On the minimax robustness against correlation and heteroscedasticity of ordinary least squares among generalized least squares estimators of regression
arxiv(2024)
摘要
We present a result according to which certain functions of covariance
matrices are maximized at scalar multiples of the identity matrix. This is used
to show that the ordinary least squares (OLS) estimate of regression is
minimax, in the class of generalized least squares estimates, when the maximum
is taken over certain classes of error covariance structures and the loss
function possesses a natural monotonicity property. We then consider regression
models in which the response function is possibly misspecified, and show that
OLS is no longer minimax. We argue that the gains from a minimax estimate are
however often outweighed by the simplicity of OLS. We also investigate the
interplay between minimax precision matrices and minimax designs. We find that
the design has by far the major influence on efficiency and that, when the two
are combined, OLS is generally at least 'almost' minimax, and often exactly so.
更多查看译文
AI 理解论文
溯源树
样例
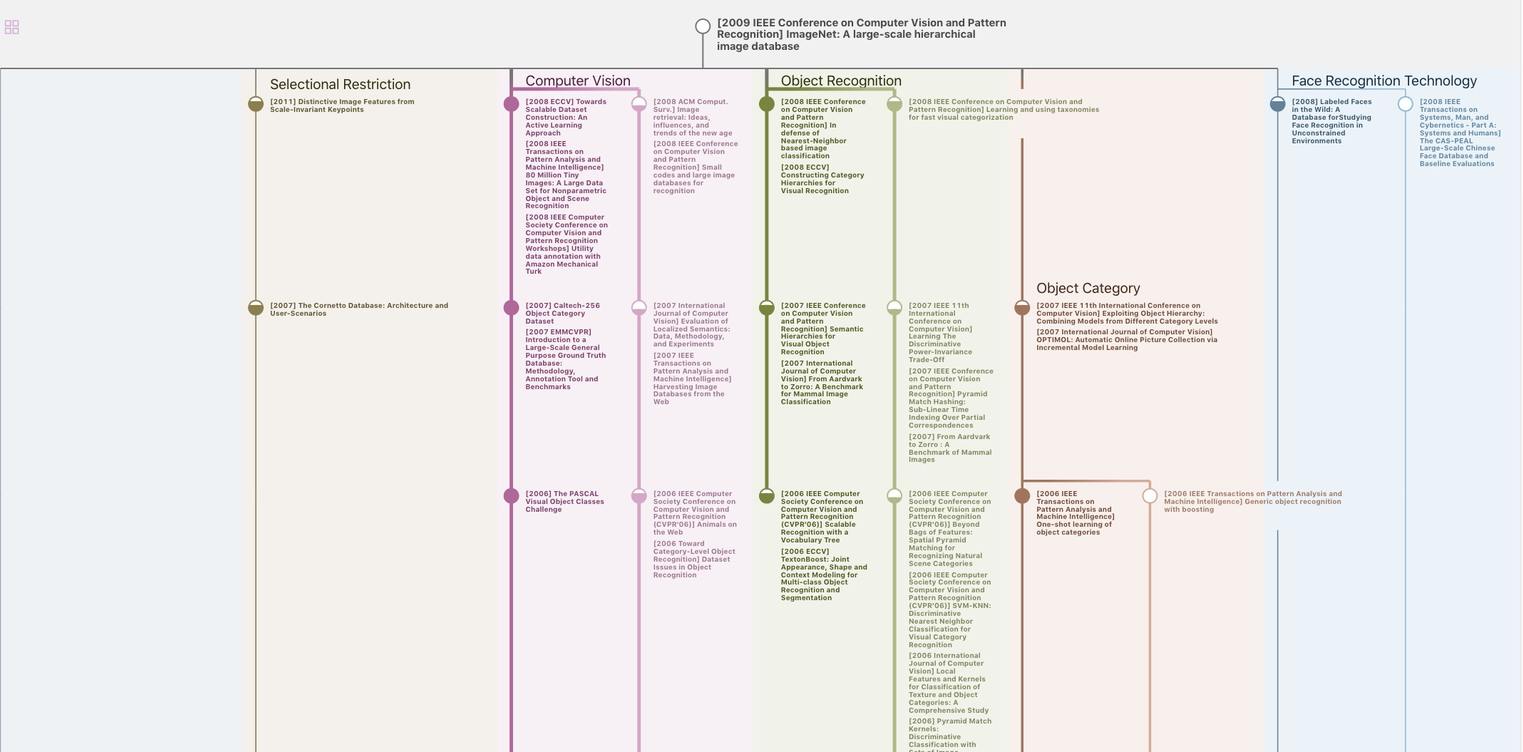
生成溯源树,研究论文发展脉络
Chat Paper
正在生成论文摘要