Memory Consolidation Enables Long-Context Video Understanding
ICML 2024(2024)
Abstract
Most transformer-based video encoders are limited to short temporal contexts due to their quadratic complexity. While various attempts have been made to extend this context, this has often come at the cost of both conceptual and computational complexity. We propose to instead re-purpose existing pre-trained video transformers by simply fine-tuning them to attend to memories derived non-parametrically from past activations. By leveraging redundancy reduction, our memory-consolidated vision transformer (MC-ViT) effortlessly extends its context far into the past and exhibits excellent scaling behavior when learning from longer videos. In doing so, MC-ViT sets a new state-of-the-art in long-context video understanding on EgoSchema, Perception Test, and Diving48, outperforming methods that benefit from orders of magnitude more parameters.
MoreTranslated text
AI Read Science
Must-Reading Tree
Example
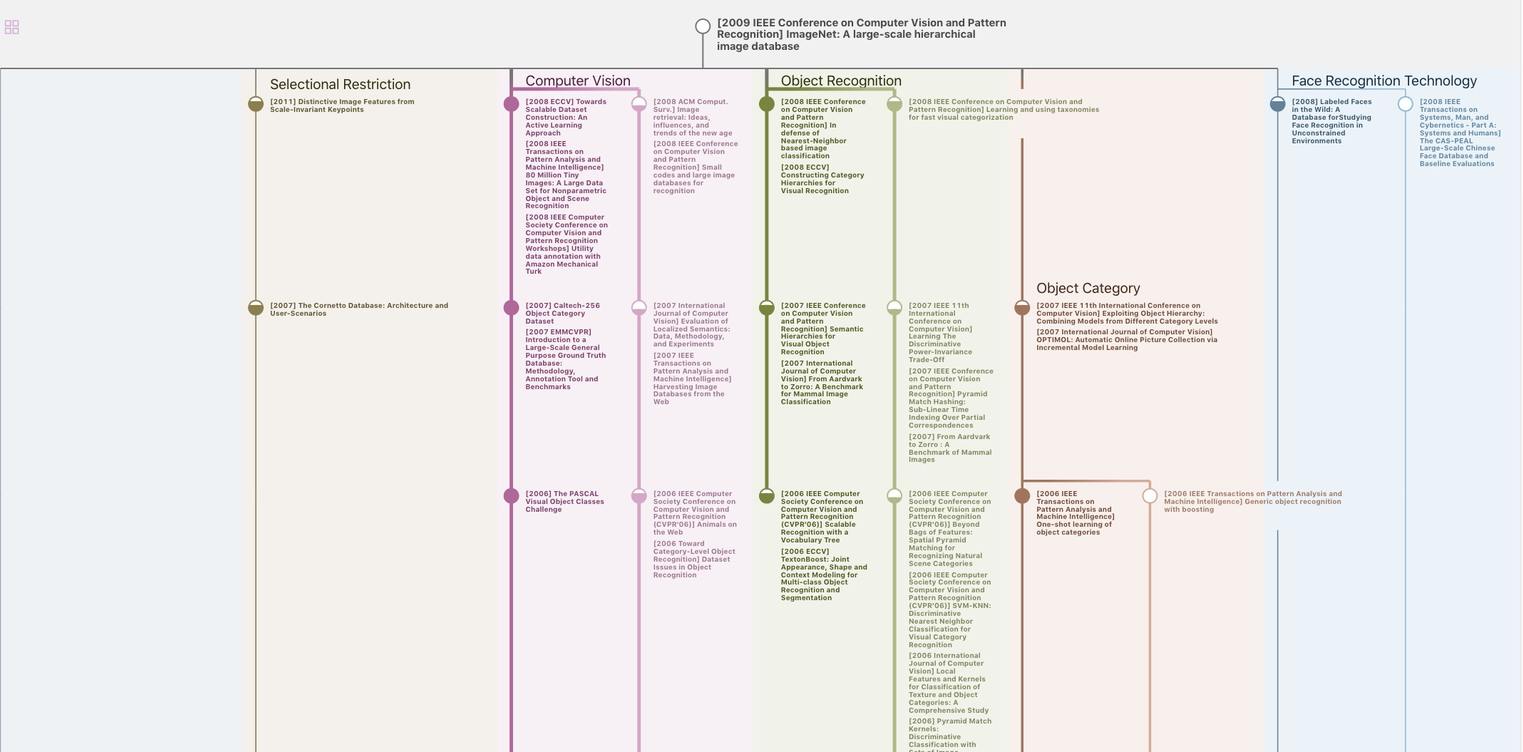
Generate MRT to find the research sequence of this paper
Chat Paper
Summary is being generated by the instructions you defined