Using Graph Theory for Improving Machine Learning-based Detection of Cyber Attacks
CoRR(2024)
摘要
Early detection of network intrusions and cyber threats is one of the main
pillars of cybersecurity. One of the most effective approaches for this purpose
is to analyze network traffic with the help of artificial intelligence
algorithms, with the aim of detecting the possible presence of an attacker by
distinguishing it from a legitimate user. This is commonly done by collecting
the traffic exchanged between terminals in a network and analyzing it on a
per-packet or per-connection basis. In this paper, we propose instead to
perform pre-processing of network traffic under analysis with the aim of
extracting some new metrics on which we can perform more efficient detection
and overcome some limitations of classical approaches. These new metrics are
based on graph theory, and consider the network as a whole, rather than
focusing on individual packets or connections. Our approach is validated
through experiments performed on publicly available data sets, from which it
results that it can not only overcome some of the limitations of classical
approaches, but also achieve a better detection capability of cyber threats.
更多查看译文
AI 理解论文
溯源树
样例
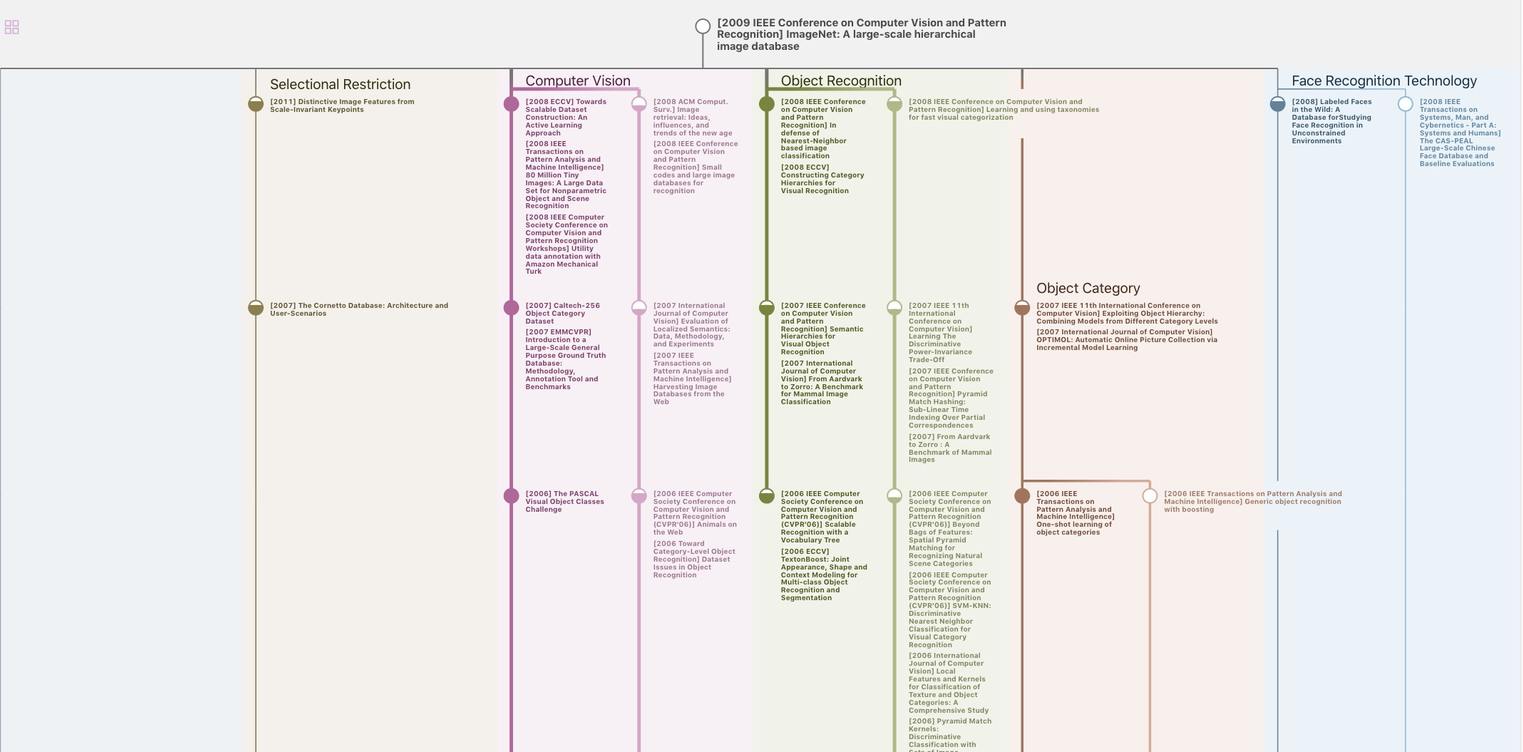
生成溯源树,研究论文发展脉络
Chat Paper
正在生成论文摘要