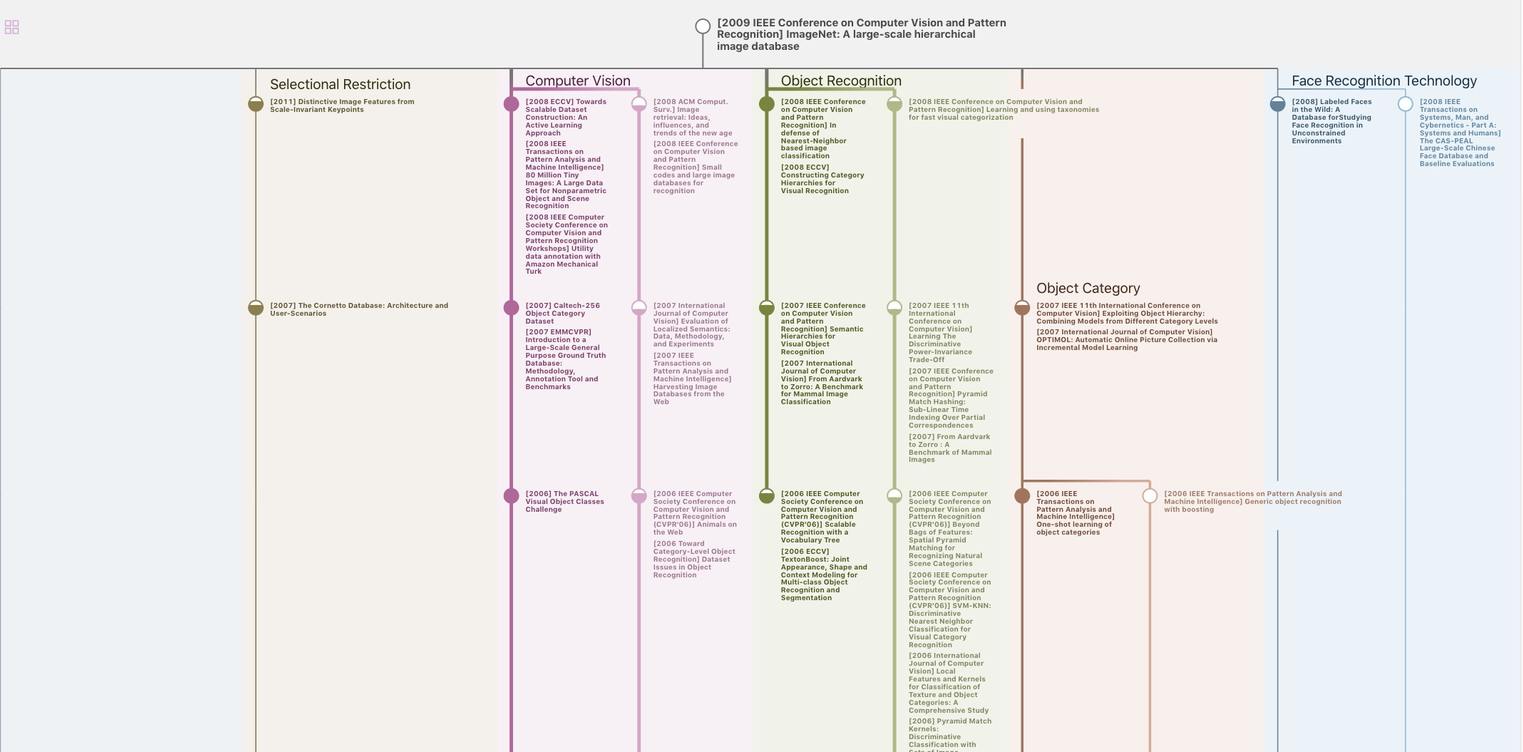
生成溯源树,研究论文发展脉络
Chat Paper
正在生成论文摘要