The Bias of Harmful Label Associations in Vision-Language Models
CoRR(2024)
Abstract
Despite the remarkable performance of foundation vision-language models, the
shared representation space for text and vision can also encode harmful label
associations detrimental to fairness. While prior work has uncovered bias in
vision-language models' (VLMs) classification performance across geography,
work has been limited along the important axis of harmful label associations
due to a lack of rich, labeled data. In this work, we investigate harmful label
associations in the recently released Casual Conversations datasets containing
more than 70,000 videos. We study bias in the frequency of harmful label
associations across self-provided labels for age, gender, apparent skin tone,
and physical adornments across several leading VLMs. We find that VLMs are
4-13x more likely to harmfully classify individuals with darker skin tones.
We also find scaling transformer encoder model size leads to higher confidence
in harmful predictions. Finally, we find improvements on standard vision tasks
across VLMs does not address disparities in harmful label associations.
MoreTranslated text
AI Read Science
Must-Reading Tree
Example
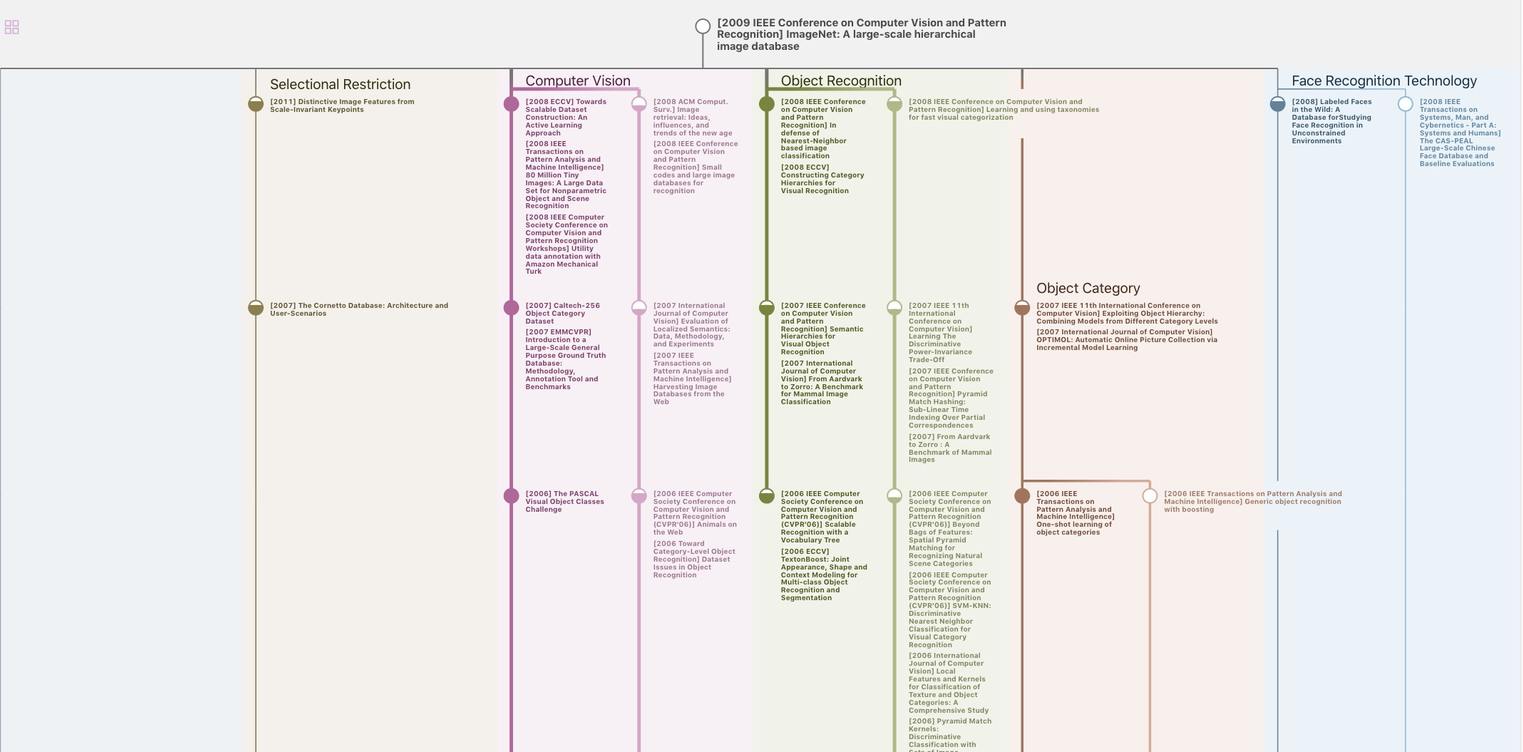
Generate MRT to find the research sequence of this paper
Chat Paper
Summary is being generated by the instructions you defined