Autonomous Data Selection with Language Models for Mathematical Texts
arxiv(2024)
摘要
To improve language models' proficiency in mathematical reasoning via
continual pretraining, we introduce a novel strategy that leverages base
language models for autonomous data selection. Departing from conventional
supervised fine-tuning or trained classifiers with human-annotated data, our
approach Autonomous Data Selection (AutoDS) utilizes meta-prompted language
models as zero-shot verifiers to evaluate and select high-quality mathematical
content autonomously. To demonstrate the efficacy of our method, we
continuously pretrained a 7B-parameter language model on our curated dataset,
achieving substantial improvements in downstream performance on the MATH,
GSM8K, and BIG-Bench Hard (BBH) tasks with a token amount reduced by orders of
magnitude compared to previous continual pretraining works. Our method
showcases a 2 times increase in pretraining token efficiency compared to
state-of-the-art baselines, underscoring the potential of our approach in
enhancing models' mathematical reasoning capabilities. The AutoMathText dataset
is available at https://huggingface.co/datasets/math-ai/AutoMathText. The code
is available at https://github.com/yifanzhang-pro/AutoMathText.
更多查看译文
AI 理解论文
溯源树
样例
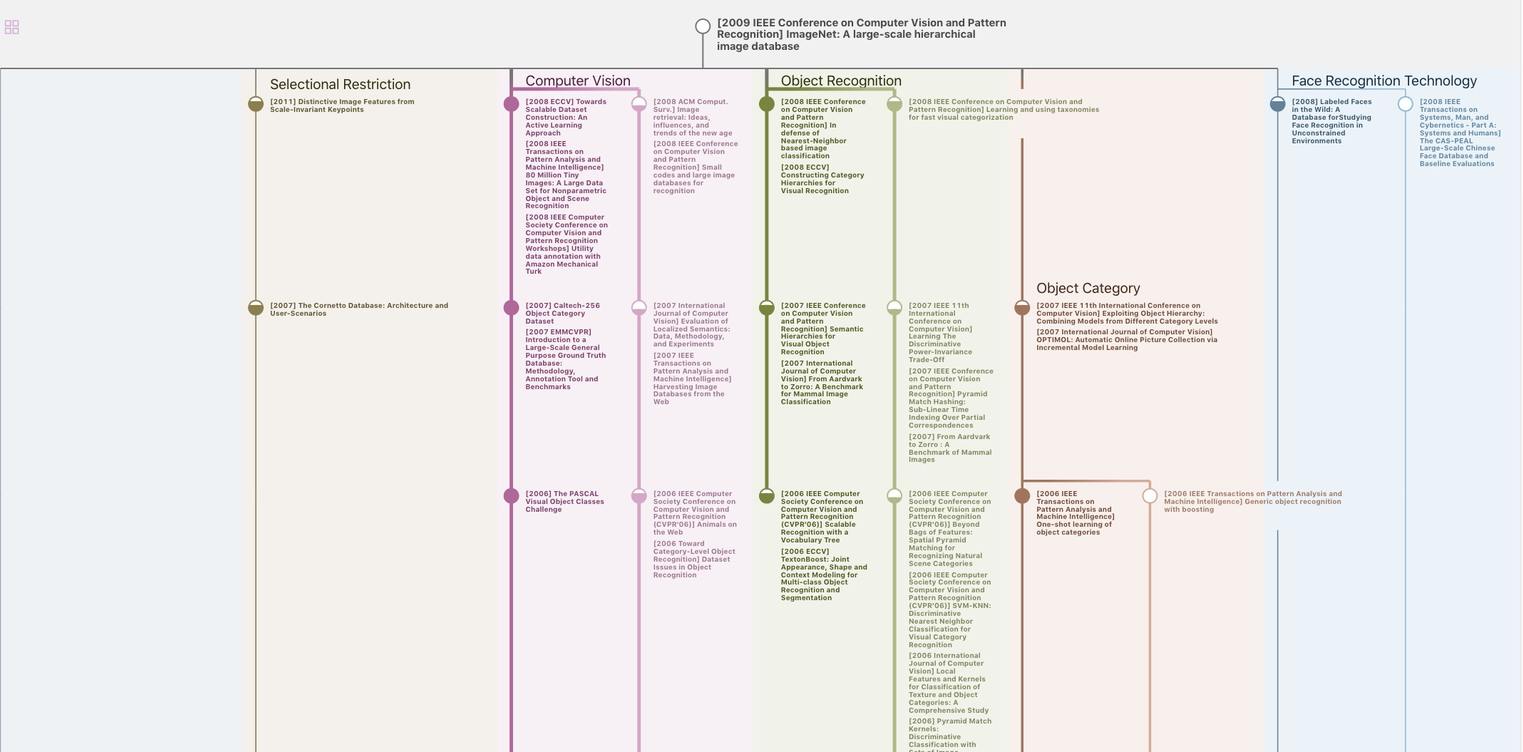
生成溯源树,研究论文发展脉络
Chat Paper
正在生成论文摘要