Offline Actor-Critic Reinforcement Learning Scales to Large Models
ICML 2024(2024)
Key words
Psychometric Models
AI Read Science
Must-Reading Tree
Example
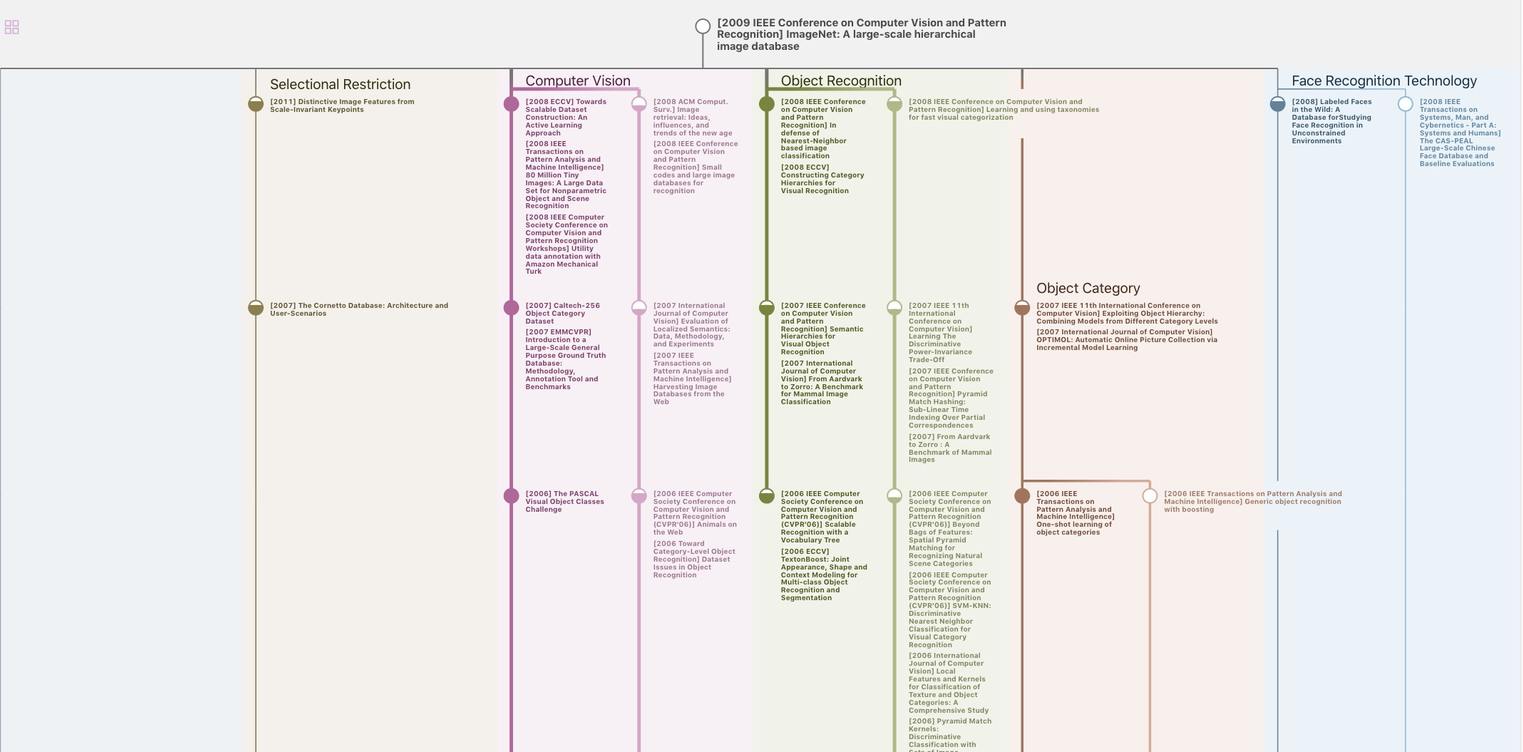
Generate MRT to find the research sequence of this paper
Chat Paper
Summary is being generated by the instructions you defined