Vision-Language Model for Generating Textual Descriptions From Clinical Images: Model Development and Validation Study
JMIR FORMATIVE RESEARCH(2024)
摘要
Background: The automatic generation of radiology reports, which seeks to create a free -text description from a clinical radiograph, is emerging as a pivotal intersection between clinical medicine and artificial intelligence. Leveraging natural language processing technologies can accelerate report creation, enhancing health care quality and standardization. However, most existing studies have not yet fully tapped into the combined potential of advanced language and vision models. Objective: The purpose of this study was to explore the integration of pretrained vision -language models into radiology report generation. This would enable the vision -language model to automatically convert clinical images into high -quality textual reports. Methods: In our research, we introduced a radiology report generation model named ClinicalBLIP, building upon the foundational InstructBLIP model and refining it using clinical image -to -text data sets. A multistage fine-tuning approach via low -rank adaptation was proposed to deepen the semantic comprehension of the visual encoder and the large language model for clinical imagery. Furthermore, prior knowledge was integrated through prompt learning to enhance the precision of the reports generated. Experiments were conducted on both the IU X-RAY and MIMIC-CXR data sets, with ClinicalBLIP compared to several leading methods. Results: Experimental results revealed that ClinicalBLIP obtained superior scores of 0.570/0.365 and 0.534/0.313 on the IU X-RAY/MIMIC-CXR test sets for the Metric for Evaluation of Translation with Explicit Ordering (METEOR) and the Recall -Oriented Understudy for Gisting Evaluation (ROUGE) evaluations, respectively. This performance notably surpasses that of existing state-of-the-art methods. Further evaluations confirmed the effectiveness of the multistage fine-tuning and the integration of prior information, leading to substantial improvements. Conclusions: The proposed ClinicalBLIP model demonstrated robustness and effectiveness in enhancing clinical radiology report generation, suggesting significant promise for real -world clinical applications.
更多查看译文
关键词
clinical image,radiology report generation,vision-language model,multistage fine-tuning,prior knowledge
AI 理解论文
溯源树
样例
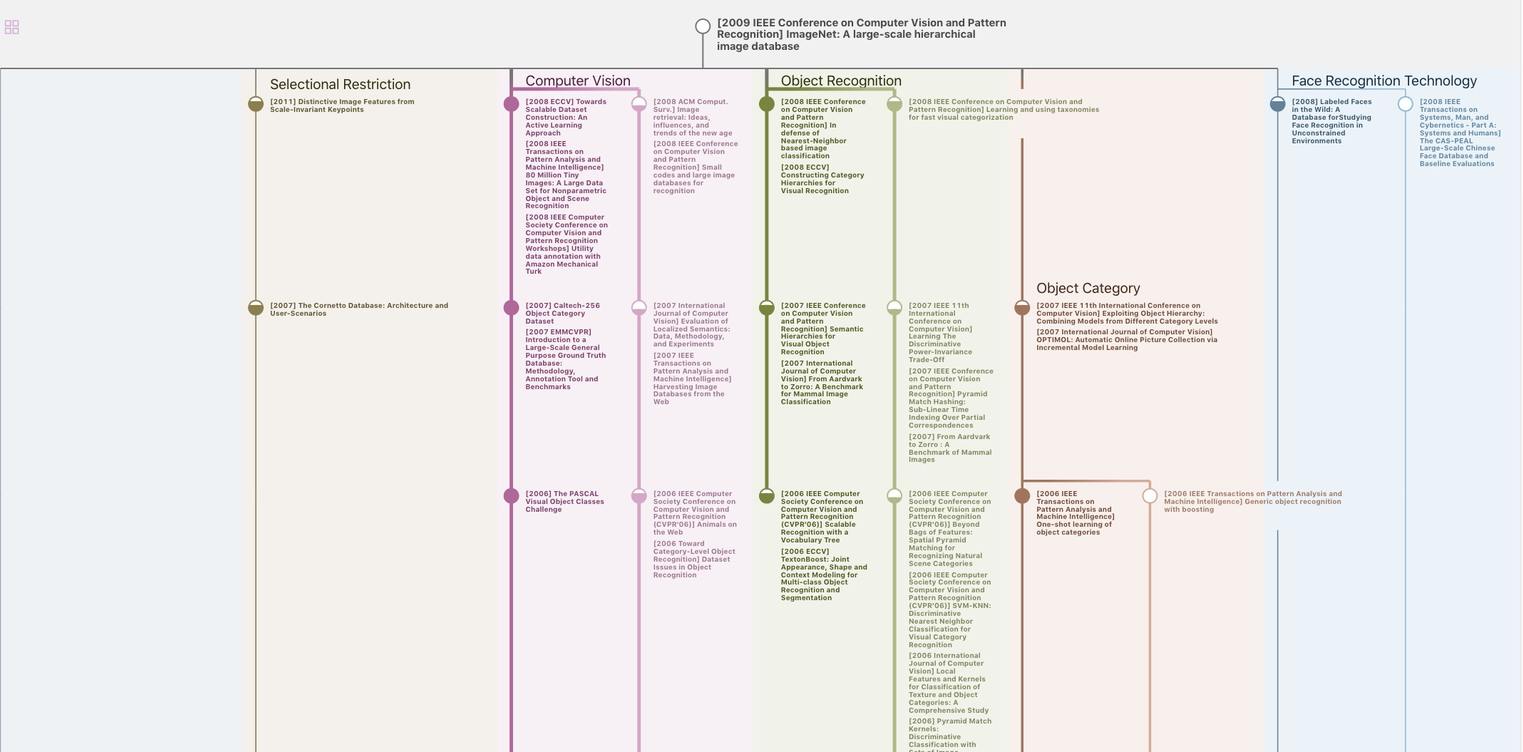
生成溯源树,研究论文发展脉络
Chat Paper
正在生成论文摘要