Near-perfect Coverage Manifold Estimation in Cellular Networks via conditional GAN
IEEE Networking Letters(2024)
摘要
This paper presents a conditional generative adversarial network (cGAN) that
translates base station location (BSL) information of any Region-of-Interest
(RoI) to location-dependent coverage probability values within a subset of that
region, called the region-of-evaluation (RoE). We train our network utilizing
the BSL data of India, the USA, Germany, and Brazil. In comparison to the
state-of-the-art convolutional neural networks (CNNs), our model improves the
prediction error (L_1 difference between the coverage manifold generated by
the network under consideration and that generated via simulation) by two
orders of magnitude. Moreover, the cGAN-generated coverage manifolds appear to
be almost visually indistinguishable from the ground truth.
更多查看译文
关键词
Coverage,Network Performance,Conditional GAN,Stochastic Geometry
AI 理解论文
溯源树
样例
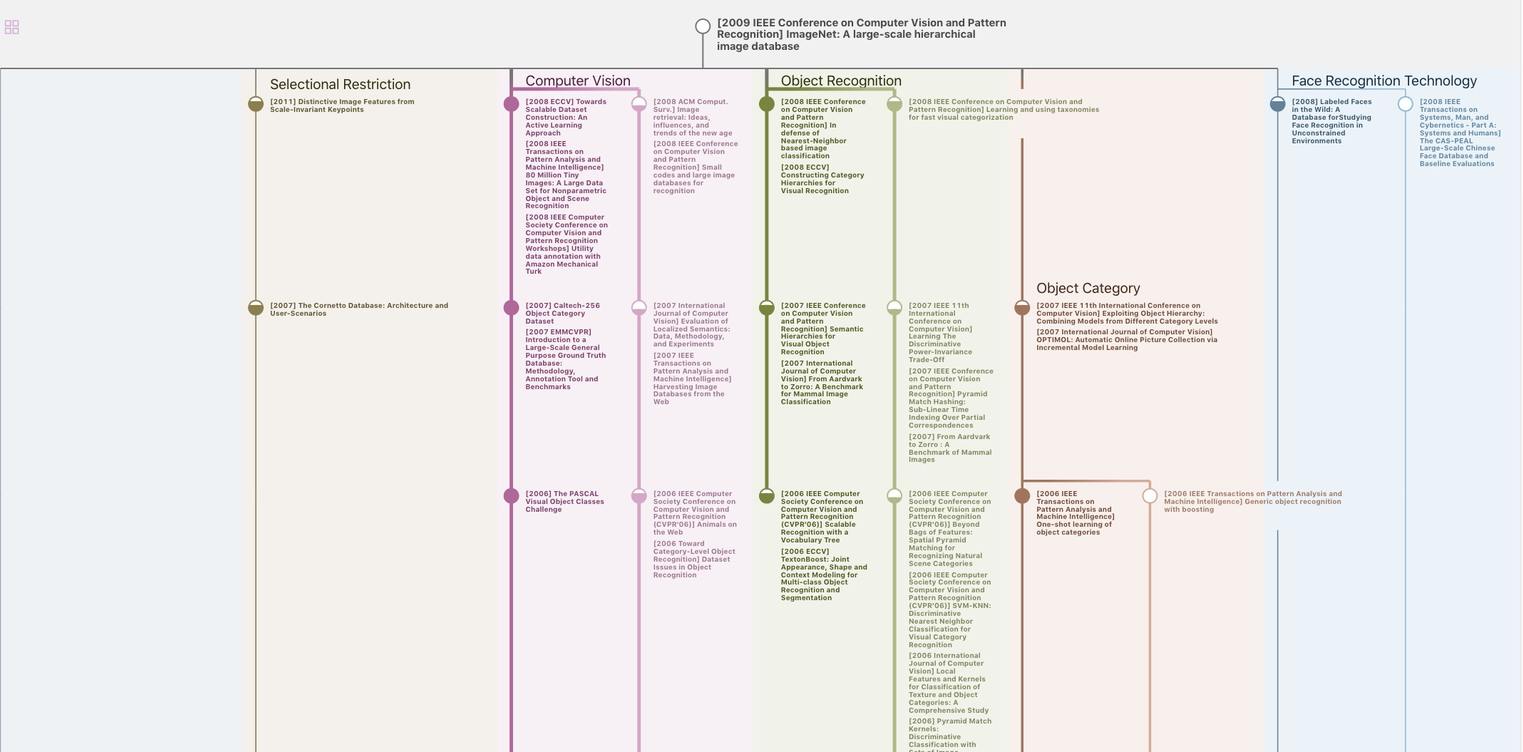
生成溯源树,研究论文发展脉络
Chat Paper
正在生成论文摘要