Semantic-Enhanced Knowledge Graph Completion
MATHEMATICS(2024)
摘要
Knowledge graphs (KGs) serve as structured representations of knowledge, comprising entities and relations. KGs are inherently incomplete, sparse, and have a strong need for completion. Although many knowledge graph embedding models have been designed for knowledge graph completion, they predominantly focus on capturing observable correlations between entities. Due to the sparsity of KGs, potential semantic correlations are challenging to capture. To tackle this problem, we propose a model entitled semantic-enhanced knowledge graph completion (SE-KGC). SE-KGC effectively addresses the issue by incorporating predefined semantic patterns, enabling the capture of semantic correlations between entities and enhancing features for representation learning. To implement this approach, we employ a multi-relational graph convolution network encoder, which effectively encodes the KG. Subsequently, we utilize a scoring decoder to evaluate triplets. Experimental results demonstrate that our SE-KGC model outperforms other state-of-the-art methods in link-prediction tasks across three datasets. Specifically, compared to the baselines, SE-KGC achieved improvements of 11.7%, 1.05%, and 2.30% in terms of MRR on these three datasets. Furthermore, we present a comprehensive analysis of the contributions of different semantic patterns, and find that entities with higher connectivity play a pivotal role in effectively capturing and characterizing semantic information.
更多查看译文
关键词
knowledge graph completion,relational graph convolutional network,higher-order semantic pattern
AI 理解论文
溯源树
样例
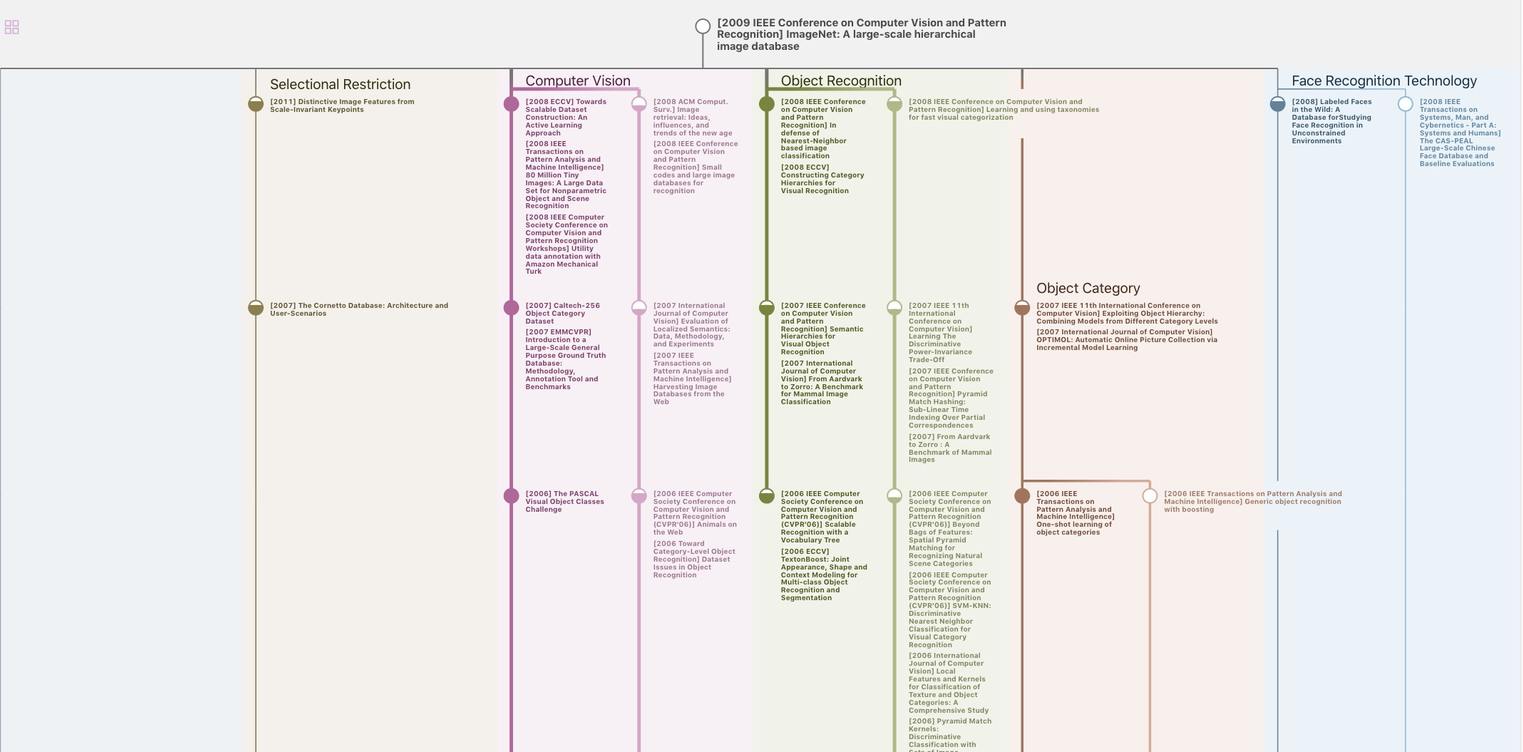
生成溯源树,研究论文发展脉络
Chat Paper
正在生成论文摘要