Multi-Person Pose Regression with Distribution-Aware Single-Stage Models.
IEEE transactions on pattern analysis and machine intelligence(2024)
摘要
Understanding human posture is a challenging topic, which encompasses several tasks, e.g., pose estimation, body mesh recovery and pose tracking. In this paper, we propose a novel Distribution-Aware Single-stage (DAS) model for the pose-related tasks. The proposed DAS model estimates human position and localizes joints simultaneously, which requires only a single pass. Meanwhile, we utilize normalizing flow to enable DAS to learn the true distribution of joint locations, rather than making simple Gaussian or Laplacian assumptions. This provides a pivotal prior and greatly boosts the accuracy of regression-based methods, thus making DAS achieve comparable performance to the volumetric-based methods. We also introduce a recursively update strategy to progressively approach the regression target, reducing the difficulty of regression and improving the regression performance. We further adapt DAS to multi-person mesh recovery and pose tracking tasks and achieve considerable performance on both tasks. Comprehensive experiments on CMU Panoptic and MuPoTS-3D demonstrate the superior efficiency of DAS, specifically 1.5 times speedup over previous best method, and its state-of-the-art accuracy for multi-person pose estimation. Extensive experiments on 3DPW and PoseTrack2018 indicate the effectiveness and efficiency of DAS for human body mesh recovery and pose tracking, respectively, which prove the generality of our proposed DAS model.
更多查看译文
关键词
Multi-Person Pose Estimation,Single-Stage Model,Normalizing Flow,Recursive Update Strategy
AI 理解论文
溯源树
样例
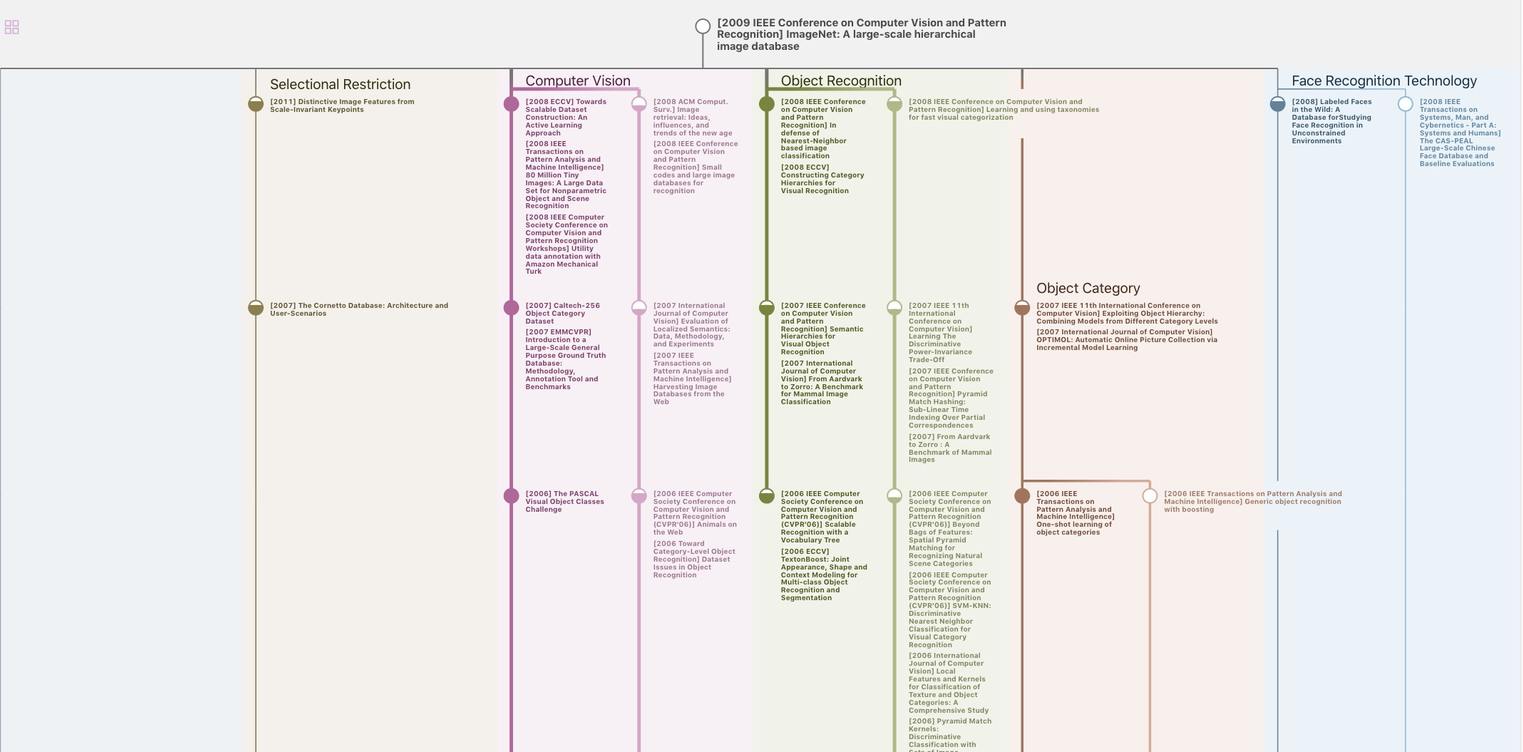
生成溯源树,研究论文发展脉络
Chat Paper
正在生成论文摘要