Mitral Valve Segmentation and Tracking from Transthoracic Echocardiography Using Deep Learning
ULTRASOUND IN MEDICINE AND BIOLOGY(2024)
摘要
Objective: Valvular heart diseases (VHDs) pose a significant public health burden, and deciding the best treatment strategy necessitates accurate assessment of heart valve function. Transthoracic echocardiography (TTE) is the key modality to evaluate VHDs, but the lack of standardized quantitative measurements leads to subjective and time-consuming assessments. We aimed to use deep learning to automate the extraction of mitral valve (MV) leaflets and annular hinge points from echocardiograms of the MV, improving standardization and reducing workload in quantitative assessment of MV disease. Methods: We annotated the MV leaflets and annulus points in 2931 images from 127 patients. We propose an approach for segmenting the annotated features using Attention UNet with deep supervision and weight scheduling of the attention coefficients to enforce saliency surrounding the MV. The derived segmentation masks were used to extract quantitative biomarkers for specific MV leaflet scallops throughout the heart cycle. Results: Evaluation performance was summarized using a Dice score of 0.63 +/- 0.14, annulus error of 3.64 +/- 2.53 and leaflet angle error of 8.7 +/- 8.3 degrees. Leveraging Attention UNet with deep supervision robustness of clinically relevant metrics was improved compared with UNet, reducing standard deviations by 2.7 degrees (angle error) and 0.73 mm (annulus error). We correctly identified cases of MV prolapse, cases of stenosis and healthy references from a clinical material using the derived biomarkers. Conclusion: Robust deep learning segmentation and tracking of MV morphology and motion is possible by leveraging attention gates and deep supervision, and holds promise for enhancing VHD diagnosis and treatment monitoring.
更多查看译文
关键词
Transthoracic echocardiography,Valvular heart disease,Mitral valve,Deep learning,Attention gates,Medical image segmentation,Tracking,Time series,Quantitative assessment
AI 理解论文
溯源树
样例
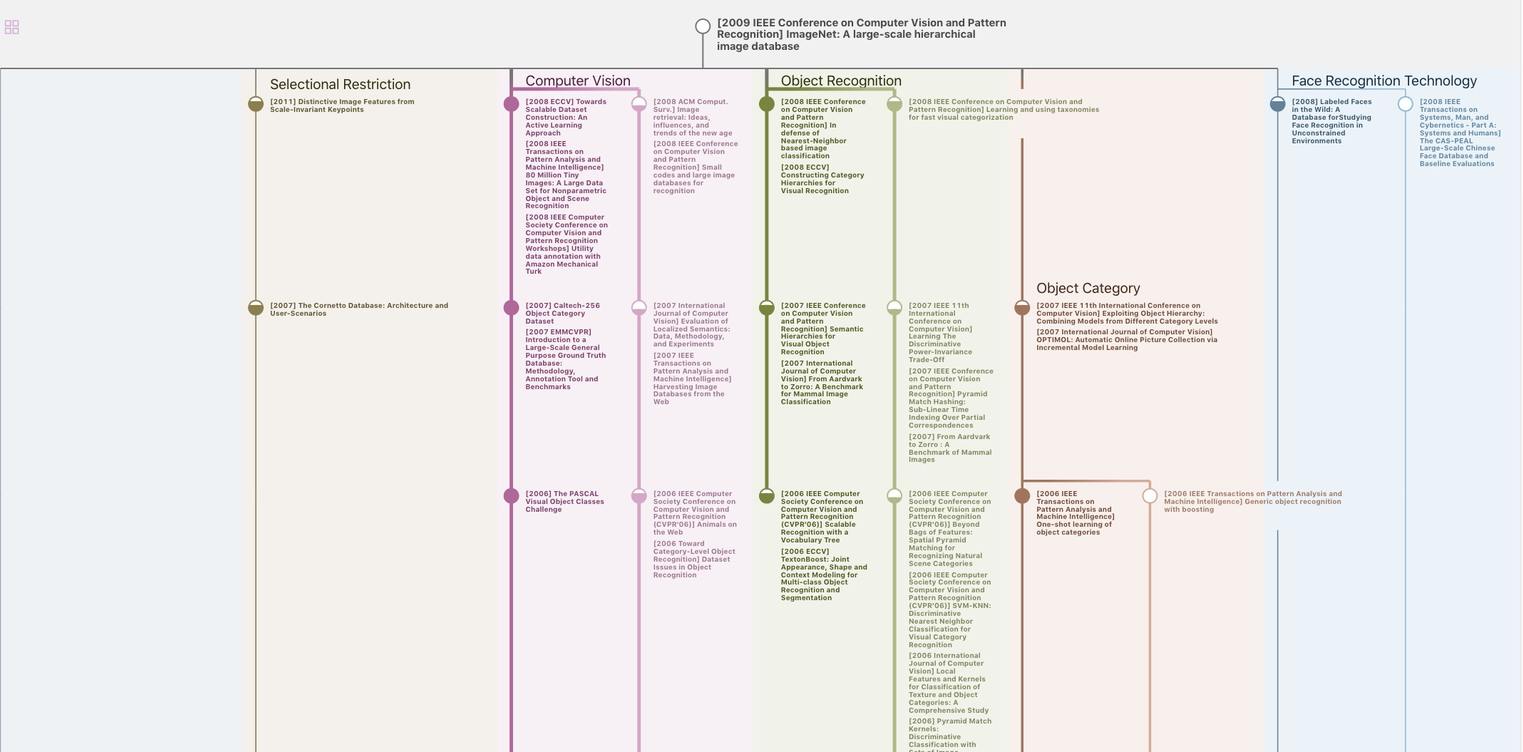
生成溯源树,研究论文发展脉络
Chat Paper
正在生成论文摘要