Data-driven Joint Detection and Localization of Acoustic Reflectors
CoRR(2024)
摘要
Room geometry inference algorithms rely on the localization of acoustic
reflectors to identify boundary surfaces of an enclosure. Rooms with highly
absorptive walls or walls at large distances from the measurement setup pose
challenges for such algorithms. As it is not always possible to localize all
walls, we present a data-driven method to jointly detect and localize acoustic
reflectors that correspond to nearby and/or reflective walls. A multi-branch
convolutional recurrent neural network is employed for this purpose. The
network's input consists of a time-domain acoustic beamforming map, obtained
via Radon transform from multi-channel room impulse responses. A modified loss
function is proposed that forces the network to pay more attention to walls
that can be estimated with a small error. Simulation results show that the
proposed method can detect nearby and/or reflective walls and improve the
localization performance for the detected walls.
更多查看译文
AI 理解论文
溯源树
样例
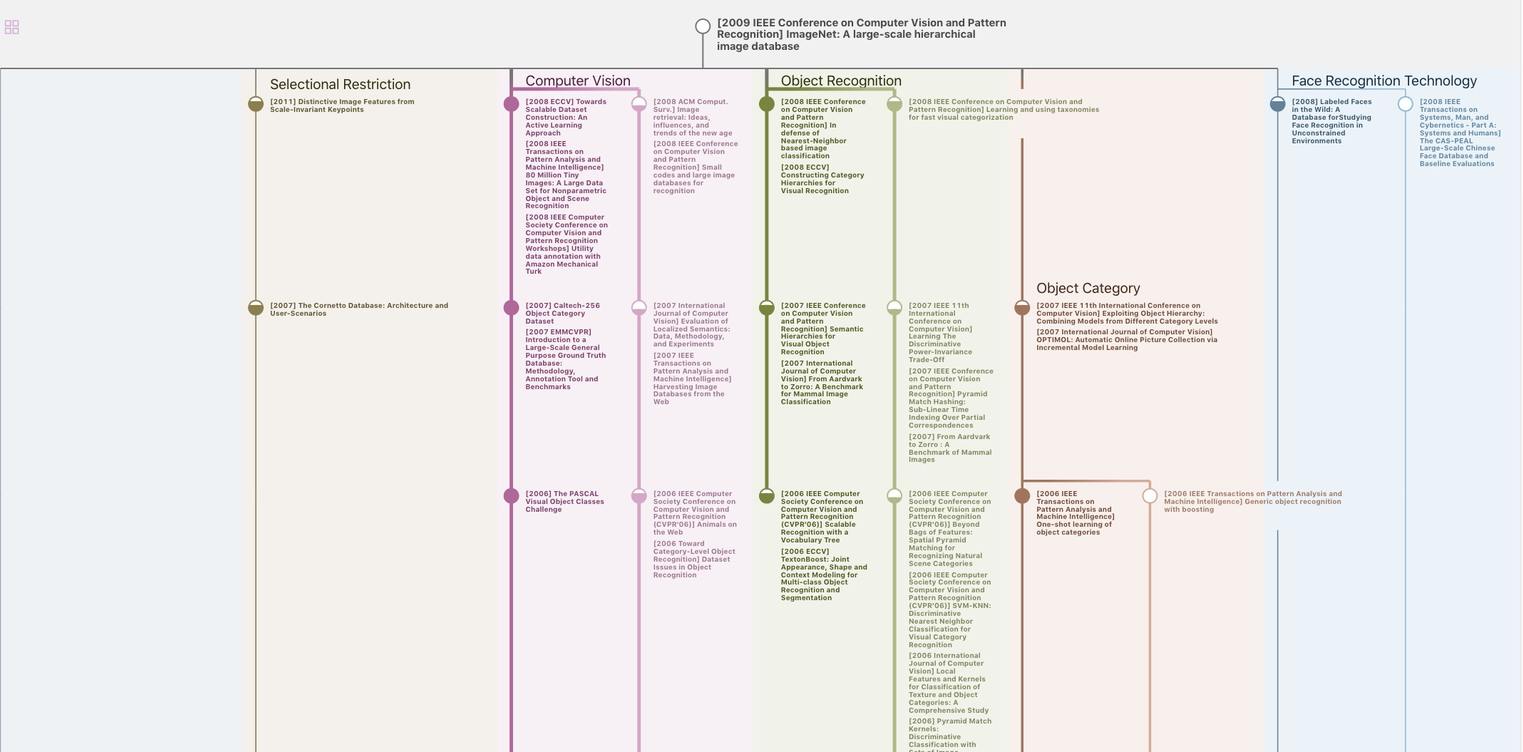
生成溯源树,研究论文发展脉络
Chat Paper
正在生成论文摘要