Scaling laws for learning with real and surrogate data
CoRR(2024)
摘要
Collecting large quantities of high-quality data is often prohibitively
expensive or impractical, and a crucial bottleneck in machine learning. One may
instead augment a small set of n data points from the target distribution
with data from more accessible sources like public datasets, data collected
under different circumstances, or synthesized by generative models. Blurring
distinctions, we refer to such data as `surrogate data'.
We define a simple scheme for integrating surrogate data into training and
use both theoretical models and empirical studies to explore its behavior. Our
main findings are: (i) Integrating surrogate data can significantly reduce
the test error on the original distribution; (ii) In order to reap this
benefit, it is crucial to use optimally weighted empirical risk minimization;
(iii) The test error of models trained on mixtures of real and surrogate data
is well described by a scaling law. This can be used to predict the optimal
weighting and the gain from surrogate data.
更多查看译文
AI 理解论文
溯源树
样例
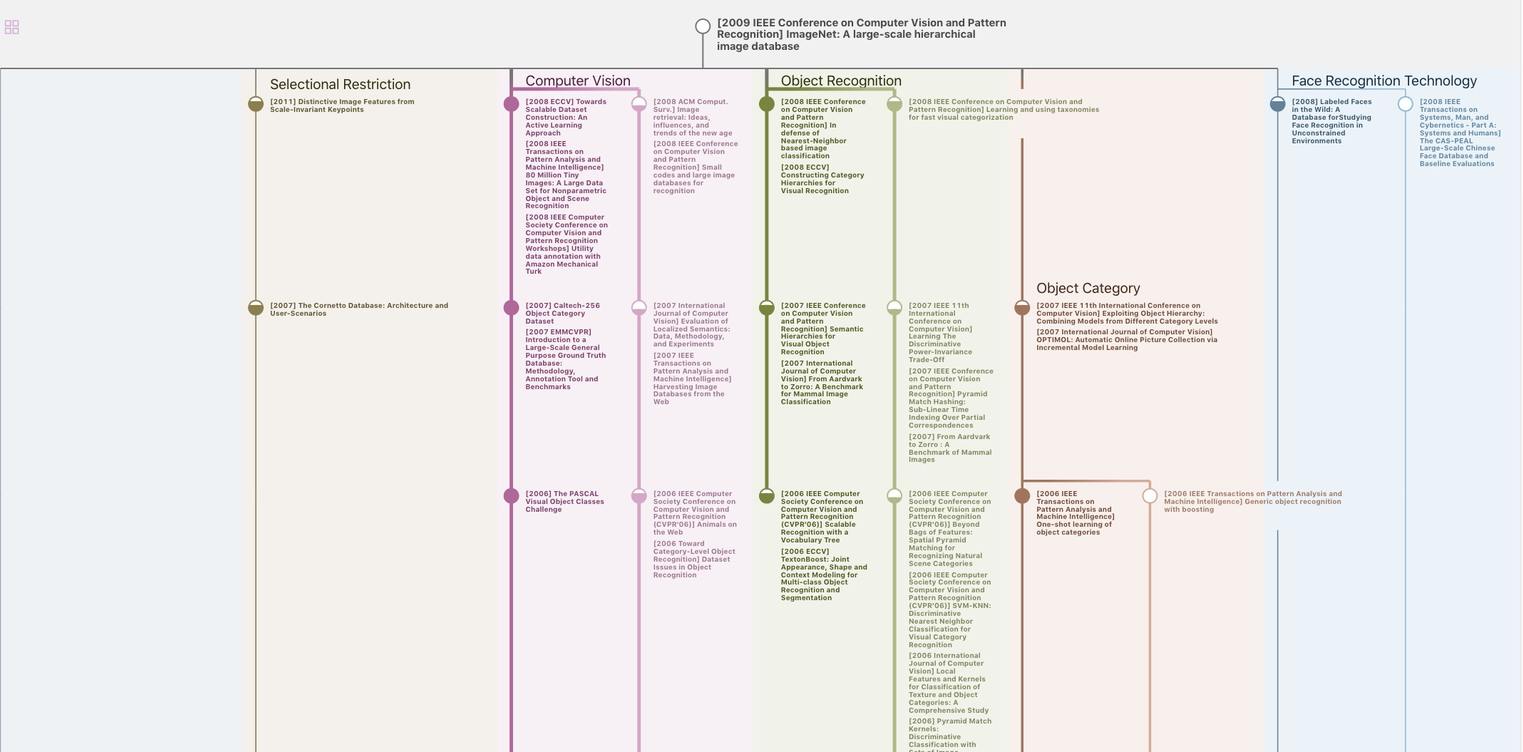
生成溯源树,研究论文发展脉络
Chat Paper
正在生成论文摘要