Deep Learning for Chest X-ray Diagnosis: Competition Between Radiologists with or Without Artificial Intelligence Assistance
Journal of Imaging Informatics in Medicine(2024)
摘要
This study aimed to assess the performance of a deep learning algorithm in helping radiologist achieve improved efficiency and accuracy in chest radiograph diagnosis. We adopted a deep learning algorithm to concurrently detect the presence of normal findings and 13 different abnormalities in chest radiographs and evaluated its performance in assisting radiologists. Each competing radiologist had to determine the presence or absence of these signs based on the label provided by the AI. The 100 radiographs were randomly divided into two sets for evaluation: one without AI assistance (control group) and one with AI assistance (test group). The accuracy, false-positive rate, false-negative rate, and analysis time of 111 radiologists (29 senior, 32 intermediate, and 50 junior) were evaluated. A radiologist was given an initial score of 14 points for each image read, with 1 point deducted for an incorrect answer and 0 points given for a correct answer. The final score for each doctor was automatically calculated by the backend calculator. We calculated the mean scores of each radiologist in the two groups (the control group and the test group) and calculated the mean scores to evaluate the performance of the radiologists with and without AI assistance. The average score of the 111 radiologists was 597 (587–605) in the control group and 619 (612–626) in the test group ( P < 0.001). The time spent by the 111 radiologists on the control and test groups was 3279 (2972–3941) and 1926 (1710–2432) s, respectively ( P < 0.001). The performance of the 111 radiologists in the two groups was evaluated by the area under the receiver operating characteristic curve (AUC). The radiologists showed better performance on the test group of radiographs in terms of normal findings, pulmonary fibrosis, heart shadow enlargement, mass, pleural effusion, and pulmonary consolidation recognition, with AUCs of 1.0, 0.950, 0.991, 1.0, 0.993, and 0.982, respectively. The radiologists alone showed better performance in aortic calcification (0.993), calcification (0.933), cavity (0.963), nodule (0.923), pleural thickening (0.957), and rib fracture (0.987) recognition. This competition verified the positive effects of deep learning methods in assisting radiologists in interpreting chest X-rays. AI assistance can help to improve both the efficacy and efficiency of radiologists.
更多查看译文
关键词
Chest X-ray,Artificial intelligence,Competition,Deep learning,Score
AI 理解论文
溯源树
样例
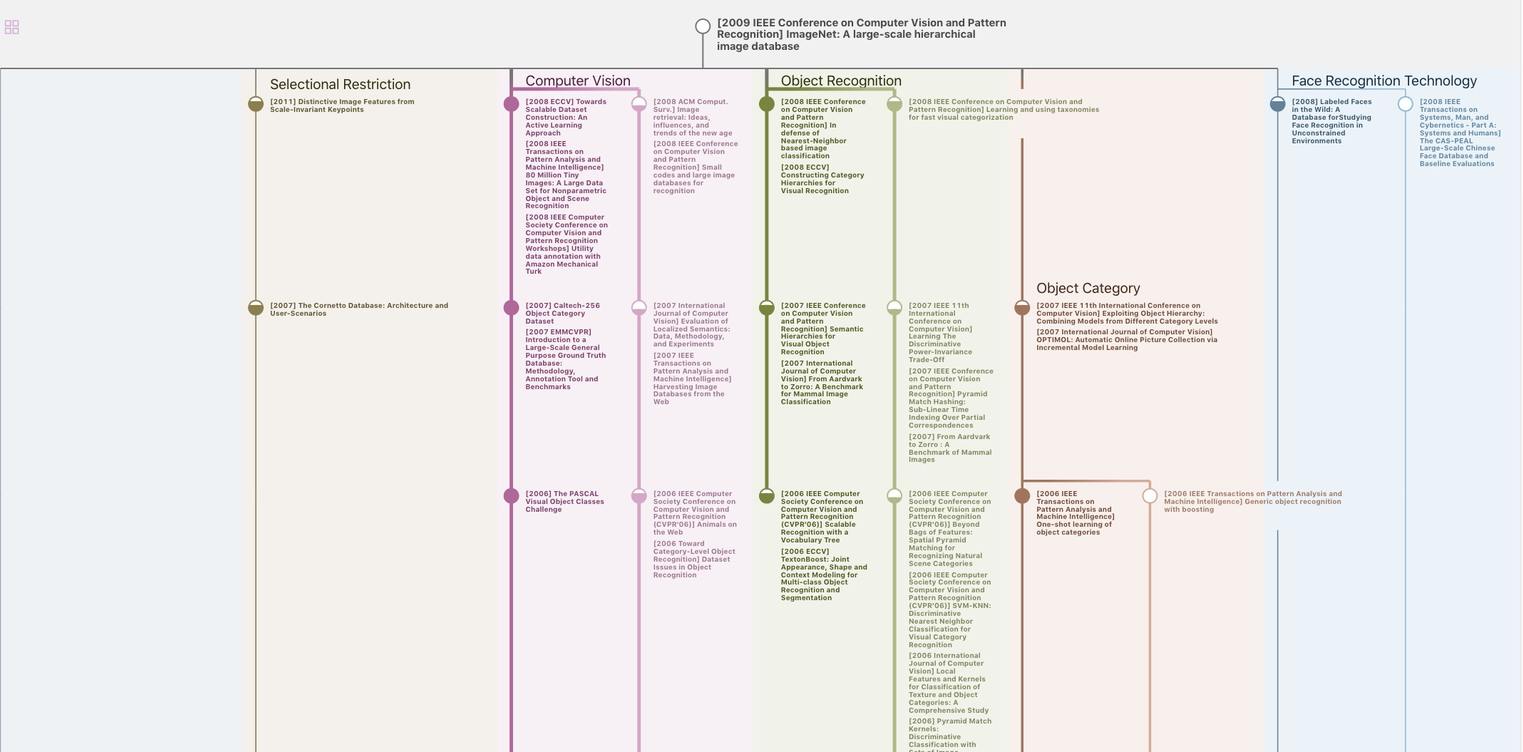
生成溯源树,研究论文发展脉络
Chat Paper
正在生成论文摘要