NORMY: Non-Uniform History Modeling for Open Retrieval Conversational Question Answering
CoRR(2024)
摘要
Open Retrieval Conversational Question Answering (OrConvQA) answers a
question given a conversation as context and a document collection. A typical
OrConvQA pipeline consists of three modules: a Retriever to retrieve relevant
documents from the collection, a Reranker to rerank them given the question and
the context, and a Reader to extract an answer span. The conversational turns
can provide valuable context to answer the final query. State-of-the-art
OrConvQA systems use the same history modeling for all three modules of the
pipeline. We hypothesize this as suboptimal. Specifically, we argue that a
broader context is needed in the first modules of the pipeline to not miss
relevant documents, while a narrower context is needed in the last modules to
identify the exact answer span. We propose NORMY, the first unsupervised
non-uniform history modeling pipeline which generates the best conversational
history for each module. We further propose a novel Retriever for NORMY, which
employs keyphrase extraction on the conversation history, and leverages
passages retrieved in previous turns as additional context. We also created a
new dataset for OrConvQA, by expanding the doc2dial dataset. We implemented
various state-of-the-art history modeling techniques and comprehensively
evaluated them separately for each module of the pipeline on three datasets:
OR-QUAC, our doc2dial extension, and ConvMix. Our extensive experiments show
that NORMY outperforms the state-of-the-art in the individual modules and in
the end-to-end system.
更多查看译文
关键词
question answering,history modeling,conversational,retriever,reranker,reader
AI 理解论文
溯源树
样例
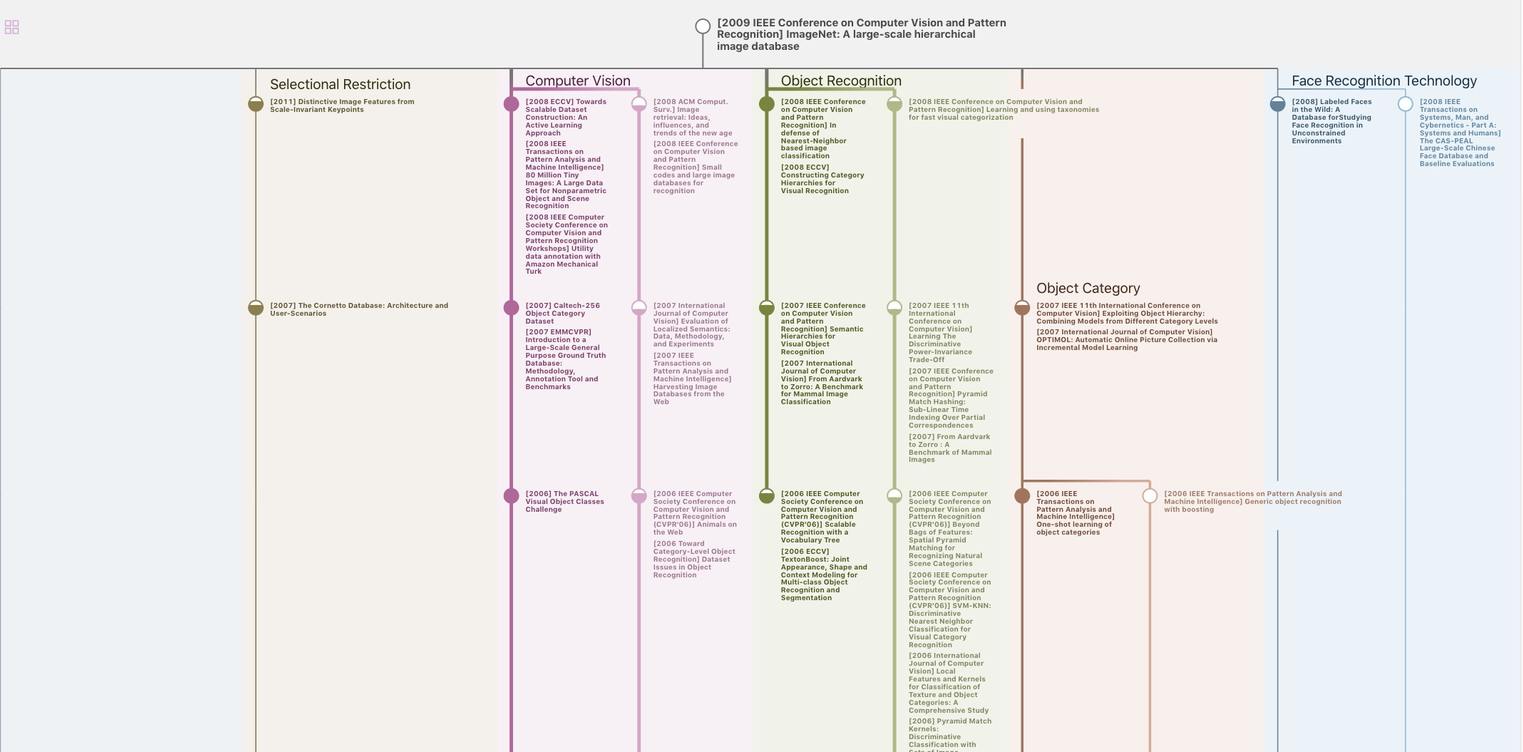
生成溯源树,研究论文发展脉络
Chat Paper
正在生成论文摘要