MS2OD: outlier detection using minimum spanning tree and medoid selection
MACHINE LEARNING-SCIENCE AND TECHNOLOGY(2024)
摘要
As an essential task in data mining, outlier detection identifies abnormal patterns in numerous applications, among which clustering-based outlier detection is one of the most popular methods for its effectiveness in detecting cluster-related outliers, especially in medical applications. This article presents an advanced method to extract cluster-based outliers by employing a scaled minimum spanning tree (MST) data structure and a new medoid selection method: 1. we compute a scaled MST and iteratively cut the current longest edge to obtain clusters; 2. we apply a new medoid selection method, considering the noise effect to improve the quality of cluster-based outlier identification. The experimental results on real-world data, including extensive medical corpora and other semantically meaningful datasets, demonstrate the wide applicability and outperforming metrics of the proposed method.
更多查看译文
关键词
outlier detection,data mining,medical data,machine learning,clustering,minimum spanning tree,medoid selection
AI 理解论文
溯源树
样例
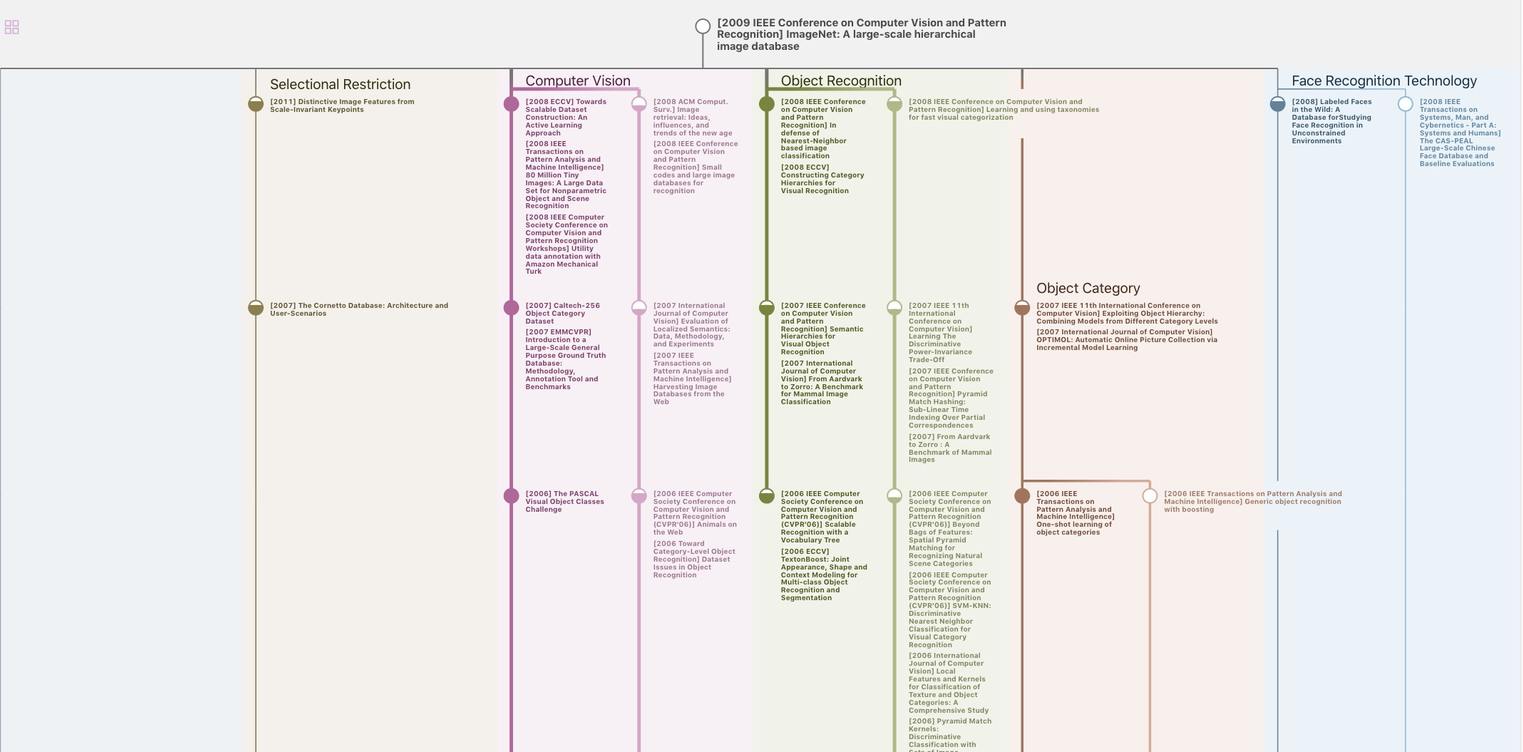
生成溯源树,研究论文发展脉络
Chat Paper
正在生成论文摘要