EPDet: Enhancing point clouds features with effective representation for 3D object detection
INTERNATIONAL JOURNAL OF APPLIED EARTH OBSERVATION AND GEOINFORMATION(2024)
摘要
Effective 3D object detection relies on strong feature representation. Both global features and representative characteristics of the objects are vital for detection. However, traditional convolution's perception range limits large receptive fields, and current object feature representation has room for enhancement. Addressing these concerns, we introduce a 3D outdoor object detector to enhance the point clouds feature, referred to as EPDet. Specifically, for global features, we propose a BEV-Offset Transformer in the BEV (Bird's Eye View) domain. This adaptable module enhances semantic connections among objects, suiting various 3D detection methods. In addition, to refine point cloud features, we employ Focal Conv as our 3D ( 3-dimensional) backbone, exploring multi -modal fusion effects. In the 2D (2-dimensional) backbone, our Pyramid-like Conv captures detailed contextual features. EPDet performs well in the dense object scene owing to scene-wide global features captured by BEV Offset Transformer. In the multi-class tests, EPDet excels in detecting smaller objects due to more refined features represented by Focal Conv and Pyramid-like Conv. In experiments, as a plug-andplay module, we validate the BEV Offset Transformer's effectiveness across single -stage (SECOND), two-stage (Voxel-RCNN), and multi -stage (CasA) algorithms. Robustness is tested on KITTI, NuScenes, and ONCE datasets. The proposed EPDet, in the KITTI subset, EPDet (CasA-based) achieves an impressive 85.56% accuracy in the car category. EPDet (Voxel-RCNN-based) surpassed baseline 1.65% mAP (mean Average Precision) (moderate subsets) in multi-class detection. The precision of EPDet is on par with the SotA (State of the Art) 3D outdoor object detectors based on point clouds.
更多查看译文
关键词
Point clouds,Feature representation,BEV offset transformer,Focal Conv,Pyramid-like Conv
AI 理解论文
溯源树
样例
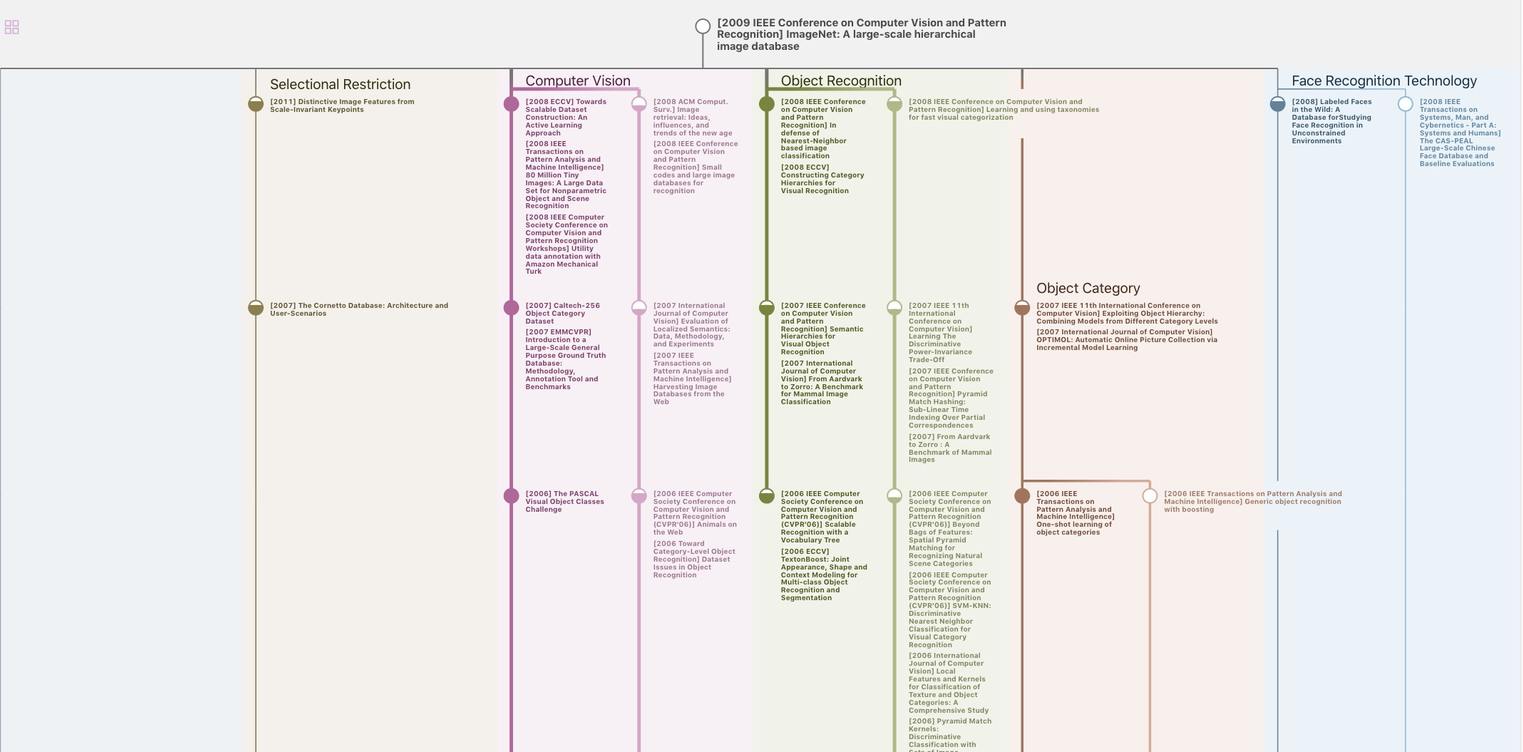
生成溯源树,研究论文发展脉络
Chat Paper
正在生成论文摘要