IR-Aware ECO Timing Optimization Using Reinforcement Learning
CoRR(2024)
摘要
Engineering change orders (ECOs) in late stages make minimal design fixes to
recover from timing shifts due to excessive IR drops. This paper integrates
IR-drop-aware timing analysis and ECO timing optimization using reinforcement
learning (RL). The method operates after physical design and power grid
synthesis, and rectifies IR-drop-induced timing degradation through gate
sizing. It incorporates the Lagrangian relaxation (LR) technique into a novel
RL framework, which trains a relational graph convolutional network (R-GCN)
agent to sequentially size gates to fix timing violations. The R-GCN agent
outperforms a classical LR-only algorithm: in an open 45nm technology, it (a)
moves the Pareto front of the delay-area tradeoff curve to the left and (b)
saves runtime over the classical method by running fast inference using trained
models at iso-quality. The RL model is transferable across timing
specifications, and transferable to unseen designs with zero-shot learning or
fine tuning.
更多查看译文
AI 理解论文
溯源树
样例
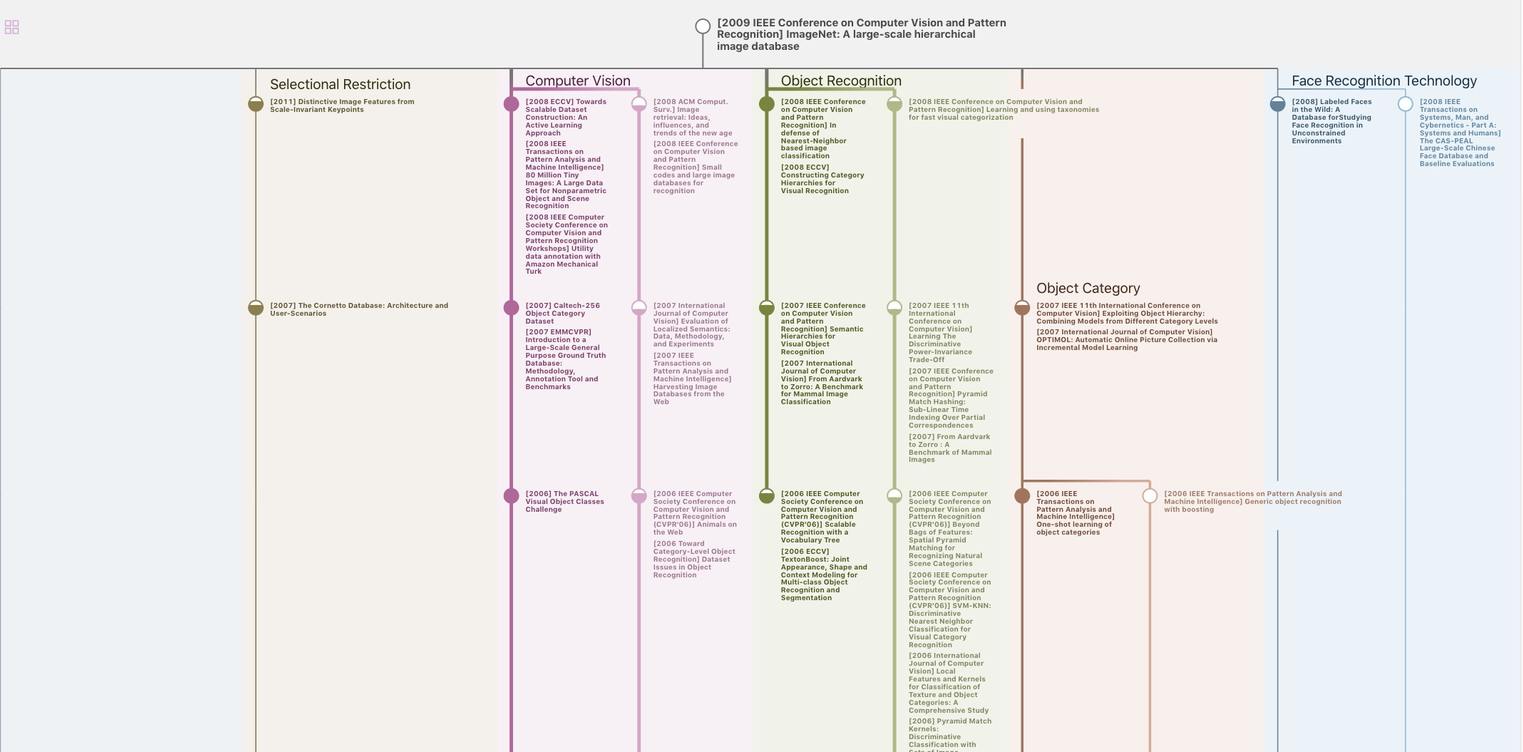
生成溯源树,研究论文发展脉络
Chat Paper
正在生成论文摘要