Optimizing for ROC Curves on Class-Imbalanced Data by Training over a Family of Loss Functions
CoRR(2024)
摘要
Although binary classification is a well-studied problem in computer vision,
training reliable classifiers under severe class imbalance remains a
challenging problem. Recent work has proposed techniques that mitigate the
effects of training under imbalance by modifying the loss functions or
optimization methods. While this work has led to significant improvements in
the overall accuracy in the multi-class case, we observe that slight changes in
hyperparameter values of these methods can result in highly variable
performance in terms of Receiver Operating Characteristic (ROC) curves on
binary problems with severe imbalance. To reduce the sensitivity to
hyperparameter choices and train more general models, we propose training over
a family of loss functions, instead of a single loss function. We develop a
method for applying Loss Conditional Training (LCT) to an imbalanced
classification problem. Extensive experiment results, on both CIFAR and Kaggle
competition datasets, show that our method improves model performance and is
more robust to hyperparameter choices. Code will be made available at:
https://github.com/klieberman/roc_lct.
更多查看译文
AI 理解论文
溯源树
样例
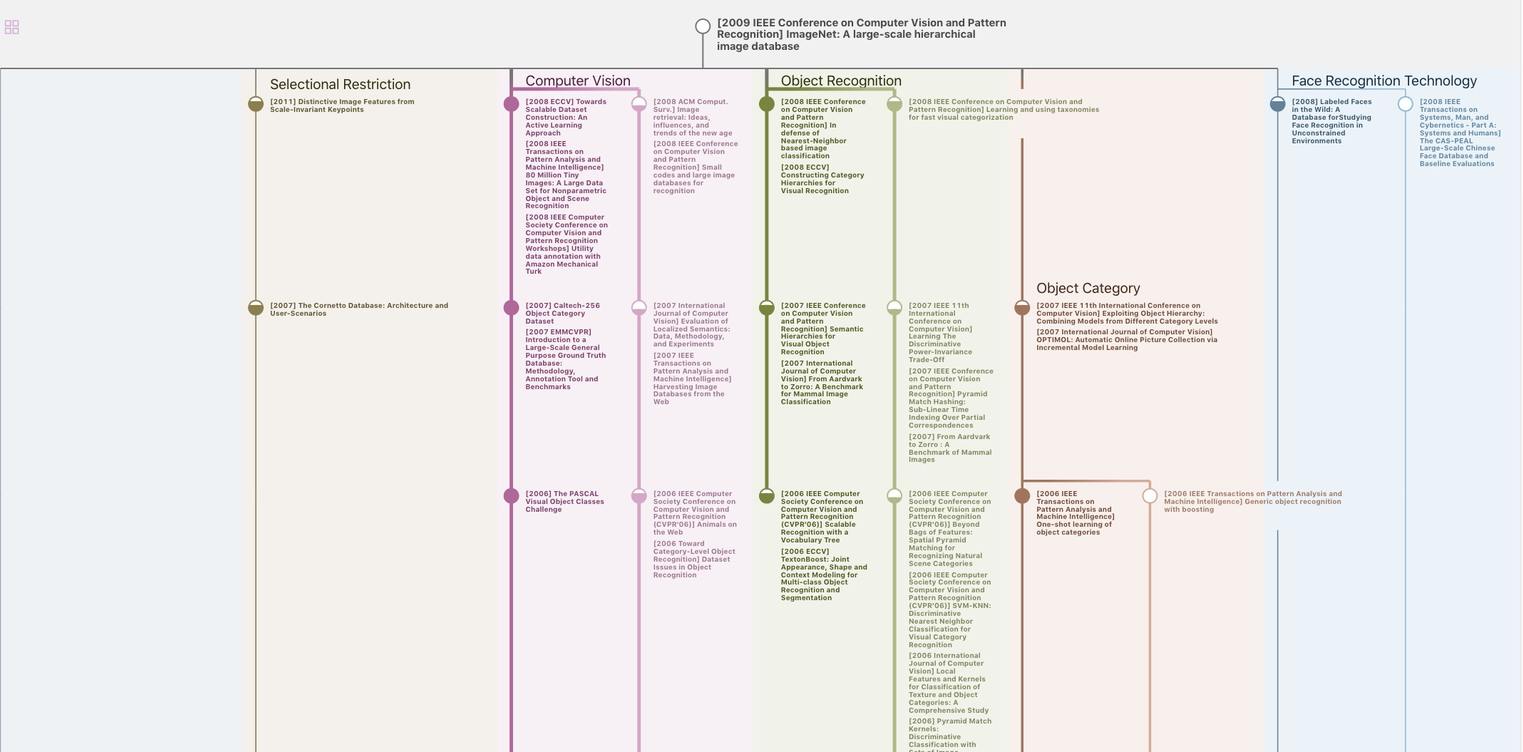
生成溯源树,研究论文发展脉络
Chat Paper
正在生成论文摘要