Characterizing Manipulator Motion Using an Evolving Type 2 Quantum Fuzzy Neural Network.
2024 IEEE/SICE International Symposium on System Integration (SII)(2024)
摘要
This paper presents a control algorithm based on the Evolving Type 2 Quantum Fuzzy Neural Network (ET2QFNN) for characterizing and controlling the joint-space motion of a manipulator. The approach integrates ET2QFNN, a hybrid neural network model that combines principles from quantum fuzzy sets and neural networks, to address the inherent complexity and uncertainty associated with manipulator systems. Unlike traditional control methods, the ET2QFNN-based approach learns and adapts through simulations of closed-loop torque control, incorporating joint position commands and corresponding motion data. By utilizing data from previous cycles, the ET2QFNN enhances the manipulator's performance and stability, particularly in repetitive tasks. To assess its effectiveness, a comparative analysis is conducted, contrasting the approach with finely tuned proportional-derivative (PD) control and integral sliding mode control (iSMC) in scenarios with and without non-parametric uncertainties. The results demonstrate the unique advantages and superior performance of the ET2QFNN-based control algorithm in addressing these challenges.
更多查看译文
关键词
Joint-space motion model,Evolving Type 2 Quantum Fuzzy Neural Network (ET2QFNN),Manipulator,Closed-loop torque controller,Non-parametric uncertainties
AI 理解论文
溯源树
样例
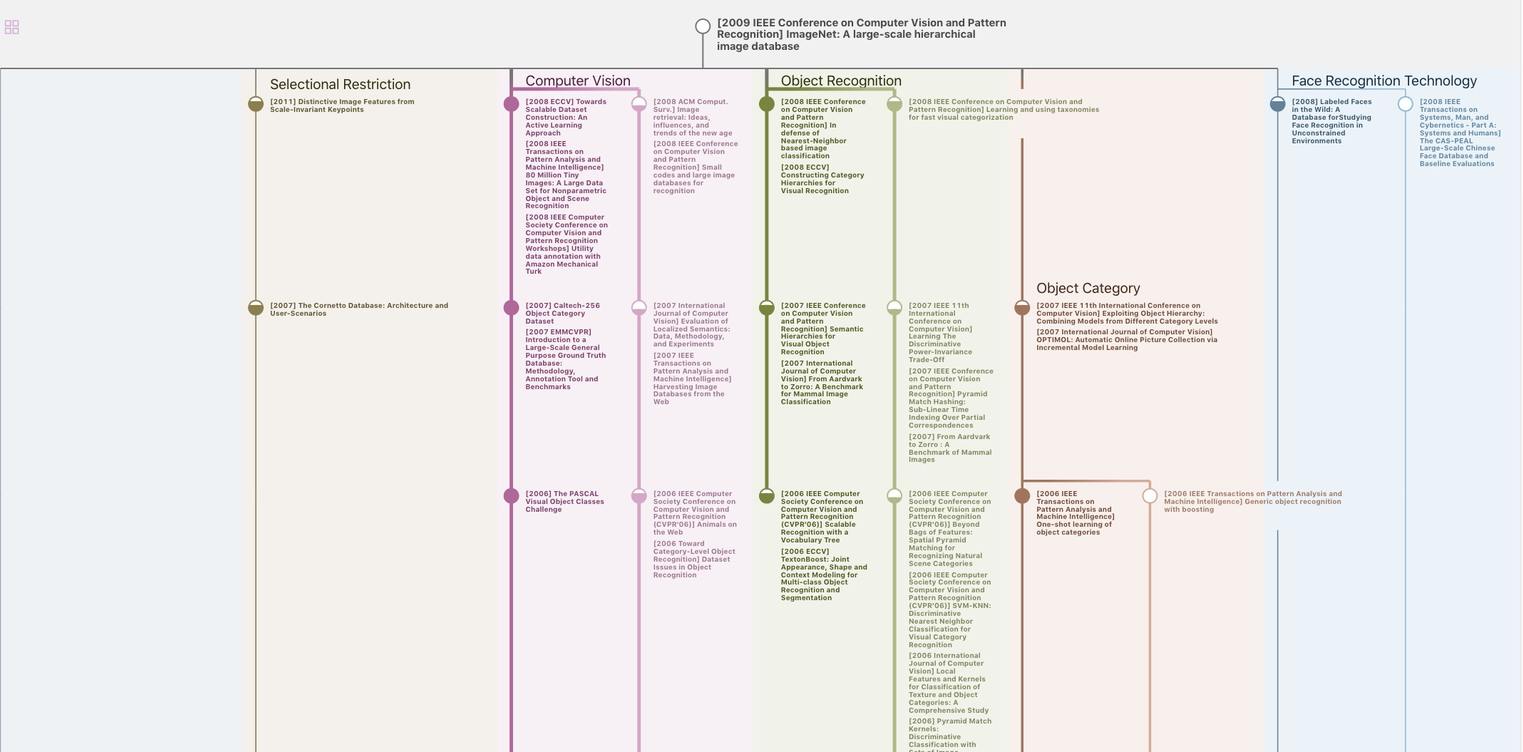
生成溯源树,研究论文发展脉络
Chat Paper
正在生成论文摘要