Resampling methods for Private Statistical Inference
CoRR(2024)
摘要
We consider the task of constructing confidence intervals with differential
privacy. We propose two private variants of the non-parametric bootstrap, which
privately compute the median of the results of multiple “little” bootstraps
run on partitions of the data and give asymptotic bounds on the coverage error
of the resulting confidence intervals. For a fixed differential privacy
parameter ϵ, our methods enjoy the same error rates as that of the
non-private bootstrap to within logarithmic factors in the sample size n. We
empirically validate the performance of our methods for mean estimation, median
estimation, and logistic regression with both real and synthetic data. Our
methods achieve similar coverage accuracy to existing methods (and non-private
baselines) while providing notably shorter (≳ 10 times) confidence
intervals than previous approaches.
更多查看译文
AI 理解论文
溯源树
样例
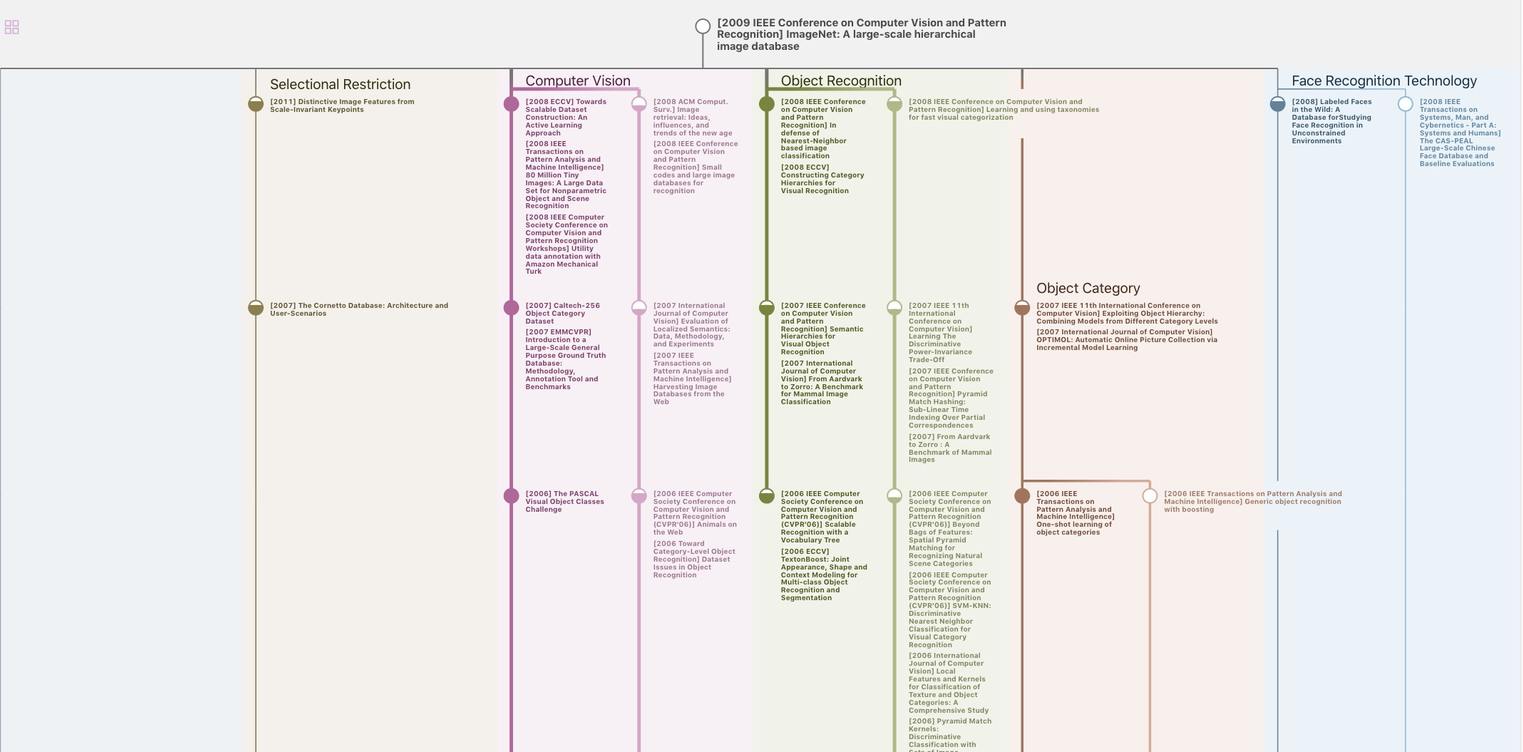
生成溯源树,研究论文发展脉络
Chat Paper
正在生成论文摘要