Gear Pitting Fault Detection: Leveraging Anomaly Detection Methods
2023 14th International Conference on Electrical and Electronics Engineering (ELECO)(2023)
摘要
Monitoring and maintaining the health of gears is crucial for the efficient and safe operation of mechanical systems. Due to harsh operating conditions, gear failures such as wear, pitting, and breakage are common. This study investigates the effectiveness of unsupervised and semi-supervised deep anomaly detection methods for identifying distributed pitting defects in gears using vibration data. In the experimental setup, gear faults of varying severity were created, and vibration data from helical gears were recorded for each level of fault severity. Autoencoders (AE), Variational Autoencoders (VAE), and Deviation Networks (DevNet) have been utilized to detect faulty gears. This study presents the performance of these techniques in predictive maintenance based on the availability of fault data.
更多查看译文
关键词
Anomaly Detection,Pitting,Anomaly Detection Methods,Harsh Conditions,Technical Performance,Variational Autoencoder,Vibration Data,Training Set,Deep Learning,Support Vector Machine,Convolutional Layers,Time Domain,Accelerometer,Long Short-term Memory,Latent Space,Types Of Defects,Vibration Signals,Image Analysis Methods,Encoder Output,Autoencoder Model,Anomaly Score,Variational Autoencoder Model,Number Of Pits,Input Shape,Severe Fault,Exceptional Capability,Horizontal Acceleration
AI 理解论文
溯源树
样例
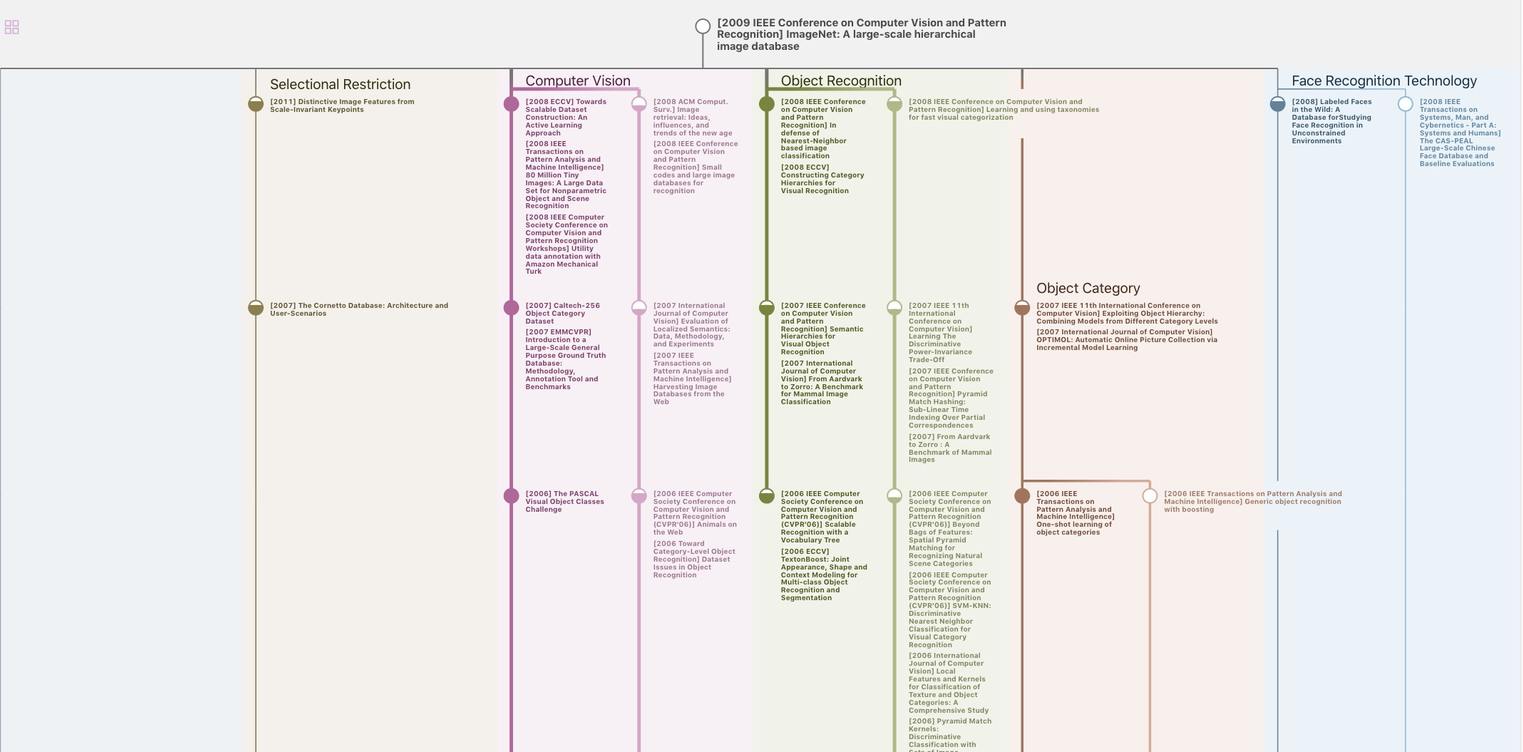
生成溯源树,研究论文发展脉络
Chat Paper
正在生成论文摘要