TendiffPure: a convolutional tensor-train denoising diffusion model for purification
Frontiers of Information Technology & Electronic Engineering(2024)
摘要
Diffusion models are effective purification methods, where the noises or adversarial attacks are removed using generative approaches before pre-existing classifiers conducting classification tasks. However, the efficiency of diffusion models is still a concern, and existing solutions are based on knowledge distillation which can jeopardize the generation quality because of the small number of generation steps. Hence, we propose TendiffPure as a tensorized and compressed diffusion model for purification. Unlike the knowledge distillation methods, we directly compress U-Nets as backbones of diffusion models using tensor-train decomposition, which reduces the number of parameters and captures more spatial information in multi-dimensional data such as images. The space complexity is reduced from O ( N 2 ) to O ( NR 2 ) with R ≤ 4 as the tensor-train rank and N as the number of channels. Experimental results show that TendiffPure can more efficiently obtain high-quality purification results and outperforms the baseline purification methods on CIFAR-10, Fashion-MNIST, and MNIST datasets for two noises and one adversarial attack.
更多查看译文
关键词
Diffusion models,Tensor decomposition,Image denoising,扩散模型,张量分解,图像去噪,TP391.4
AI 理解论文
溯源树
样例
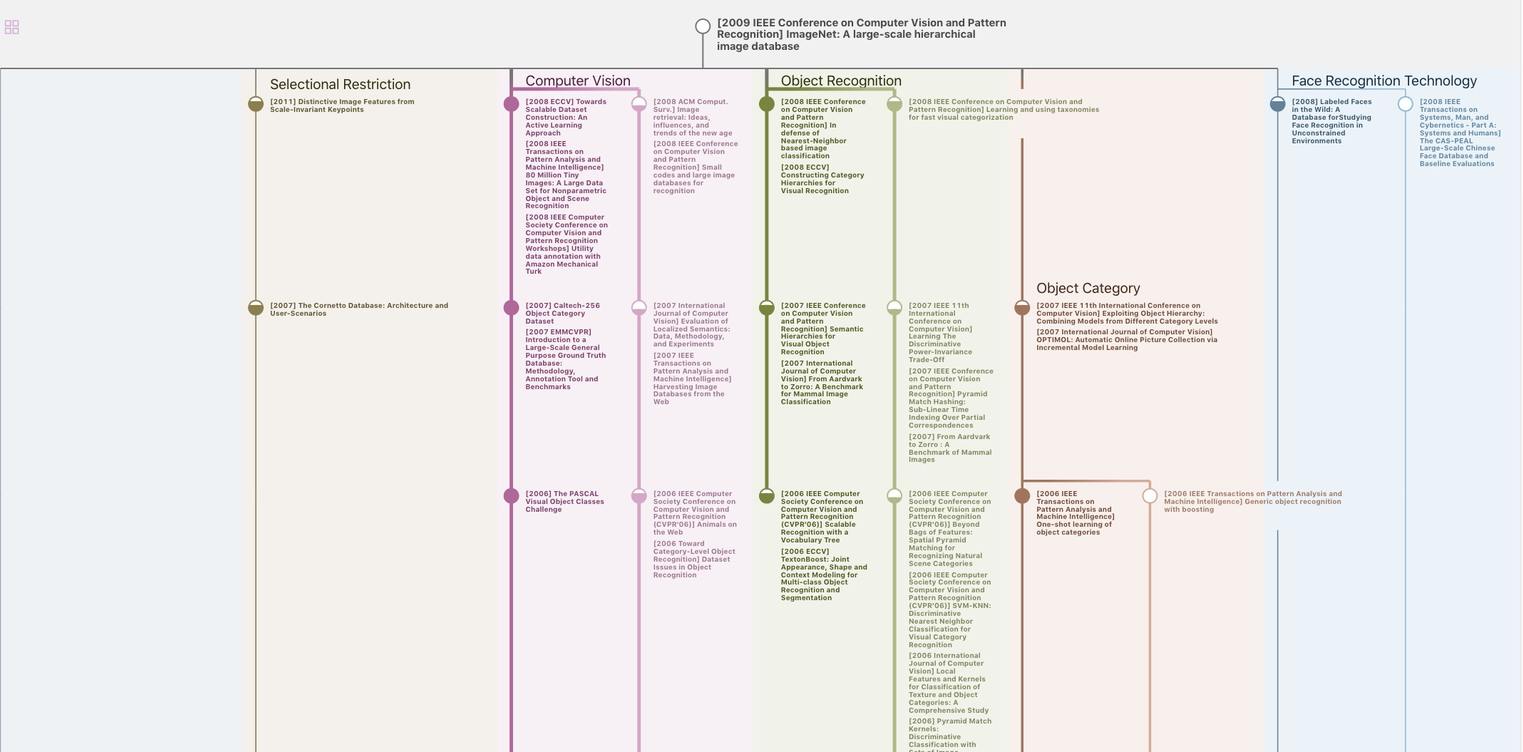
生成溯源树,研究论文发展脉络
Chat Paper
正在生成论文摘要