Hierarchical Transformer With Lightweight Attention for Radar-Based Precipitation Nowcasting
IEEE GEOSCIENCE AND REMOTE SENSING LETTERS(2024)
摘要
The U-net and Transformer have garnered significant attention in precipitation nowcasting due to their impressive capabilities in modeling sequential information. However, the performance is still constrained by the computational complexity of attention mechanism and the persistence of redundant information transmission between encoding and decoding stages. To address the above problems, we propose a novel hierarchical transformer with lightweight attention (HTLA) for precipitation nowcasting, which can integrate the Transformer and U-Net architectures to comprehensively explore the intrinsic characteristics of rainfall data with less complexity. Specifically, HTLA incorporates cross-channel self-attention with lightweight and dual feedforward module as fundamental components for encoding and decoding, efficiently fusing the advantages of Transformer and U-Net. A Gaussian pooling skip-connection strategy is proposed to adaptively weight information, effectively suppressing the redundant interference from the encoder to the decoder. The experimental results demonstrate the effectiveness and robustness of our HTLA, achieving improvements of 5.6% and 5.1% in terms of critical success index (CSI) and Heidke skill score (HSS) with only 3.6% parameters compared to the state-of-the-art method. The code is available at https://github.com/precipitation-zy/HTLA.
更多查看译文
关键词
Precipitation nowcasting,transformer,U-net
AI 理解论文
溯源树
样例
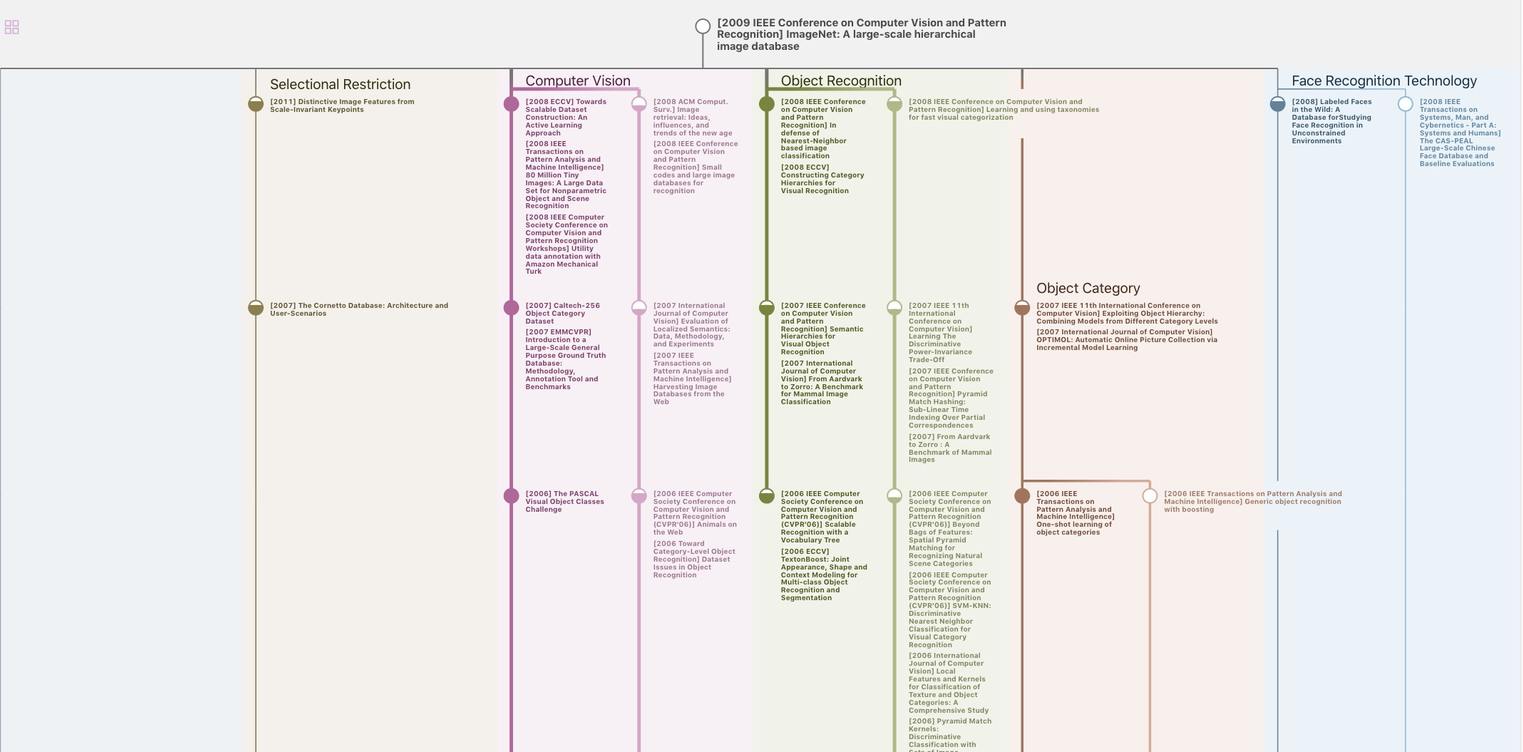
生成溯源树,研究论文发展脉络
Chat Paper
正在生成论文摘要