Food Recommendation as Language Processing (F-RLP): A Personalized and Contextual Paradigm
CoRR(2024)
摘要
State-of-the-art rule-based and classification-based food recommendation
systems face significant challenges in becoming practical and useful. This
difficulty arises primarily because most machine learning models struggle with
problems characterized by an almost infinite number of classes and a limited
number of samples within an unbalanced dataset. Conversely, the emergence of
Large Language Models (LLMs) as recommendation engines offers a promising
avenue. However, a general-purpose Recommendation as Language Processing (RLP)
approach lacks the critical components necessary for effective food
recommendations. To address this gap, we introduce Food Recommendation as
Language Processing (F-RLP), a novel framework that offers a food-specific,
tailored infrastructure. F-RLP leverages the capabilities of LLMs to maximize
their potential, thereby paving the way for more accurate, personalized food
recommendations.
更多查看译文
AI 理解论文
溯源树
样例
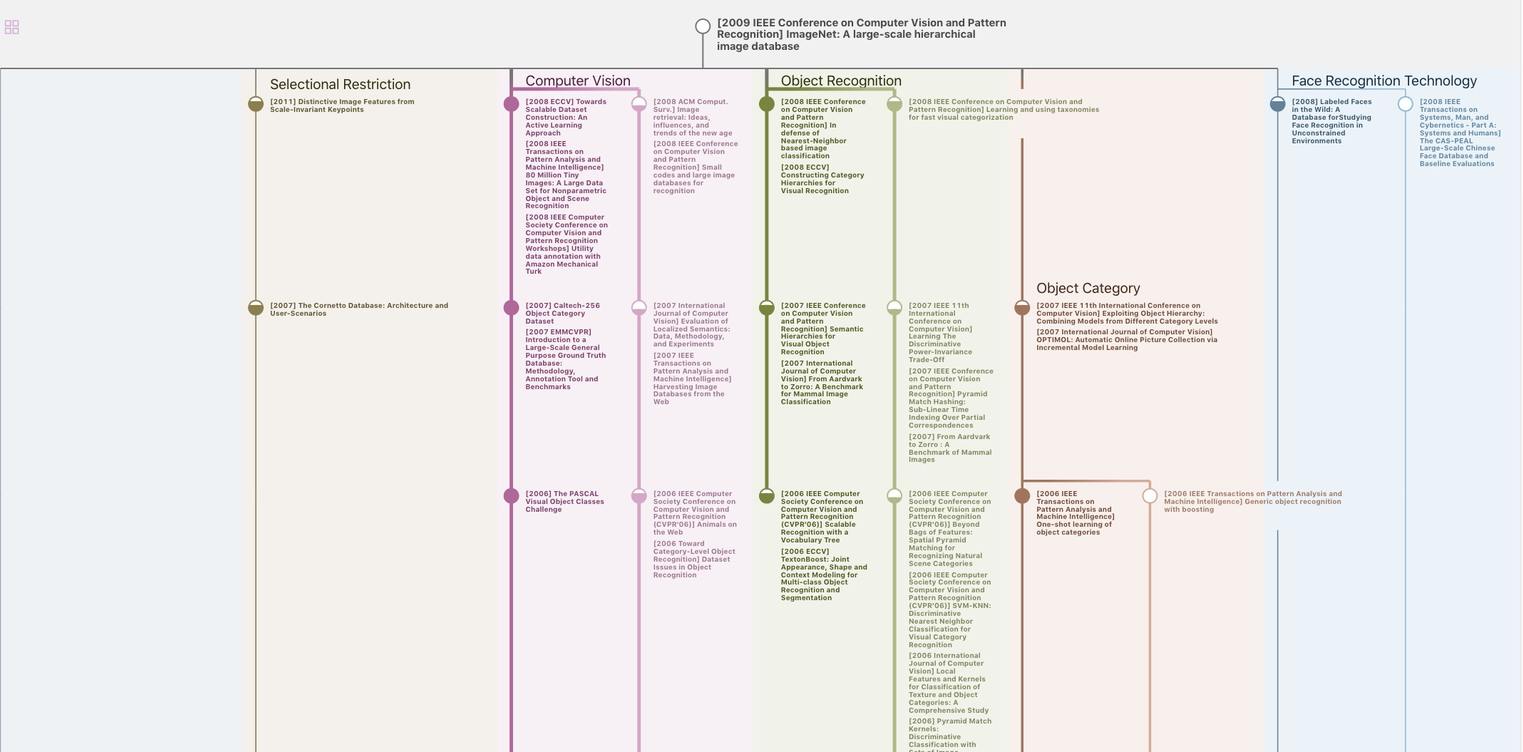
生成溯源树,研究论文发展脉络
Chat Paper
正在生成论文摘要