Built Environment Renewal Strategies Aimed at Improving Metro Station Vitality via the Interpretable Machine Learning Method: A Case Study of Beijing
SUSTAINABILITY(2024)
摘要
Understanding the built environment's impact on metro ridership is essential for developing targeted strategies for built environment renewal. Taking into consideration the limitations of existing studies, such as not proposing targeted strategies, using unified pedestrian catchment areas (PCA), and not determining the model's accuracy, Beijing was divided into three zones from inside to outside by the distribution pattern of metro stations. Three PCAs were assumed for each zone and a total of 27 PCA combinations. The study compared the accuracy of the Ordinary Least Square (OLS) and several machine learning models under each PCA combination to determine the model to be used in this study and the recommended PCA combination for the three zones. Under the recommended PCA combinations for the three zones, the model with the highest accuracy was used to explore the built environment's impact on metro ridership. Finally, prioritized stations for renewal were identified based on ridership and the built environment's impact on metro ridership. The results are as follows: (1) The eXtreme Gradient Boosting (XGBoost) model has a higher accuracy and was appropriate for this study. The recommended PCA combination for the three zones in Beijing was 1000 m_1200 m_1800 m. (2) During the morning peak hours, the density of office and apartment facilities greatly influenced the ridership, with a strong threshold effect and spatial heterogeneity. Our research framework also provides a new way for other cities to determine the scope of Transit-Oriented Development (TOD) and proposes a new decision-making method for improving the vibrancy of metro stations.
更多查看译文
关键词
built environment,renewal strategies,pedestrian catchment areas (PCA),machine learning,eXtreme Gradient Boosting (XGBoost),metro station vitality
AI 理解论文
溯源树
样例
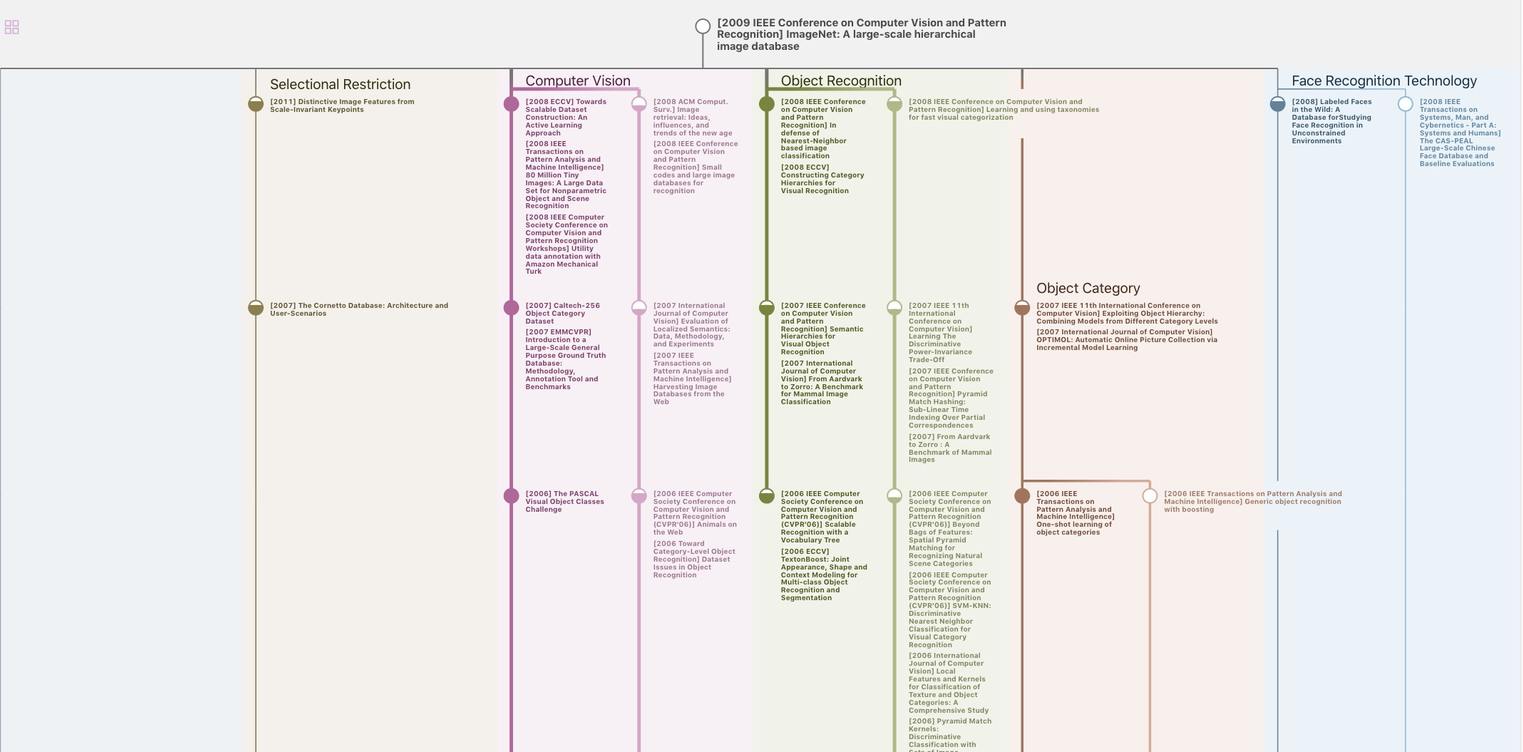
生成溯源树,研究论文发展脉络
Chat Paper
正在生成论文摘要