Predicting product quality and optimising process design using dynamic time warping in batch processes with varying batch times
Case Studies in Chemical and Environmental Engineering(2024)
摘要
In this study, the proposed method combines dynamic time warping with a genetic algorithm, enabling the construction of a robust machine learning model tailored for batch process data characterised by diverse batch and sampling times. This comprehensive model incorporates crucial factors, including initial process conditions, time-series data of process variables, and product quality. Furthermore, it allows for the simultaneous design of both initial process conditions and time-series data of the process variables (that is, batch profiles) for achieving the target values of predefined product quality. By leveraging actual semi-batch process data for polymer production, we confirmed that the proposed method can accurately predict the product quality during and at the end of the batch process. Concurrently, it facilitates the design of reasonable initial process conditions and batch profiles.
更多查看译文
关键词
Batch design,Batch optimisation,Batch profile,Endpoint,Dynamic time warping,Genetic algorithm,Soft sensor
AI 理解论文
溯源树
样例
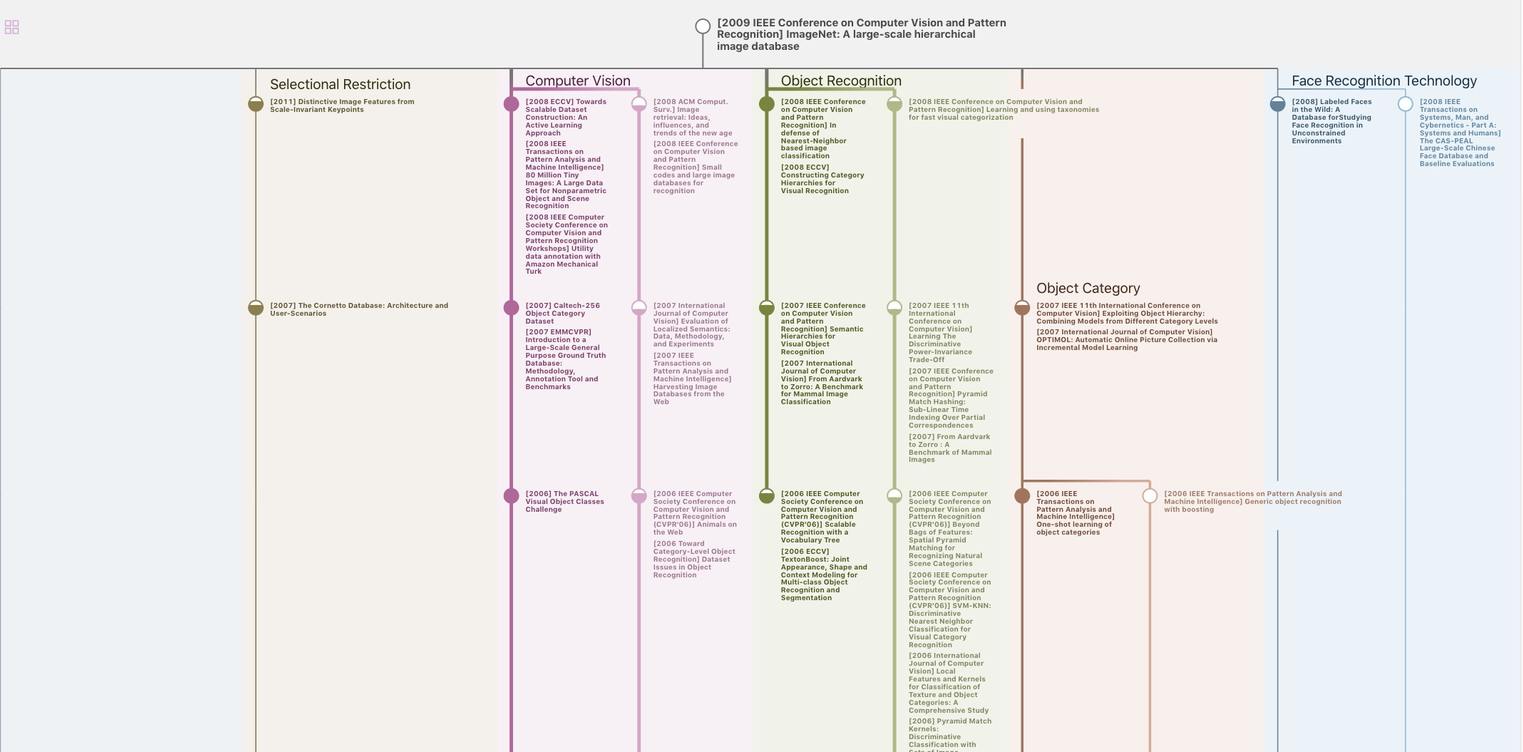
生成溯源树,研究论文发展脉络
Chat Paper
正在生成论文摘要