A Novel Respiratory Rate Estimation Algorithm from Photoplethysmogram Using Deep Learning Model
DIAGNOSTICS(2024)
摘要
Respiratory rate (RR) is a critical vital sign that can provide valuable insights into various medical conditions, including pneumonia. Unfortunately, manual RR counting is often unreliable and discontinuous. Current RR estimation algorithms either lack the necessary accuracy or demand extensive window sizes. In response to these challenges, this study introduces a novel method for continuously estimating RR from photoplethysmogram (PPG) with a reduced window size and lower processing requirements. To evaluate and compare classical and deep learning algorithms, this study leverages the BIDMC and CapnoBase datasets, employing the Respiratory Rate Estimation (RRest) toolbox. The optimal classical techniques combination on the BIDMC datasets achieves a mean absolute error (MAE) of 1.9 breaths/min. Additionally, the developed neural network model utilises convolutional and long short-term memory layers to estimate RR effectively. The best-performing model, with a 50% train-test split and a window size of 7 s, achieves an MAE of 2 breaths/min. Furthermore, compared to other deep learning algorithms with window sizes of 16, 32, and 64 s, this study's model demonstrates superior performance with a smaller window size. The study suggests that further research into more precise signal processing techniques may enhance RR estimation from PPG signals.
更多查看译文
关键词
photoplethysmogram,respiratory rate,deep learning,neural network
AI 理解论文
溯源树
样例
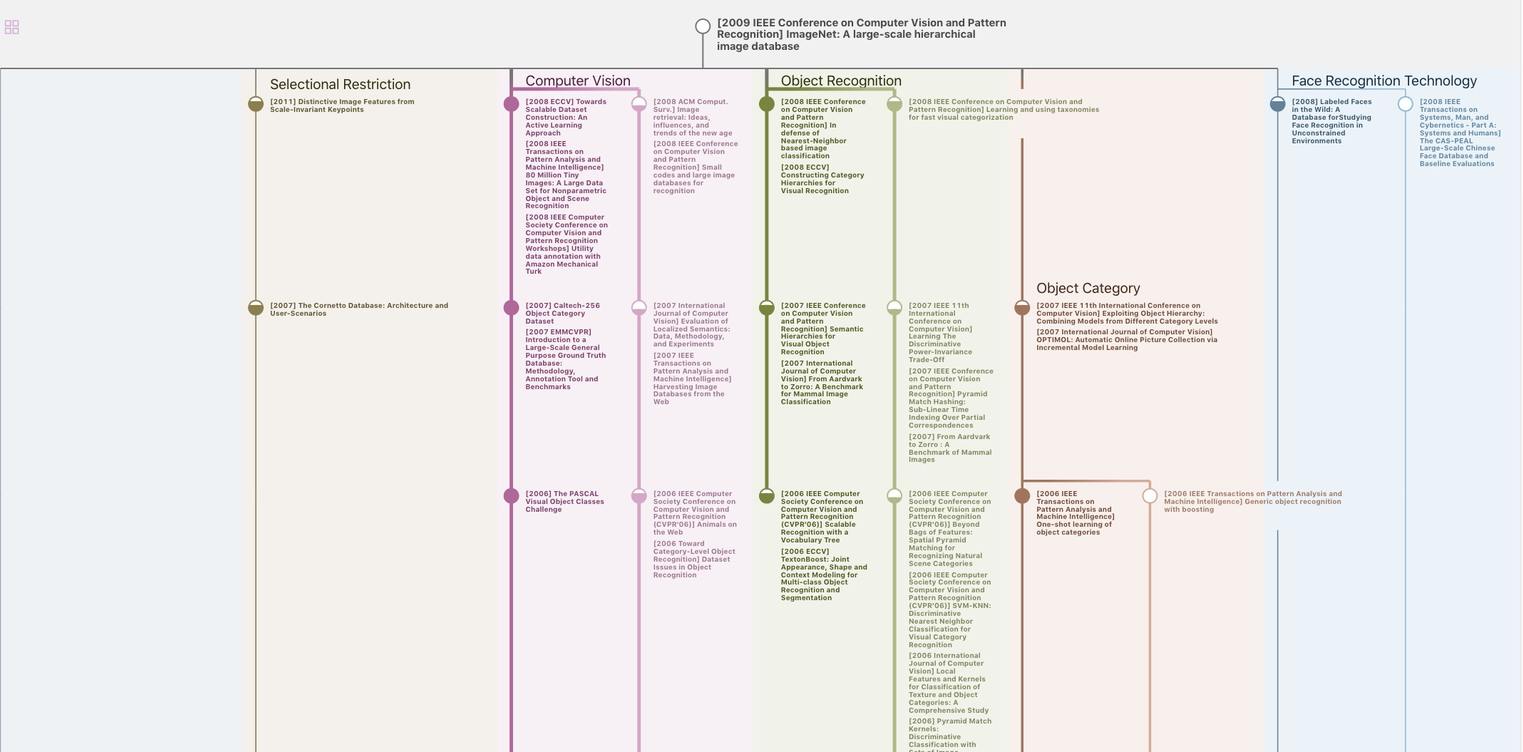
生成溯源树,研究论文发展脉络
Chat Paper
正在生成论文摘要