Efficient Invariant Kalman Filter for Inertial-based Odometry with Large-sample Environmental Measurements
CoRR(2024)
摘要
A filter for inertial-based odometry is a recursive method used to estimate
the pose from measurements of ego-motion and relative pose. Currently, there is
no known filter that guarantees the computation of a globally optimal solution
for the non-linear measurement model. In this paper, we demonstrate that an
innovative filter, with the state being SE_2(3) and the
√(n)-consistent pose as the initialization, efficiently achieves
asymptotic optimality in terms of minimum mean square error. This
approach is tailored for real-time SLAM and inertial-based odometry
applications.
Our first contribution is that we propose an iterative filtering method based
on the Gauss-Newton method on Lie groups which is numerically to solve the
estimation of states from a priori and non-linear measurements. The filtering
stands out due to its iterative mechanism and adaptive initialization. Second,
when dealing with environmental measurements of the surroundings, we utilize a
√(n)-consistent pose as the initial value for the update step in a single
iteration. The solution is closed in form and has computational complexity
O(n). Third, we theoretically show that the approach can achieve asymptotic
optimality in the sense of minimum mean square error from the a priori and
virtual relative pose measurements (see Problem ).
Finally, to validate our method, we carry out extensive numerical and
experimental evaluations. Our results consistently demonstrate that our
approach outperforms other state-of-the-art filter-based methods, including the
iterated extended Kalman filter and the invariant extended Kalman filter, in
terms of accuracy and running time.
更多查看译文
AI 理解论文
溯源树
样例
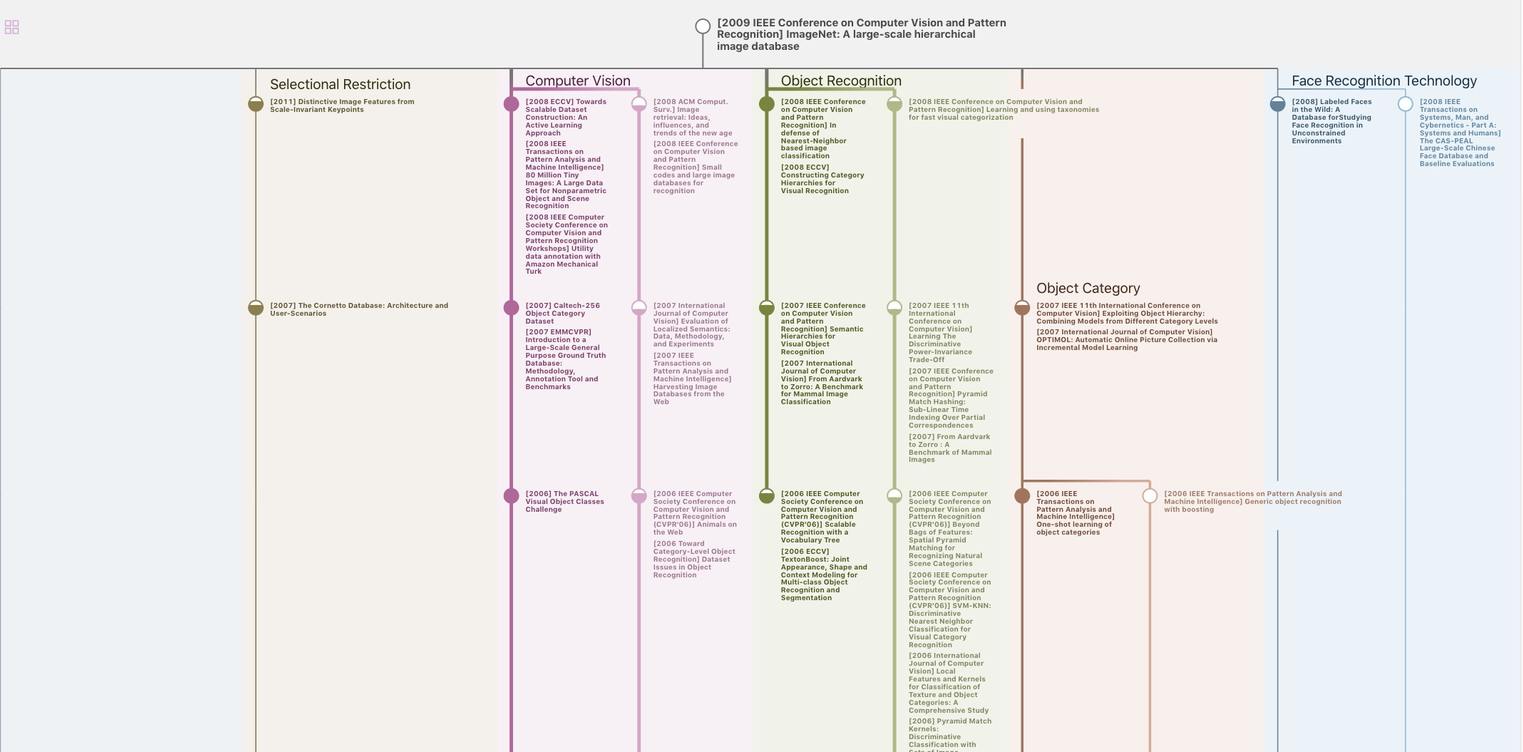
生成溯源树,研究论文发展脉络
Chat Paper
正在生成论文摘要