RIFD-Net: A Robust Image Forgery Detection Network
IEEE ACCESS(2024)
摘要
Image splicing forensic technologies reveal manipulations that add or remove objects from images. However, the performance of existing splicing forensic methods is fatally degraded when detecting noisy images, as they often ignore the influence of image noise. In this paper, we propose a new forgery detection network called the robust image forgery detection network (RIFD-Net) based on convolutional neural networks (CNNs). With the help of multi-classifiers and a denoising network, RIFD-Net can effectively filter out multiple types of image noise before forgery detection. To determine the extent of tampering, we follow the Siamese network to calculate the similarity between two image patches, without prior knowledge of forensic traces. Results from extensive experiments on benchmark datasets indicate that our method outperforms existing image splicing forensic methods, achieving a substantial improvement of over 20% in the mean average precision (mAP) for forgery detection. Furthermore, RIFD-Net accurately locates splice areas, even in the presence of noise.
更多查看译文
关键词
Splicing forensics,forgery detection,image denoising,convolutional neural networks
AI 理解论文
溯源树
样例
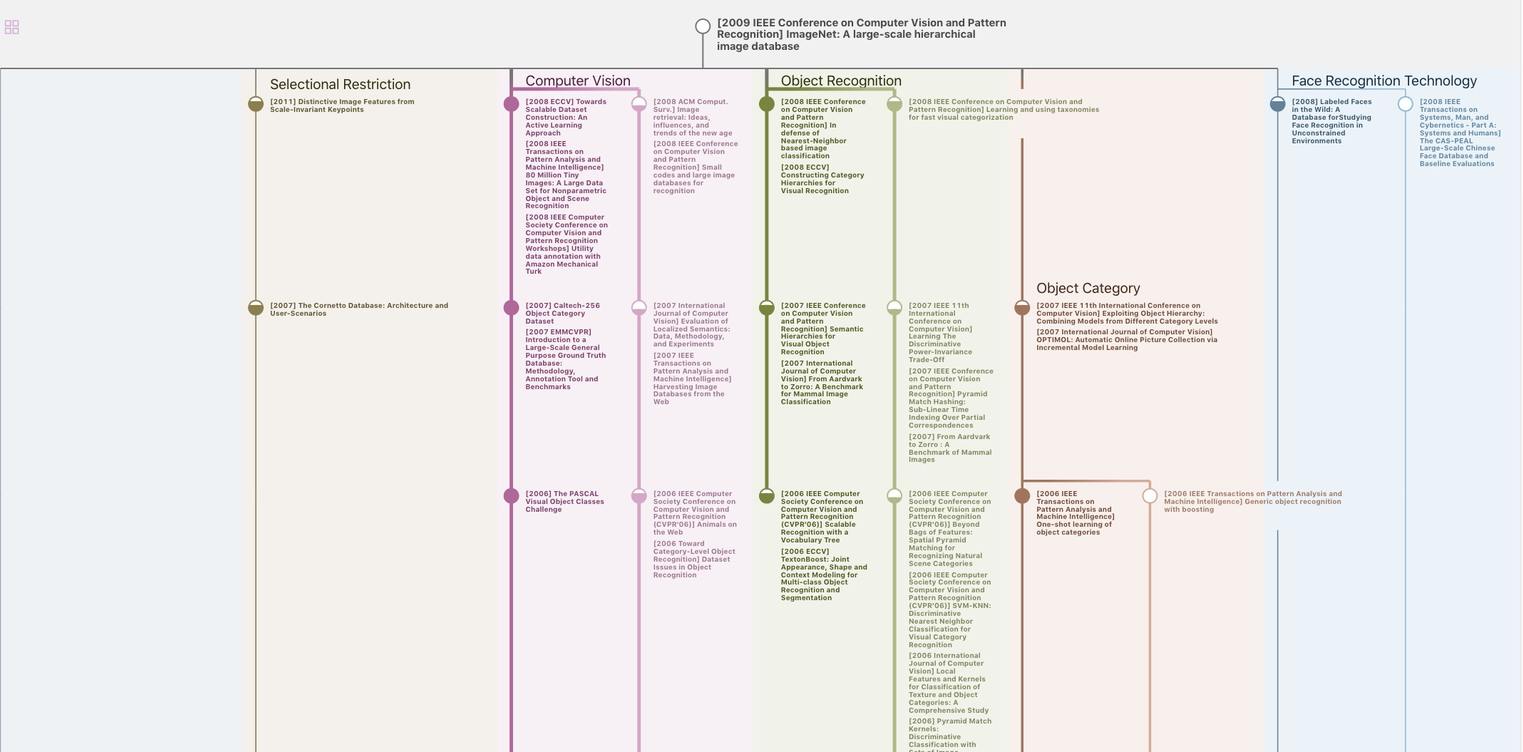
生成溯源树,研究论文发展脉络
Chat Paper
正在生成论文摘要