Validation workflow for machine learning interatomic potentials for complex ceramics
COMPUTATIONAL MATERIALS SCIENCE(2024)
Abstract
The number of published Machine Learning Interatomic Potentials (MLIPs) has increased significantly in recent years. These new data-driven potential energy approximations often lack the physics-based foundations that inform many traditionally-developed interatomic potentials and hence require robust validation methods for their accuracy, computational efficiency, and applicability to the intended applications. This work presents a sequential, three-stage workflow for MLIP validation: (i) preliminary validation, (ii) static property prediction, and (iii) dynamic property prediction. This material-agnostic procedure is demonstrated in a tutorial approach for the development of a robust MLIP for boron carbide (B4C), a widely employed, structurally complex ceramic that undergoes a deleterious deformation mechanism called 'amorphization' under high-pressure loading. It is shown that the resulting B4C MLIP offers a more accurate prediction of properties compared to the available empirical potential.
MoreTranslated text
Key words
Boron carbide,Neural network,Molecular Dynamics,Extreme environments,Shock,Advanced ceramics,Structural ceramics,LAMMPS,DeePMD-kit,Tutorial
AI Read Science
Must-Reading Tree
Example
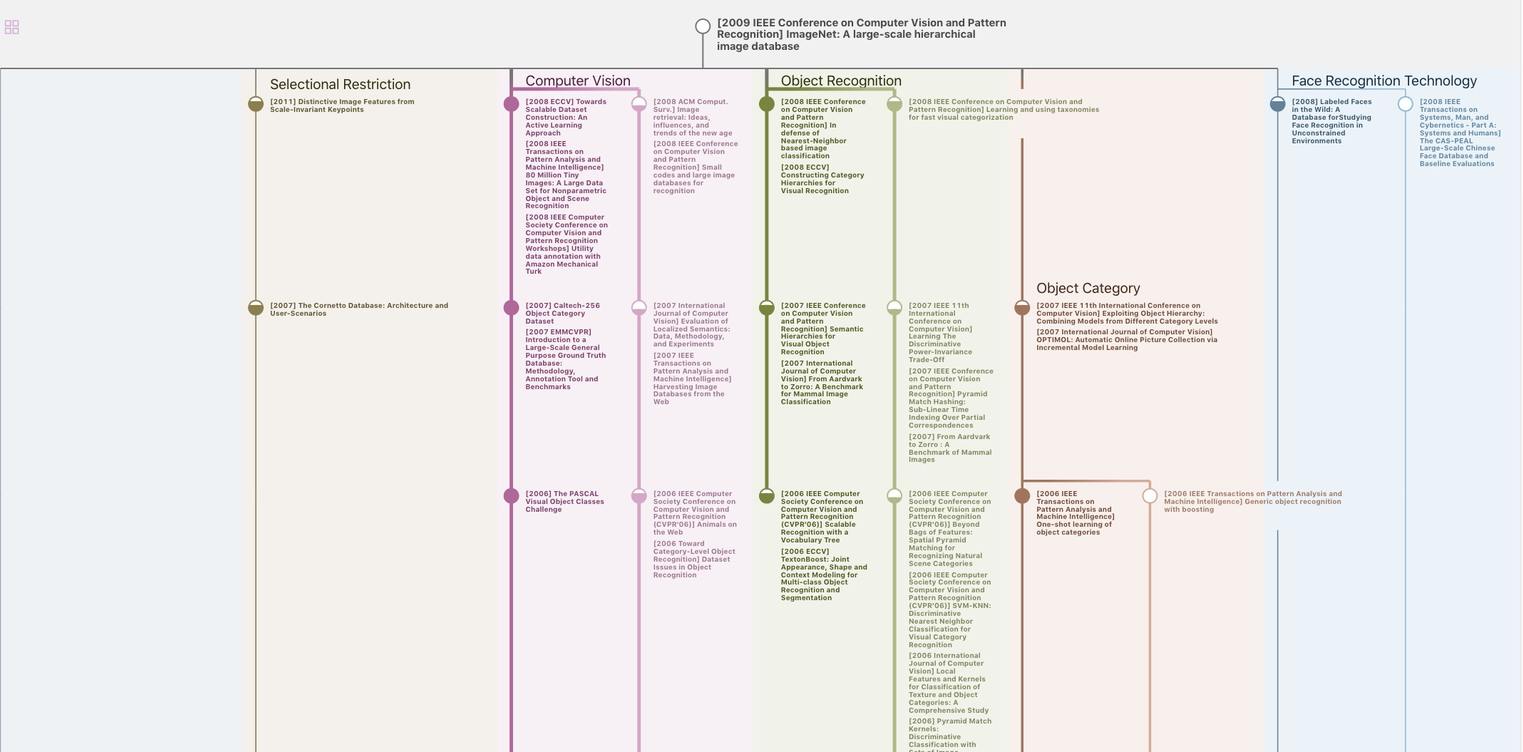
Generate MRT to find the research sequence of this paper
Chat Paper
Summary is being generated by the instructions you defined