Enhancing DDoS attack detection with hybrid feature selection and ensemble-based classifier: A promising solution for robust cybersecurity
Measurement: Sensors(2024)
摘要
Distributed denial-of-service (DDoS) attacks pose a significant threat to computer networks and systems by disrupting services through the saturation of targeted systems with traffic from multiple sources. Real-time detection of these attacks has become a critical cybersecurity task. However, current DDoS attack detection methods suffer from high false positive rates and limited ability to capture the complex patterns of attack traffic. This research proposes an enhanced approach for detecting DDoS attacks using a hybrid feature selection technique in combination with an ensemble-based classifiers. The ensemble-based approach aggregates many decision trees to increase classification accuracy and reduce overfitting and model robustness. The feature selection technique uses correlation analysis, mutual information, and principal component analysis to identify the most useful characteristics for attack detection. The ensemble-based Random Forest classifier from the various ensemble-based approaches with the specified relevant features produces the best detection rates. Many datasets related to identifying DDoS attacks are used to evaluate the proposed model, and experimental findings demonstrate that it surpasses existing techniques in terms of accuracy, recall, precision, f1-score, and false positive rate, with other evaluation metrics. The proposed approach achieves almost 100 % accuracy, 100 % true positive rate, and 0 % error rate making it a promising solution for DDoS attack detection.
更多查看译文
关键词
DDoS attack detection,Hybrid feature selection to identify DDoS attacks,Ensemble-based approach to detect DDoS attacks,Ensemble random forest in cybersecurity
AI 理解论文
溯源树
样例
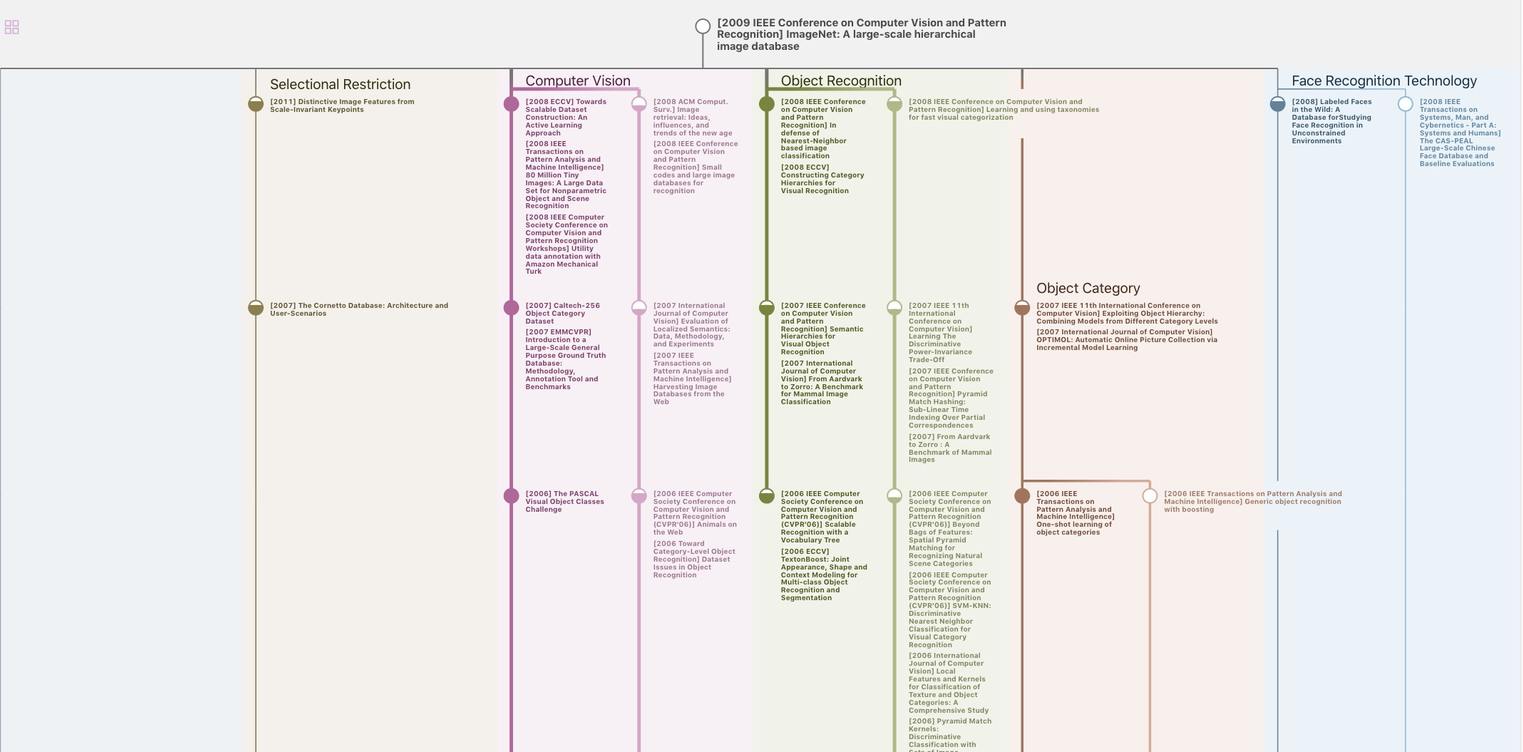
生成溯源树,研究论文发展脉络
Chat Paper
正在生成论文摘要