Square-Based Black-Box Adversarial Attack on Time Series Classification Using Simulated Annealing and Post-Processing-Based Defense
ELECTRONICS(2024)
摘要
While deep neural networks (DNNs) have been widely and successfully used for time series classification (TSC) over the past decade, their vulnerability to adversarial attacks has received little attention. Most existing attack methods focus on white-box setups, which are unrealistic as attackers typically only have access to the model's probability outputs. Defensive methods also have limitations, relying primarily on adversarial retraining which degrades classification accuracy and requires excessive training time. On top of that, we propose two new approaches in this paper: (1) A simulated annealing-based random search attack that finds adversarial examples without gradient estimation, searching only on the l(infinity)-norm hypersphere of allowable perturbations. (2) A post-processing defense technique that periodically reverses the trend of corresponding loss values while maintaining the overall trend, using only the classifier's confidence scores as input. Experiments applying these methods to InceptionNet models trained on the UCR dataset benchmarks demonstrate the effectiveness of the attack, achieving up to 100% success rates. The defense method provided protection against up to 91.24% of attacks while preserving prediction quality. Overall, this work addresses important gaps in adversarial TSC by introducing novel black-box attack and lightweight defense techniques.
更多查看译文
关键词
time series classification,adversarial attack,adversarial attack defense
AI 理解论文
溯源树
样例
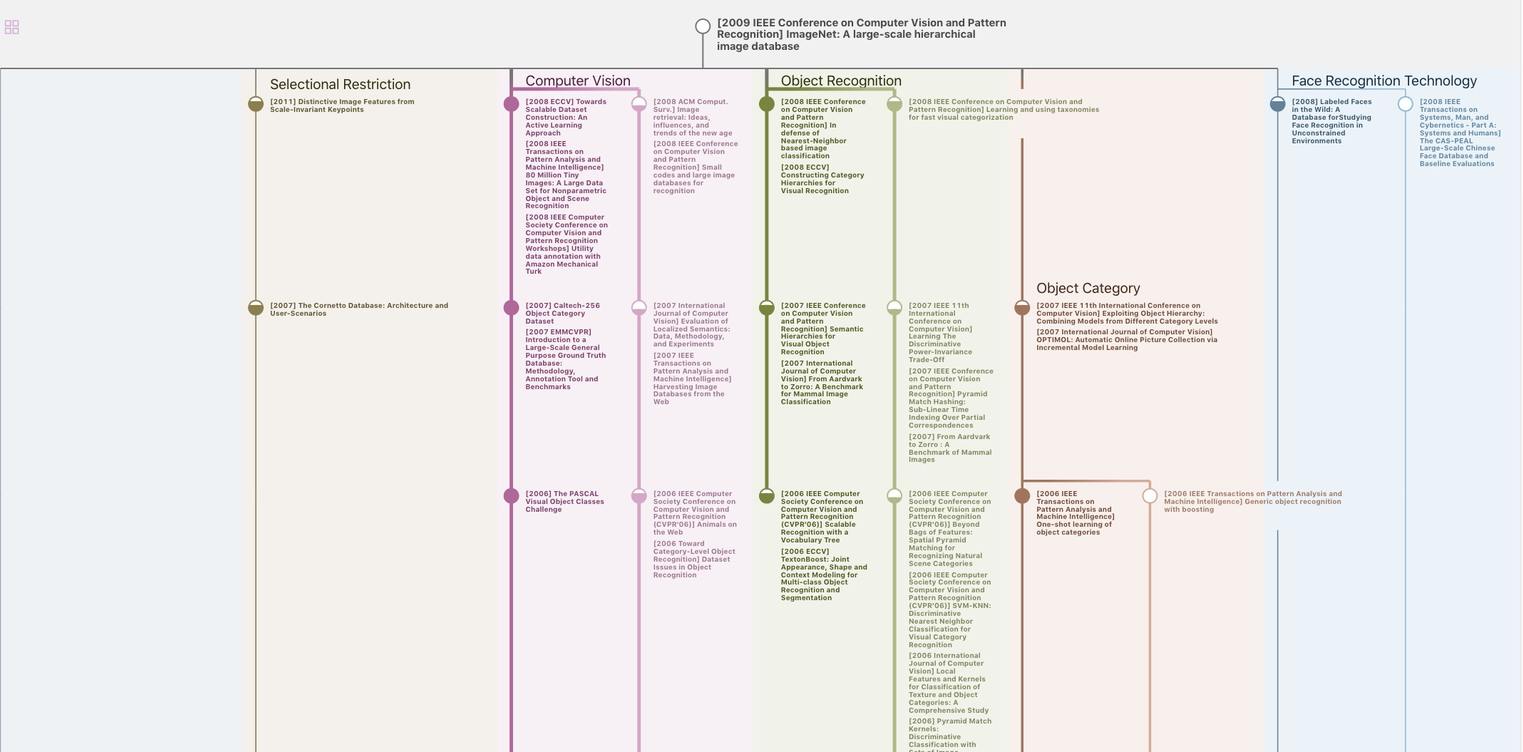
生成溯源树,研究论文发展脉络
Chat Paper
正在生成论文摘要