FAMEWS: a Fairness Auditing tool for Medical Early-Warning Systems
medrxiv(2024)
摘要
Machine learning applications hold promise to aid clinicians in a wide range of clinical tasks, from diagnosis to prognosis, treatment, and patient monitoring. These potential applications are accompanied by a surge of ethical concerns surrounding the use of Machine Learning (ML) models in healthcare, especially regarding fairness and non-discrimination. While there is an increasing number of regulatory policies to ensure the ethical and safe integration of such systems, the translation from policies to practices remains an open challenge. Algorithmic frameworks, aiming to bridge this gap, should be tailored to the application to enable the translation from fundamental human-right principles into accurate statistical analysis, capturing the inherent complexity and risks associated with the system. In this work, we propose a set of fairness impartial checks especially adapted to ML early-warning systems in the medical context, comprising on top of standard fairness metrics, an analysis of clinical outcomes, and a screening of potential sources of bias in the pipeline. Our analysis is further fortified by the inclusion of event-based and prevalence-corrected metrics, as well as statistical tests to measure biases. Additionally, we emphasize the importance of considering subgroups beyond the conventional demographic attributes. Finally, to facilitate operationalization, we present an open-source tool FAMEWS to generate comprehensive fairness reports. These reports address the diverse needs and interests of the stakeholders involved in integrating ML into medical practice. The use of FAMEWS has the potential to reveal critical insights that might otherwise remain obscured. This can lead to improved model design, which in turn may translate into enhanced health outcomes.
### Competing Interest Statement
The authors have declared no competing interest.
### Funding Statement
This project was supported by grant \#2022-278 of the Strategic Focus Area Personalized Health and Related Technologies (PHRT) of the ETH Domain (Swiss Federal Institutes of Technology), and ETH core funding (to GR). OM is also supported by the Max Planck ETH Center for Learning Systems. We acknowledge discussions with David Berger, Martin Faltys and Effy Vayena. Computational analyses were performed at the LeonhardMed Trusted Research Environment at ETH Zurich ( ).
### Author Declarations
I confirm all relevant ethical guidelines have been followed, and any necessary IRB and/or ethics committee approvals have been obtained.
Yes
The details of the IRB/oversight body that provided approval or exemption for the research described are given below:
The institutional review board (IRB) of the Canton of Bern approved the study on retrospective ICU (BASEC 2016 01463). The need for obtaining informed patient consent for patient data from our institution was waived owing to the retrospective and observational nature of the study.
I confirm that all necessary patient/participant consent has been obtained and the appropriate institutional forms have been archived, and that any patient/participant/sample identifiers included were not known to anyone (e.g., hospital staff, patients or participants themselves) outside the research group so cannot be used to identify individuals.
Yes
I understand that all clinical trials and any other prospective interventional studies must be registered with an ICMJE-approved registry, such as ClinicalTrials.gov. I confirm that any such study reported in the manuscript has been registered and the trial registration ID is provided (note: if posting a prospective study registered retrospectively, please provide a statement in the trial ID field explaining why the study was not registered in advance).
Yes
I have followed all appropriate research reporting guidelines, such as any relevant EQUATOR Network research reporting checklist(s) and other pertinent material, if applicable.
Yes
In this study, we primarily experiment with HIRID dataset, which is available for download on PhysioNet: , and with the benchmark models for early-detection of organ failure whose code base is available at . The FAMEWS open-source tool is available at: .
更多查看译文
AI 理解论文
溯源树
样例
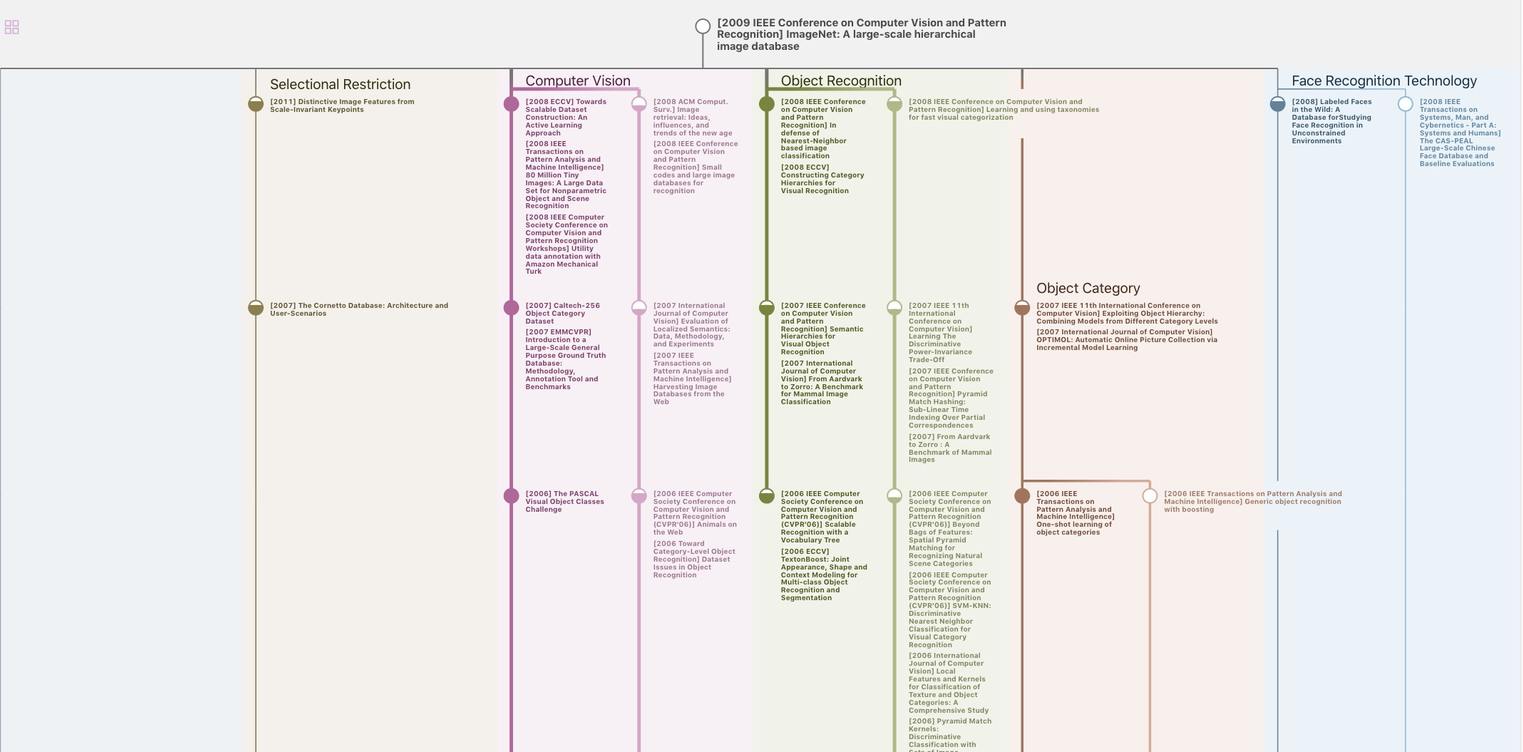
生成溯源树,研究论文发展脉络
Chat Paper
正在生成论文摘要