Ego-Noise Prediction Based on Multimodal Learning for Enhanced Discrimination in UAV Hammering Inspection.
2024 IEEE/SICE International Symposium on System Integration (SII)(2024)
摘要
In this paper, we propose an ego-noise prediction method applicable to Unmanned Aerial Vehicles (UAVs). Sensing sound using UAV has a significantly reduced Signal-to-Noise Ratio due to the strong ego-noise from the propellers and motors. Template-based ego-noise prediction for robots with pre-set movements, such as humanoid robots, has previously been studied. However, that approach is not applicable to UAVs which continuously adjust the propeller's rotation speed based on the situational requirements. Here, we propose a novel framework for ego-noise prediction by focusing on propellers, which are the main ego-noise source, and translating the modality of propeller vibrations into the modality of ego-noise sound. Assuming a strong relation between the vibration frequency of a propeller and the ego-noise it generates, we propose using a Recurrent Neural Network to predict the ego-noise spectrogram, using vibration frequency as a feature. The prediction accuracy of ego-noise spectrograms was at a level that suggests the potential for effective ego-noise reduction, and the results are promising for applications in UAV hammering inspection.
更多查看译文
关键词
Unmanned Aerial Vehicles,Neural Network,Spectroscopic,Rotational Speed,Recurrent Neural Network,Humanoid Robot,Time Series,Deep Neural Network,Prediction Error,Accelerometer,Training Phase,Time Resolution,Microphone,Angular Frequency,Reverberation,Environmental Noise,Gated Recurrent Unit,Revolutions Per Minute,Bias Term,Short-time Fourier Transform
AI 理解论文
溯源树
样例
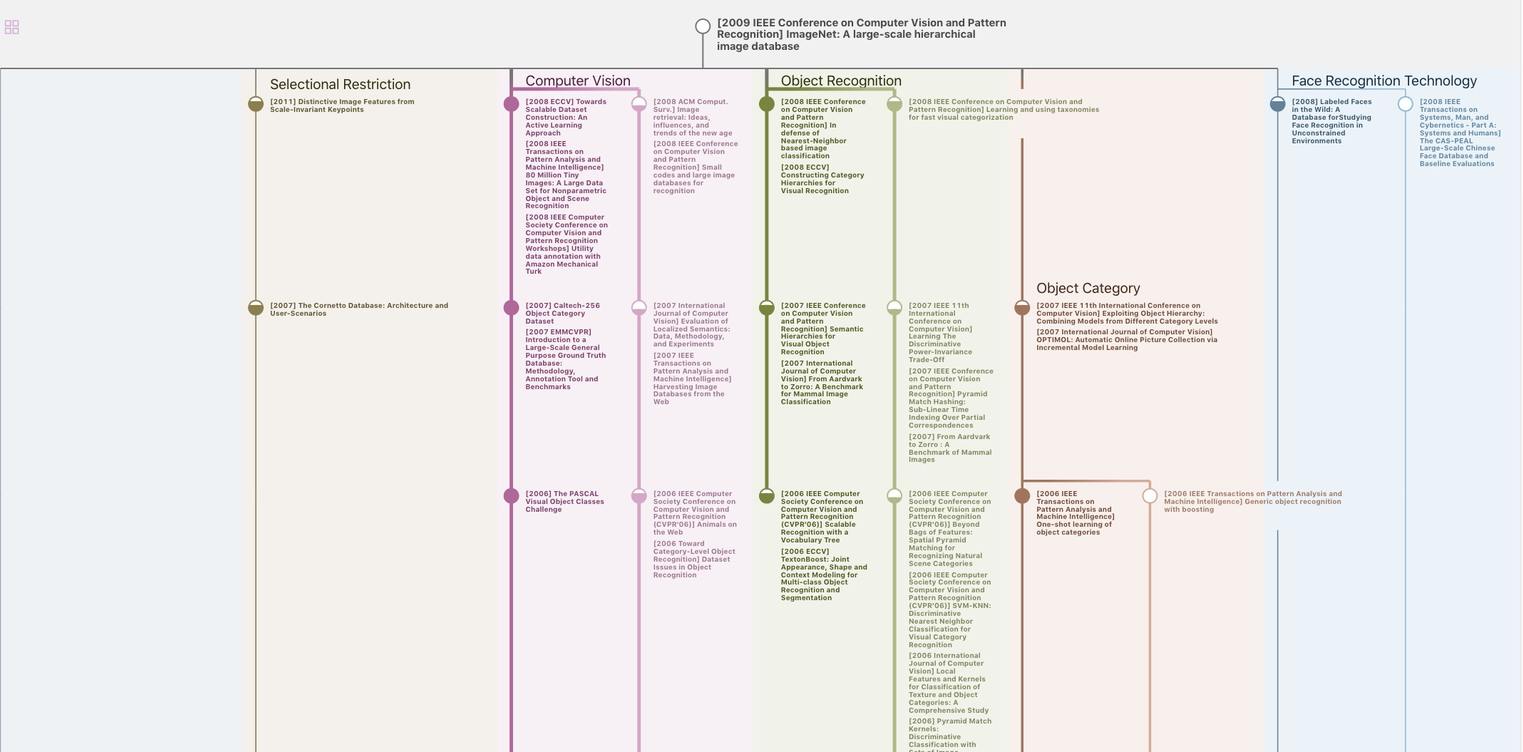
生成溯源树,研究论文发展脉络
Chat Paper
正在生成论文摘要