Limits of Transformer Language Models on Learning Algorithmic Compositions
CoRR(2024)
摘要
We analyze the capabilities of Transformer language models on learning
discrete algorithms. To this end, we introduce two new tasks demanding the
composition of several discrete sub-tasks. On both training LLaMA models from
scratch and prompting on GPT-4 and Gemini we measure learning compositions of
learned primitives. We observe that the compositional capabilities of
state-of-the-art Transformer language models are very limited and sample-wise
scale worse than relearning all sub-tasks for a new algorithmic composition. We
also present a theorem in complexity theory, showing that gradient descent on
memorizing feedforward models can be exponentially data inefficient.
更多查看译文
AI 理解论文
溯源树
样例
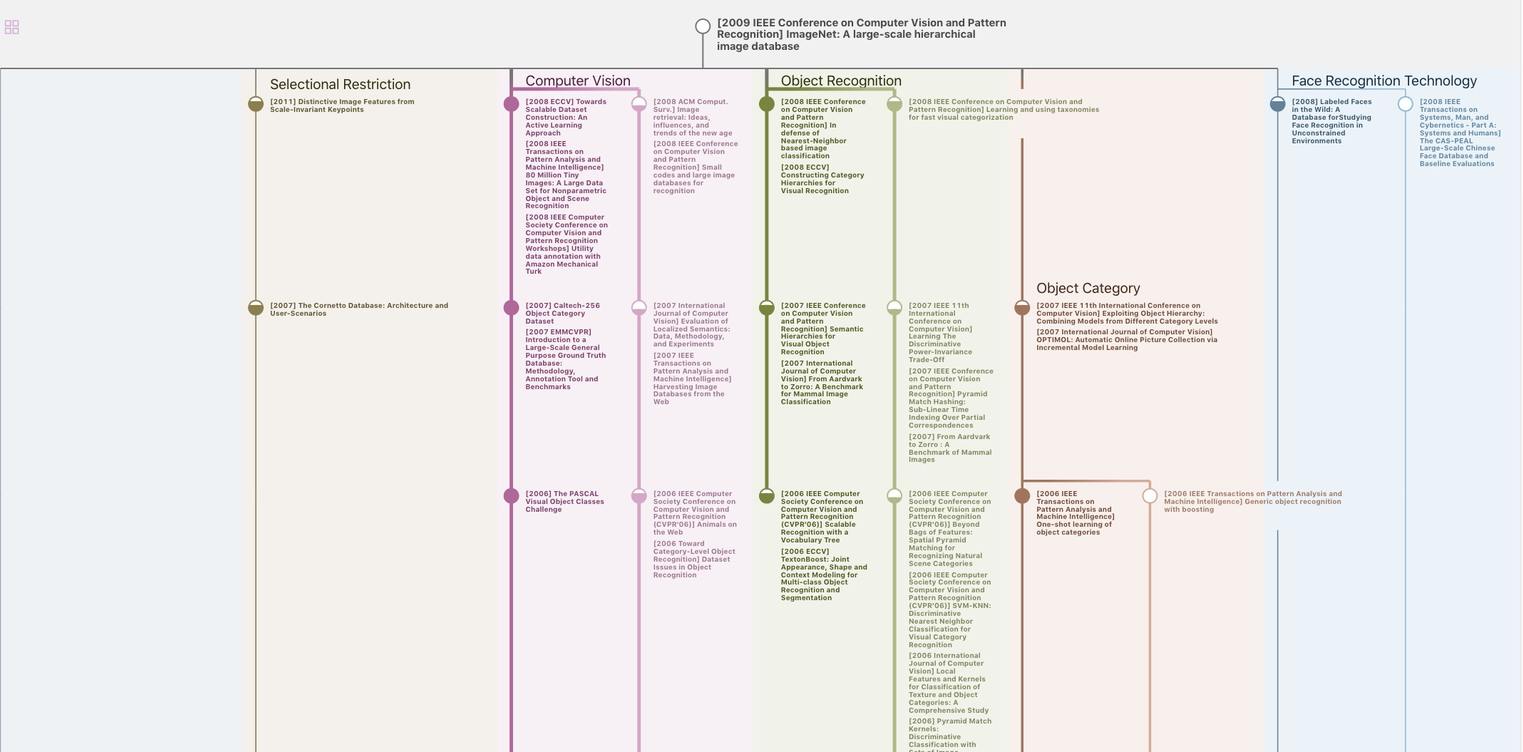
生成溯源树,研究论文发展脉络
Chat Paper
正在生成论文摘要