A Radar Echo Simulator for the Synthesis of Randomized Training Data Sets in the Context of AI-Based Applications
SENSORS(2024)
摘要
Supervised machine learning algorithms usually require huge labeled data sets to produce sufficiently good results. For many applications, these data sets are still not available today, and the reasons for this can be manifold. As a solution, the missing training data can be generated by fast simulators. This procedure is well studied and allows filling possible gaps in the training data, which can further improve the results of a machine learning model. For this reason, this article deals with the development of a two-dimensional electromagnetic field simulator for modeling the response of a radar sensor in an imaging system based on the synthetic aperture radar principle. The creation of completely random scenes is essential to achieve data sets with large variance. Therefore, special emphasis is placed on the development of methods that allow creating random objects, which can then be assembled into an entire scene. In the context of this contribution, we focus on humanitarian demining with regard to improvised explosive devices using a ground-penetrating radar system. This is an area where the use of trained classifiers is of great importance, but in practice, there are little to no labeled datasets for the training process. The simulation results show good agreement with the measurement results obtained in a previous contribution, demonstrating the possibility of enhancing sparse training data sets with synthetic data.
更多查看译文
关键词
computational EM,SAR,ground-penetrating radar (GPR),landmine detection,full-wave simulation
AI 理解论文
溯源树
样例
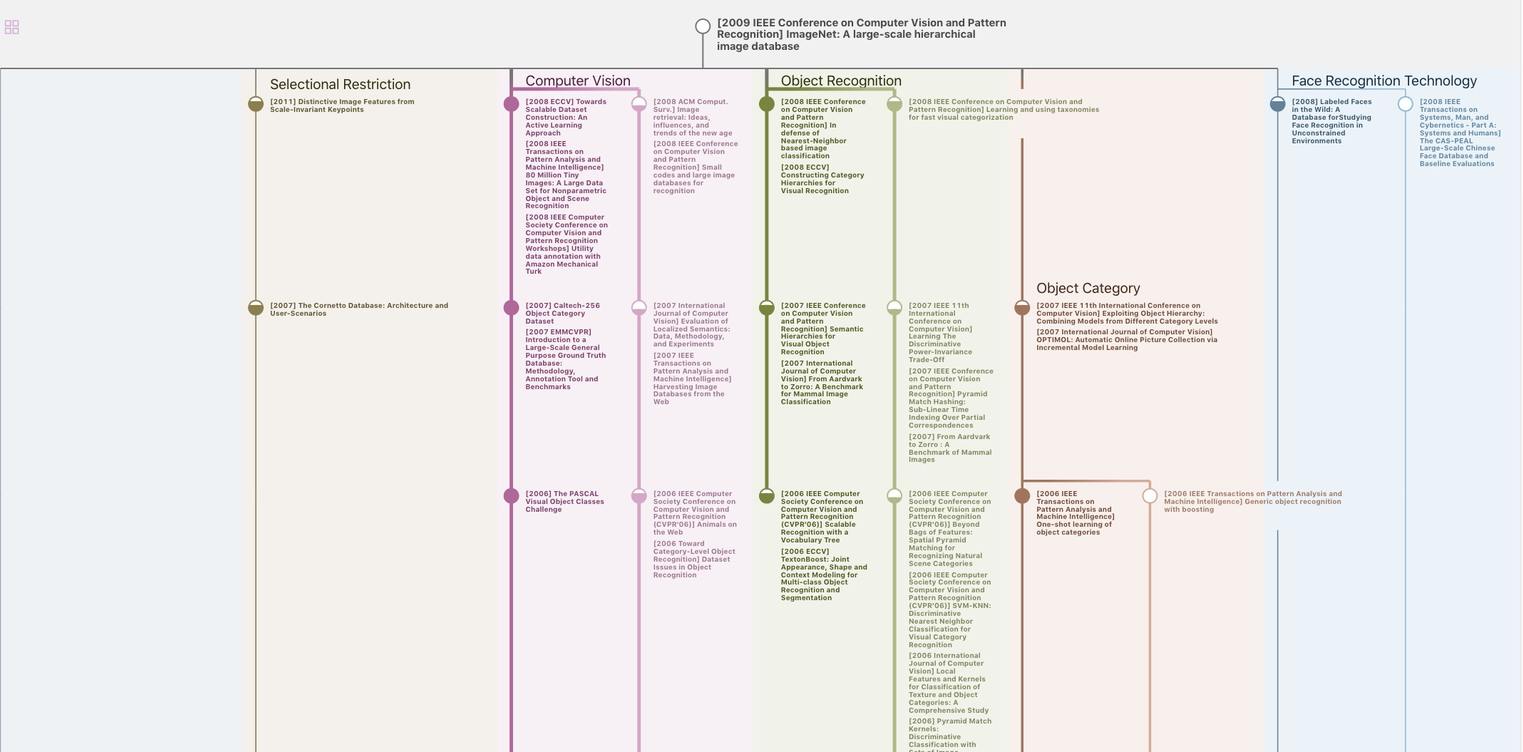
生成溯源树,研究论文发展脉络
Chat Paper
正在生成论文摘要