Attentive graph structure learning embedded in deep spatial-temporal graph neural network for traffic forecasting
Applied Intelligence(2024)
Abstract
A smooth traffic flow is very crucial for an intelligent traffic system. Consequently, traffic forecasting is critical in achieving unwrinkled traffic flow. However, due to its spatial and temporal interdependence, traffic forecasting might be more difficult. Graph neural networks (GNN) are widely used to obtain traffic forecasting due to their capability to operate in non-Euclidean data. The topological connection of the traffic network plays a crucial role in graph structure learning. Therefore, the importance of the adjacency matrix in graph construction might be significant for effective traffic forecasting. As a result, for efficient graph construction, a Deep Spatial-Temporal Graph Neural Network (DSTGNN) is proposed for the construction of the weighted adjacency matrix and traffic speed prediction accurately by recording topological and temporal information. Three different weighted adjacency matrices are suggested depending on three different proposed algorithms for different variations of traffic’s spatial condition. The new adjacency matrix is used in a novel DSTGNN for predicting the traffic state. The novel model comprised multiple layers with skip connections to adequately extract the variation of temporal and spatial information. DSTGNN outperforms the standard and other graph neural network models on four traffic speed datasets, SZ-taxi, Los-loop, PeMSD7, and METR-LA. Graphical abstract
MoreTranslated text
Key words
Traffic forecasting,Graph structure learning,Deep spatial-temporal graph neural network,Spatial-temporal,Gated recurrent unit
AI Read Science
Must-Reading Tree
Example
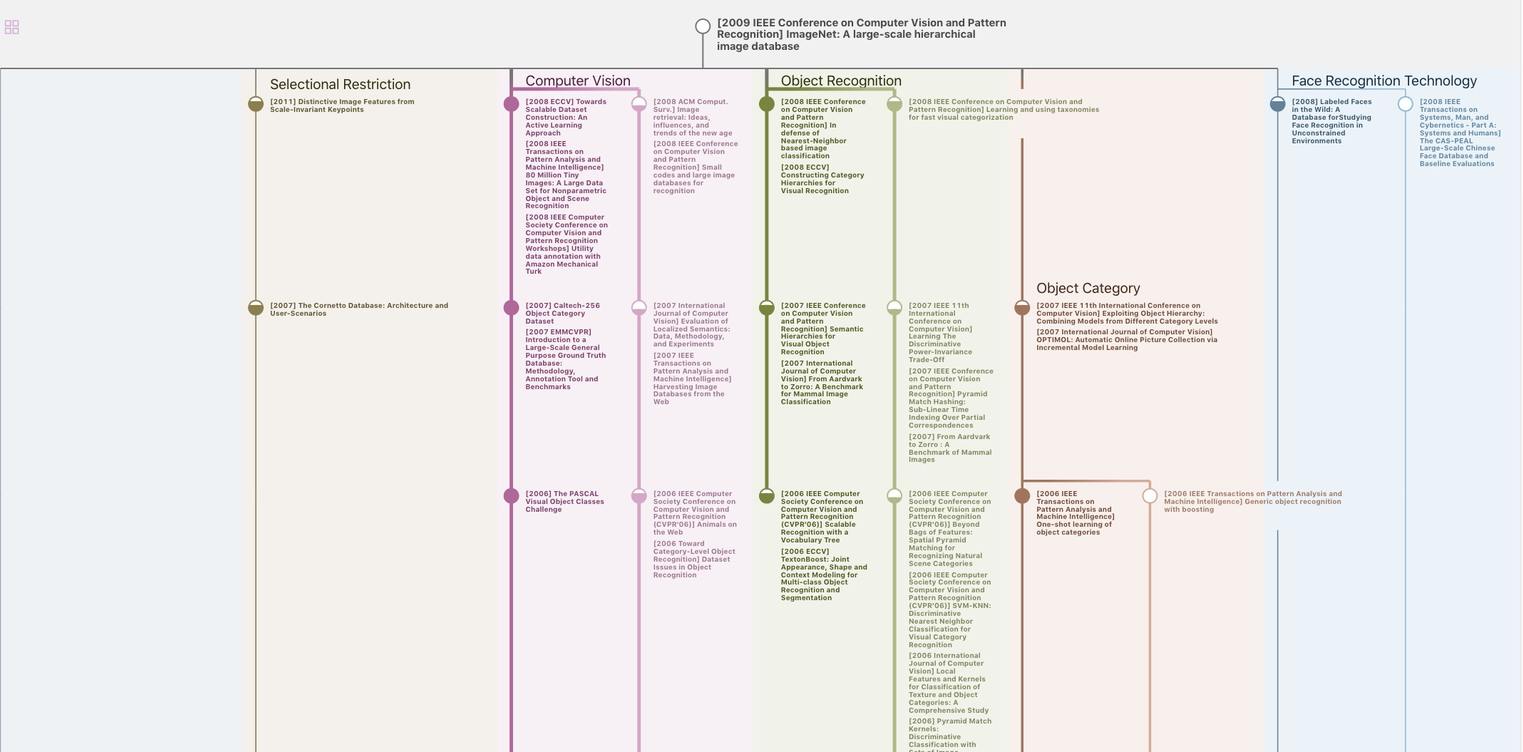
Generate MRT to find the research sequence of this paper
Chat Paper
Summary is being generated by the instructions you defined