Seismic Coherent Noise Removal With Residual Network and Synthetic Seismic Samples.
IEEE Geoscience and Remote Sensing Letters(2024)
摘要
Seismic coherent noise is often found in poststack seismic data, which contaminates the resolution and integrity of seismic images. It is difficult to remove the coherent noise since the features of coherent noise, e.g., frequency, are highly related to signals. Recently, deep learning has proven to be uniquely advantageous in image denoise problems. To enhance the quality of the poststack seismic image, in this letter, we propose a novel deep-residual-learning-based neural network named DR-Unet to efficiently learn the features of seismic coherent noise. It includes an encoder branch and a decoder branch. Moreover, in order to collect enough training data, we propose a workflow that adds real seismic noise into synthetic seismic data to construct the training data. Experiments show that the proposed method can achieve good denoising results in both synthetic and field seismic data, even better than the traditional method.
更多查看译文
关键词
Coherent noise,deep learning,residual learning,seismic data denoising,synthetic training samples
AI 理解论文
溯源树
样例
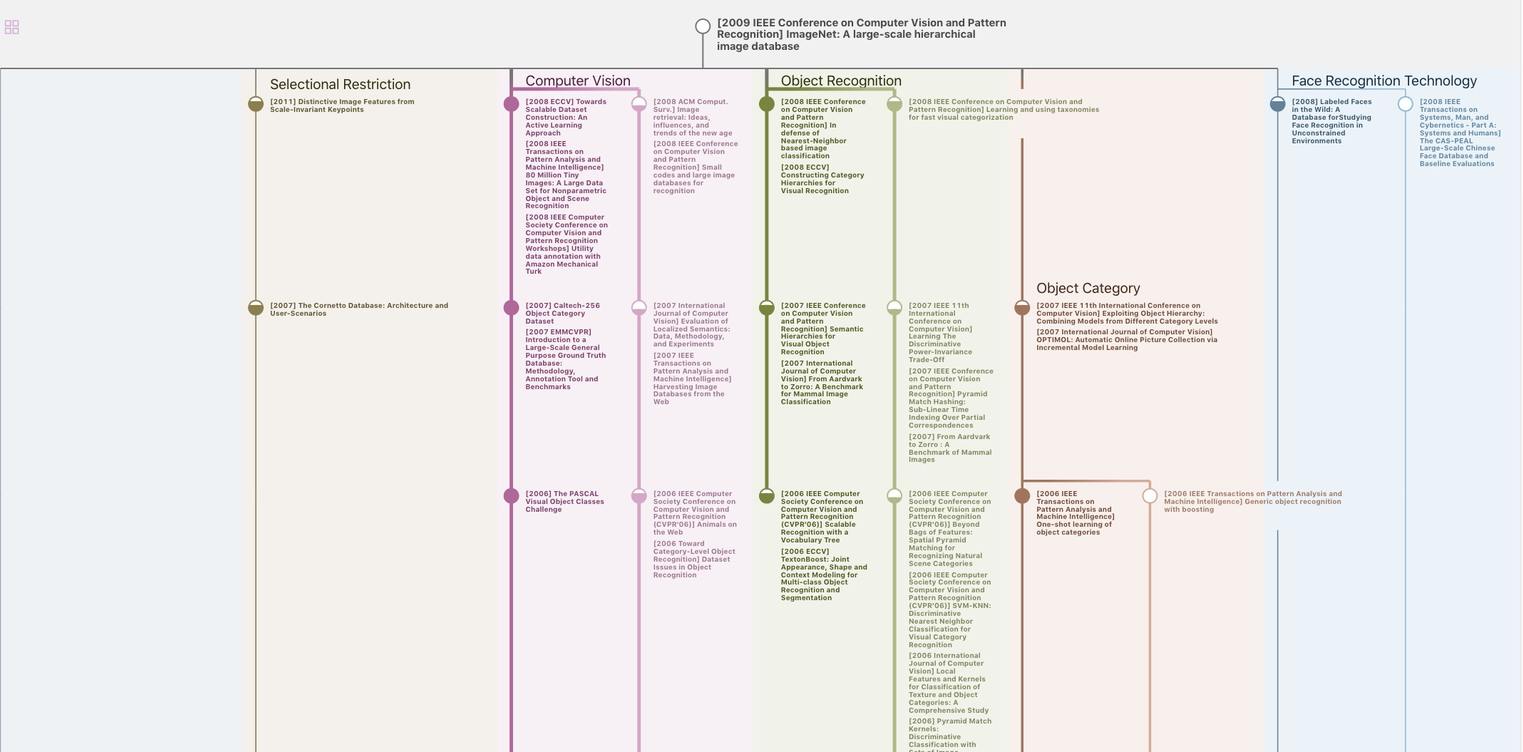
生成溯源树,研究论文发展脉络
Chat Paper
正在生成论文摘要