Local-Global Feature Fusion Network for Efficient Hyperspectral Image Super-Resolution
IEEE GEOSCIENCE AND REMOTE SENSING LETTERS(2024)
摘要
Numerous hyperspectral image (HSI) super-resolution (SR) approaches have been proposed and attained remarkable performance recently. However, the enormous computational and memory costs of the deeper and heavier networks make the application challenging in the actual scene. Therefore, a lightweight but efficient local-global feature fusion module network (LGFFMN) is proposed to reduce the computational burden while achieving superior reconstruction performance. In particular, a module local feature fusion module (LFFM) is proposed to use convolutional neural network (CNN) for effective local feature extraction. Meanwhile, to exploit global information and avoid the computational burden of traditional transformers, a multiscale representation-based feature modulation mechanism is adopted to build a vision transformer (ViT)-like block global feature fusion module (GFFM). To reduce the high dimension of HSI while using the higher similarity among adjacent bands, the overall processing manner is group by group. Experiments demonstrate that our LGFFMN achieves a satisfactory balance in SR reconstruction performance and efficiency metrics and is highly competitive compared with other state-of-the-art HSI SR methods.
更多查看译文
关键词
Convolutional neural network (CNN),hyperspectral image (HSI) super-resolution (SR),vision transformer (ViT)
AI 理解论文
溯源树
样例
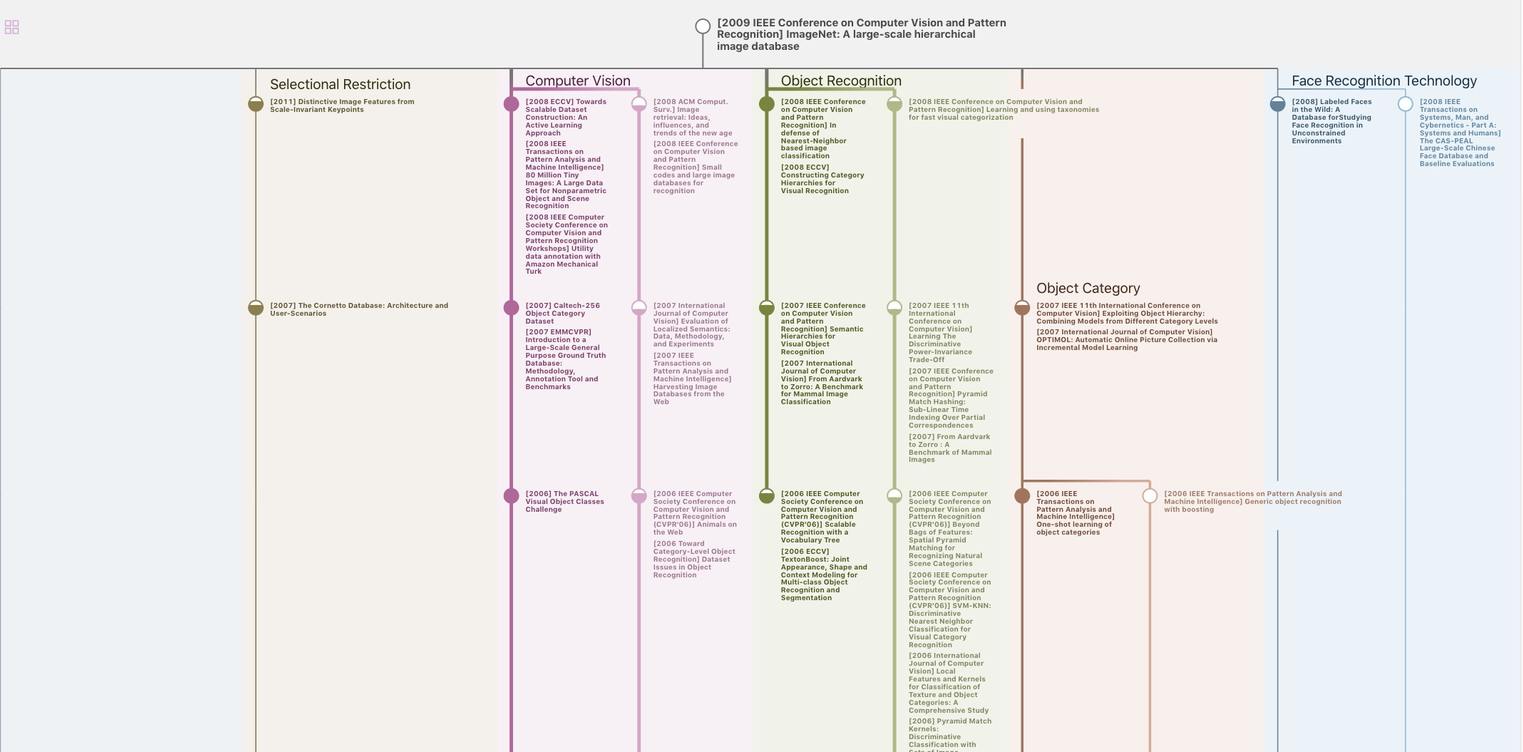
生成溯源树,研究论文发展脉络
Chat Paper
正在生成论文摘要