LDnADMM-Net: A Denoising Unfolded Deep Neural Network for Direction-of-Arrival Estimations in A Low Signal-to-Noise Ratio
REMOTE SENSING(2024)
摘要
In this paper, we explore the problem of direction-of-arrival (DOA) estimation for a non-uniform linear array (NULA) under strong noise. The compressed sensing (CS)-based methods are widely used in NULA DOA estimations. However, these methods commonly rely on the tuning of parameters, which are hard to fine-tune. Additionally, these methods lack robustness under strong noise. To address these issues, this paper proposes a novel DOA estimation approach using a deep neural network (DNN) for a NULA in a low SNR. The proposed network is designed based on the denoising convolutional neural network (DnCNN) and the alternating direction method of multipliers (ADMM), which is dubbed as LDnADMM-Net. First, we construct an unfolded DNN architecture that mimics the behavior of the iterative processing of an ADMM. In this way, the parameters of an ADMM can be transformed into the network weights, and thus we can adaptively optimize these parameters through network training. Then, we employ the DnCNN to develop a denoising module (DnM) and integrate it into the unfolded DNN. Using this DnM, we can enhance the anti-noise ability of the proposed network and obtain a robust DOA estimation in a low SNR. The simulation and experimental results show that the proposed LDnADMM-Net can obtain high-accuracy and super-resolution DOA estimations for a NULA with strong robustness in a low signal-to-noise ratio (SNR).
更多查看译文
关键词
direction-of-arrival (DOA) estimation,non-uniform linear array (NULA),deep neural network (DNN),signal denoising
AI 理解论文
溯源树
样例
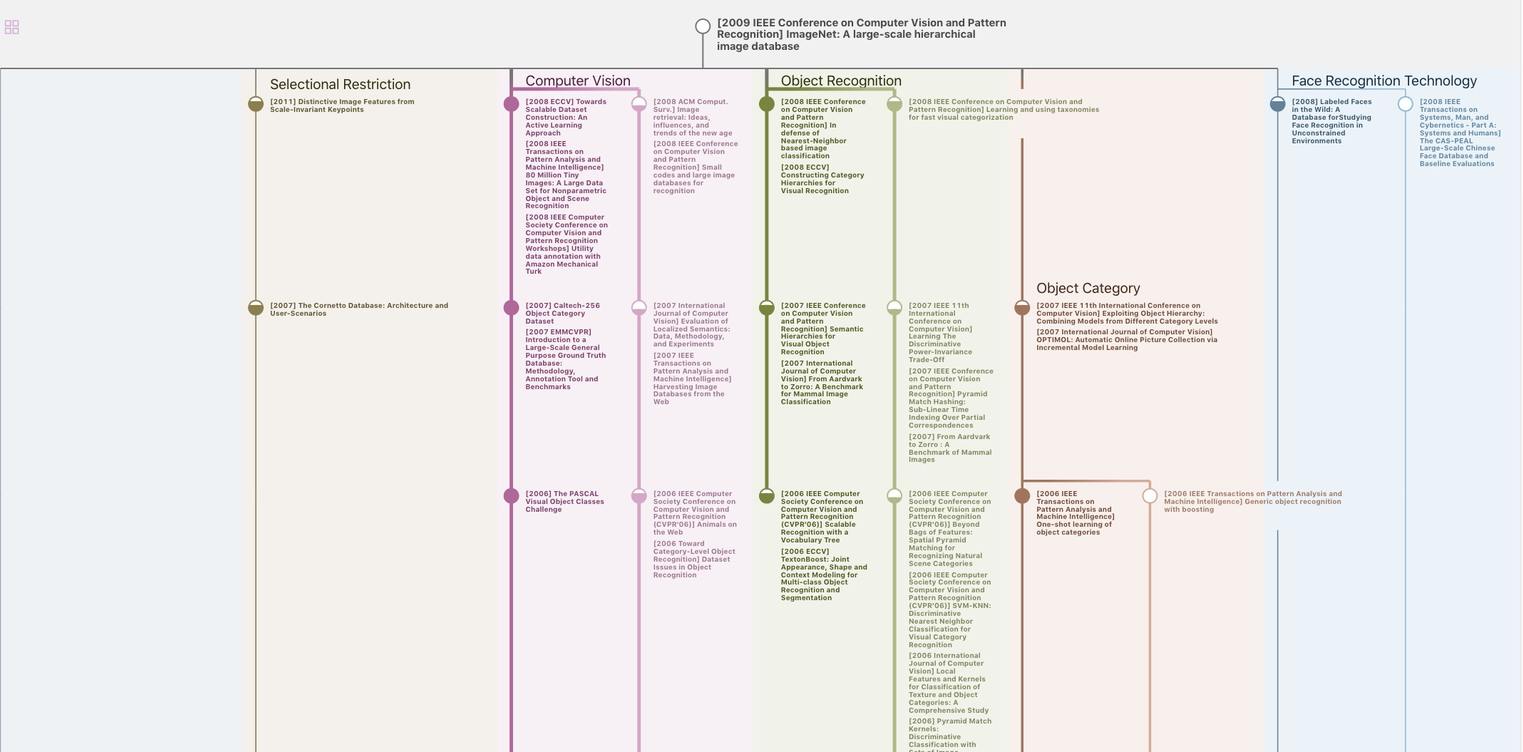
生成溯源树,研究论文发展脉络
Chat Paper
正在生成论文摘要