Evaluating Reinforcement Learning Algorithms in Residential Energy Saving and Comfort Management
Energies(2024)
摘要
The challenge of maintaining optimal comfort in residents while minimizing energy consumption has long been a focal point for researchers and practitioners. As technology advances, reinforcement learning (RL)—a branch of machine learning where algorithms learn by interacting with the environment—has emerged as a prominent solution to this challenge. However, the modern literature exhibits a plethora of RL methodologies, rendering the selection of the most suitable one a significant challenge. This work focuses on evaluating various RL methodologies for saving energy while maintaining adequate comfort levels in a residential setting. Five prominent RL algorithms—Proximal Policy Optimization (PPO), Deep Deterministic Policy Gradient (DDPG), Deep Q-Network (DQN), Advantage Actor-Critic (A2C), and Soft Actor-Critic (SAC)—are being thoroughly compared towards a baseline conventional control approach, exhibiting their potential to improve energy use while ensuring a comfortable living environment. The integrated comparison between the different RL methodologies emphasizes the subtle strengths and weaknesses of each algorithm, indicating that the best selection relies heavily on particular energy and comfort objectives.
更多查看译文
关键词
reinforcement learning,energy efficiency,thermal comfort,buildings,residents,Energym
AI 理解论文
溯源树
样例
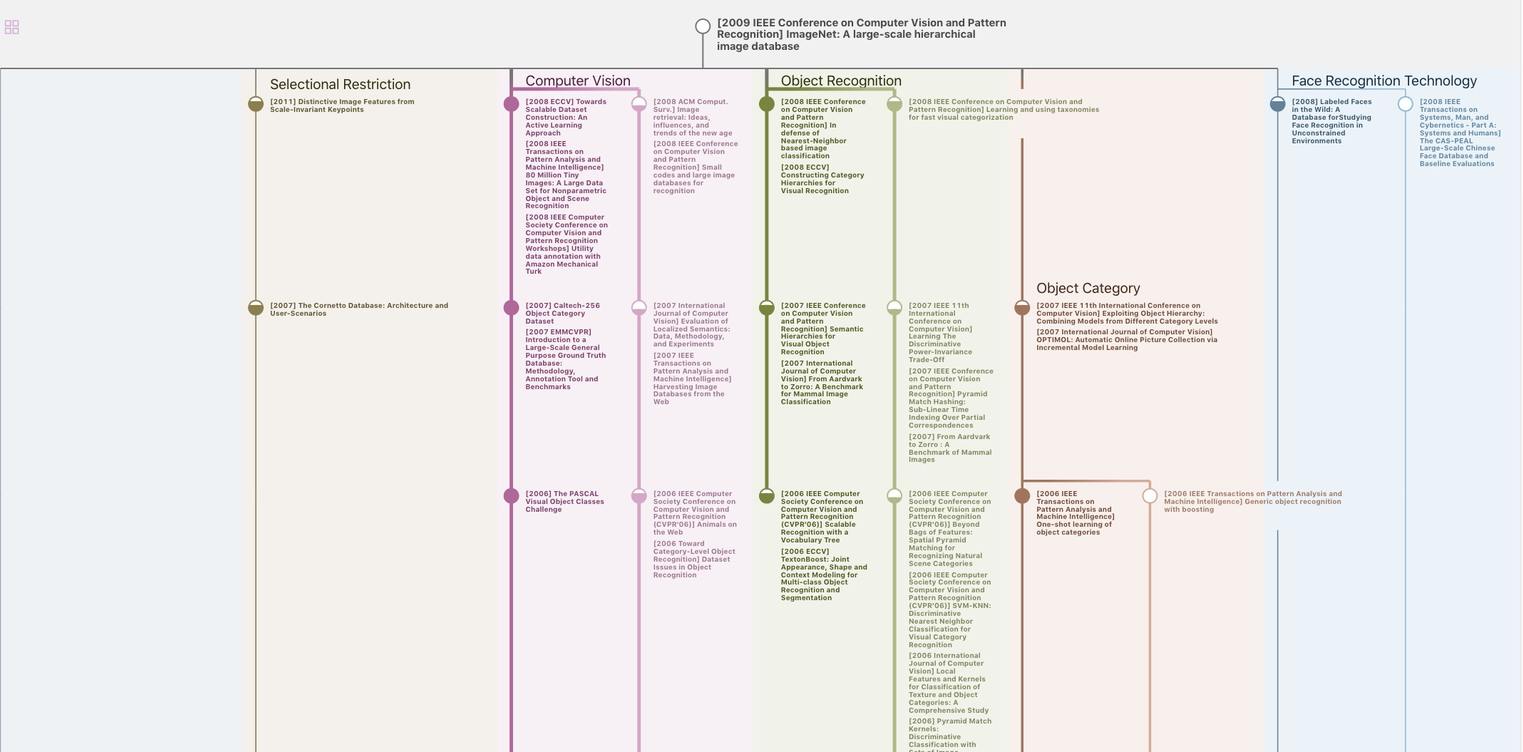
生成溯源树,研究论文发展脉络
Chat Paper
正在生成论文摘要