Efficient Sample Collection to Construct Observation Models for Contact-Based Object Pose Estimation
2024 IEEE/SICE International Symposium on System Integration (SII)(2024)
Key words
Sample Collection,Pose Estimation,Human Pose Estimation,Object Pose,Visual Information,Object Shape,Tactile Sensor,Tactile Information,Soft Sensor,Sample Collection Process,Random Sampling,True Value,Cost Function,Grid Search,Gaussian Process,Particle Filter,Active Sensors,Search Efficiency,Fewer Samples,Acquisition Function,Sensor Values,Bayesian Optimization,World Coordinate System,Bayesian Filtering,Sensor Model,Entropy Of Distribution,Robotic Hand,Hand Position,Black-box Function,Action Condition
AI Read Science
Must-Reading Tree
Example
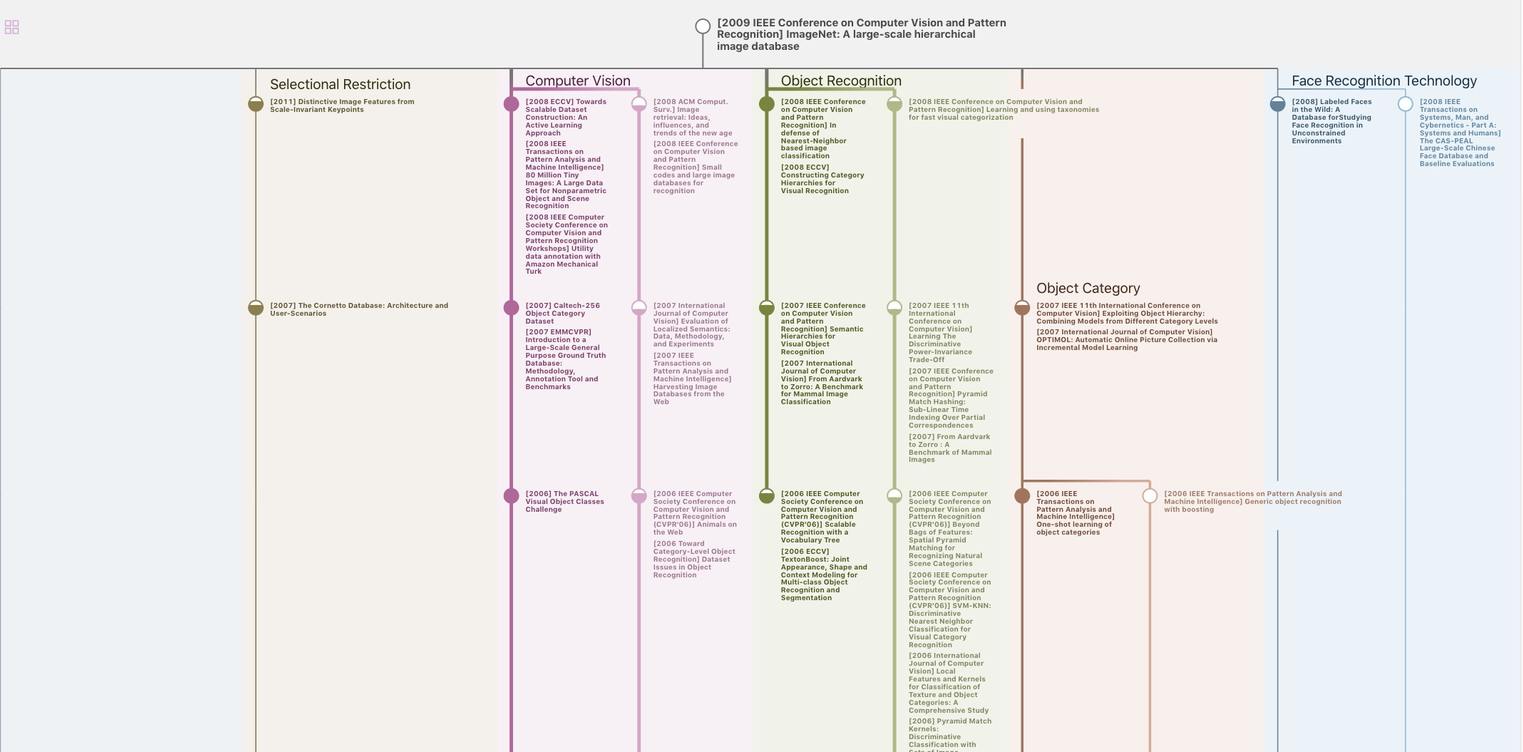
Generate MRT to find the research sequence of this paper
Chat Paper
Summary is being generated by the instructions you defined