Mixed Reality-Based 6D-Pose Annotation System for Robot Manipulation in Retail Environments.
2024 IEEE/SICE International Symposium on System Integration (SII)(2024)
摘要
Robot manipulation in retail environments is a challenging task due to the need for large amounts of annotated data for accurate 6D-pose estimation of items. Onsite data collection, additional manual annotation, and model fine-tuning are often required when deploying robots in new environments, as varying lighting conditions, clutter, and occlusions can significantly diminish performance. Therefore, we propose a system to annotate the 6D pose of items using mixed reality (MR) to enhance the robustness of robot manipulation in retail environments. Our main contribution is a system that can display 6D-pose estimation results of a trained model from multiple perspectives in MR, and enable onsite (re-)annotation of incorrectly inferred item poses using hand gestures. The proposed system is compared to a PC-based annotation system using a mouse and the robot camera's point cloud in an extensive quantitative experiment. Our experimental results indicate that MR can increase the accuracy of pose annotation, especially by reducing position errors.
更多查看译文
关键词
Robot Manipulator,Annotation System,Retail Environment,6D Pose Annotations,Large Amount Of Data,Point Cloud,Multiple Perspectives,Position Error,Hand Gestures,Additional Annotations,Estimation Results Of Model,Mixed Reality,Varying Lighting Conditions,Robots In Environments,Need For Large Amounts,Experimental Protocol,Ecosystem Services,Average Error,Bounding Box,Single Viewpoint,Rotation Error,Service Robots,Deep Learning-based Methods,Depth Camera,Human-robot Interaction,Ground Truth Pose,Error Reduction,RGB Images,Symmetry Axis
AI 理解论文
溯源树
样例
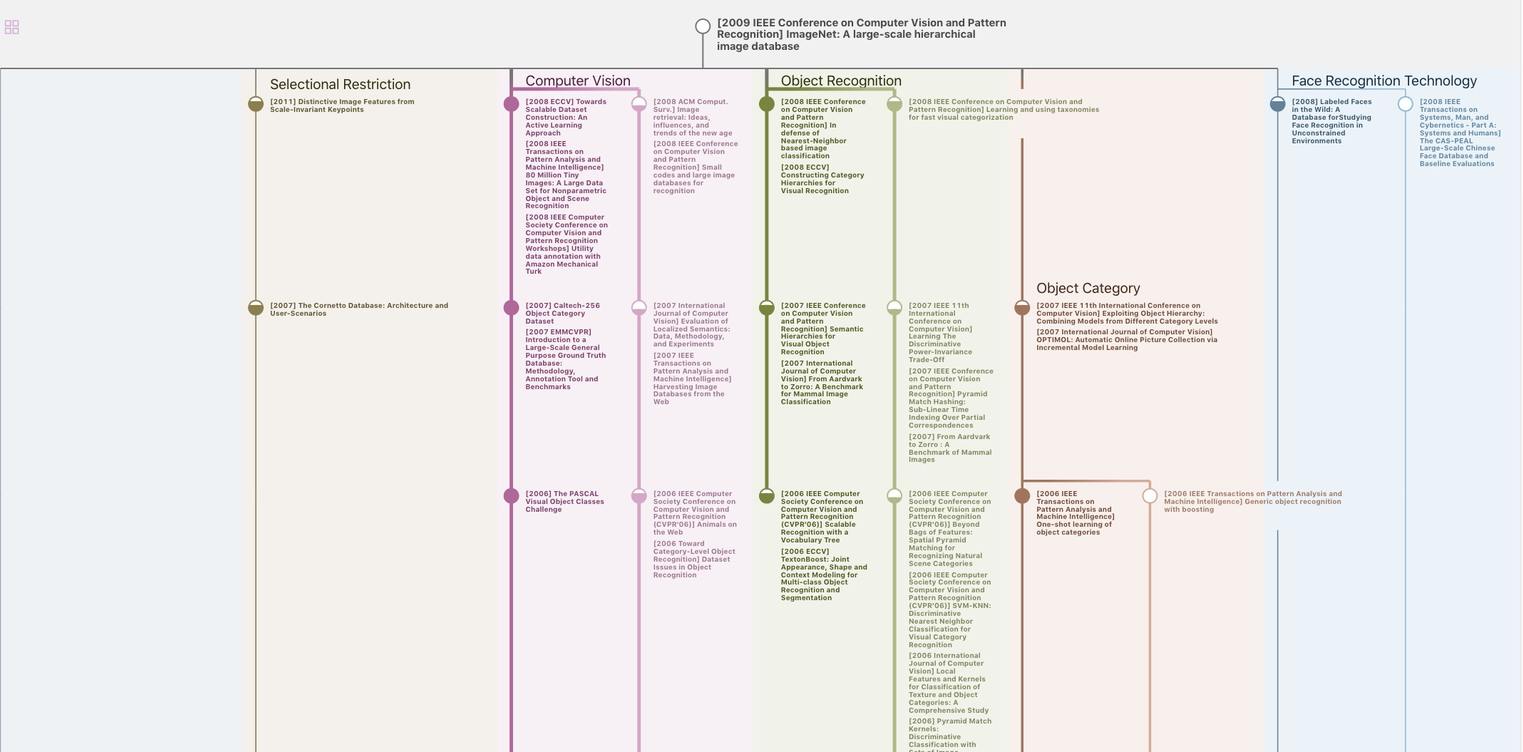
生成溯源树,研究论文发展脉络
Chat Paper
正在生成论文摘要