A machine learning algorithm for peripheral artery disease prognosis using biomarker data
ISCIENCE(2024)
摘要
Peripheral artery disease (PAD) biomarkers have been studied in isolation; however, an algorithm that considers a protein panel to inform PAD prognosis may improve predictive accuracy. Biomarker-based prediction models were developed and evaluated using a model development (n = 270) and prospective validation cohort (n = 277). Plasma concentrations of 37 proteins were measured at baseline and the patients were followed for 2 years. The primary outcome was 2 -year major adverse limb event (MALE; composite of vascular intervention or major amputation). Of the 37 proteins tested, 6 were differentially expressed in patients with vs. without PAD (ADAMTS13, ICAM-1, ANGPTL3, Alpha 1-microglobulin, GDF15, and endostatin). Using 10 -fold cross -validation, we developed a random forest machine learning model that accurately predicts 2 -year MALE in a prospective validation cohort of PAD patients using a 6 -protein panel (AUROC 0.84). This algorithm can support PAD risk stratification, informing clinical decisions on further vascular evaluation and management.
更多查看译文
关键词
Artificial intelligence,Cardiovascular medicine,Machine learning
AI 理解论文
溯源树
样例
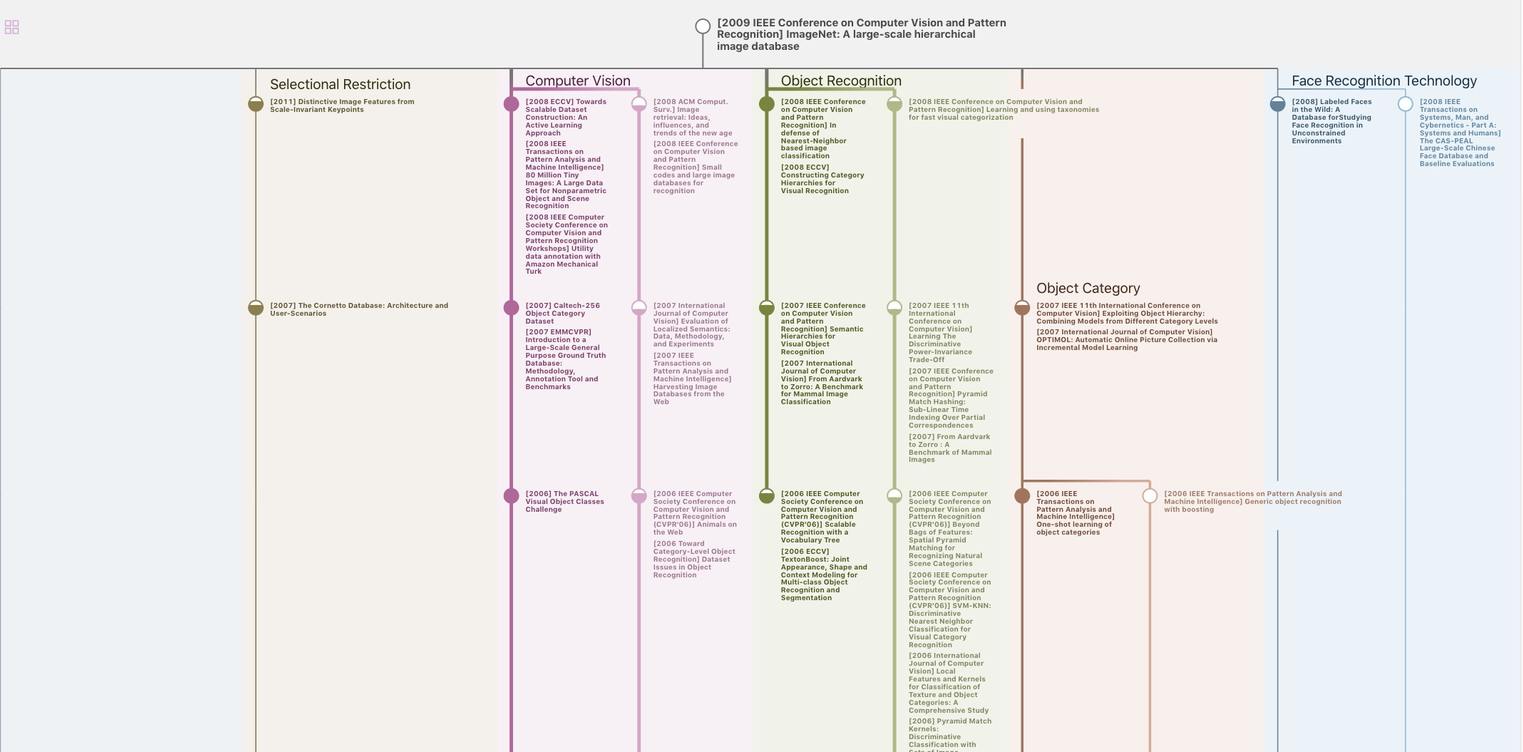
生成溯源树,研究论文发展脉络
Chat Paper
正在生成论文摘要